Xilinx Project Everest 7nm ACAP Family
As part of the discussion, Victor Peng, the new CEO of Xilinx went into a teaser of the first ACAP family. We were also able to ask some important questions regarding how Xilinx plans to drive adoption for this new architecture.
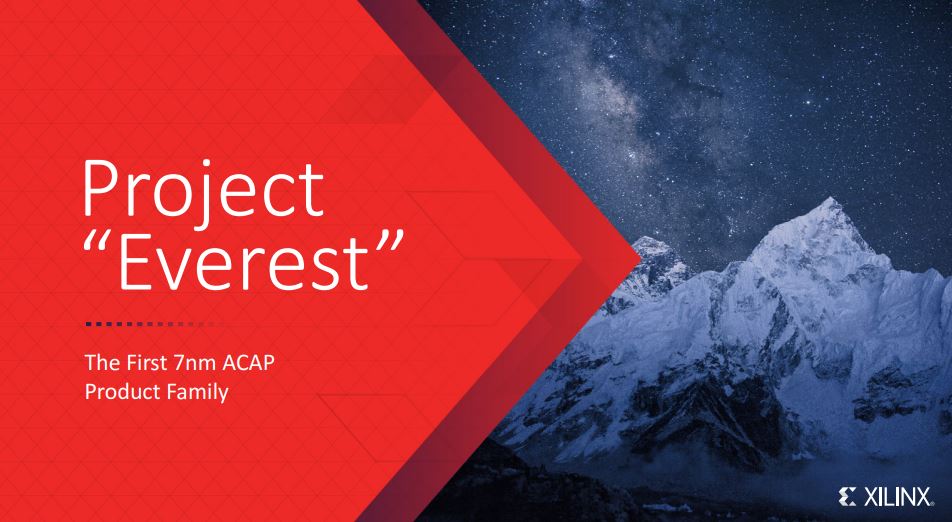
The Xilinx Project Everest is the culmination of a large-scale R&D program. The project spanned four years, 1500 engineers and over $1B in R&D spend. To say this is a big bet for Xilinx is an understatement.

The goal was clearly to address a few emerging trends. Two big ones here are the 5G rollout and the boom in AI compute, especially on the inferencing side.

Wireless networks have been a stronghold of FPGAs for generations. The new Xilinx Project Everest ACAP is designed with a number of acceleration blocks along with up to 112G SerDes. In comparison, we just covered the Intel Stratix 10 TX Launch for Communications Markets that supports up to 58G SerDes, not 112G from what we were told.

The reason this was a bit of a teaser is we did not get a lot of information behind the blocks. We were told that software tools were coming, silicon tape out is 2018 with production shipments in 2019. This is essentially next year’s technology. At the same time, if you are trying to disrupt the market, getting the market excited about your new hardware and software early in the process is a good thing.
In terms of the ACAP, this is a better look at the block diagram above. You can see that there are a number of different logic and I/O blocks all connected via a high-speed interconnect.

We will hopefully get more details, but there was a burning question: how will Xilinx penetrate a market dominated by NVIDIA and to some extent Intel?
Probing how Xilinx will Attack NVIDIA with ACAP
STH: How do we help get the big ecosystems primed for using Xilinx when much of the video processing, deep learning, and AI work is focused on the NVIDIA ecosystem at present? Is it simply offering the libraries to current tools so one can offload to a different type of compute or will there be programs supporting universities and ecosystems specific for the ACAP line?
Xilinx: Much work on deep learning/AI is indeed done with CPUs and GPUs for the training of deep neural network models. But for AI inference – when networks are deployed, these are still very early days for the industry. Most AI inference is still done on inefficient CPUs and ecosystem players are working on the best way to deliver acceleration of efficient and highly responsive (low latency) implementations. What’s more, GPUs are not efficient at a number of workloads such as for video transcoding, genomics, and big data analytics. They are lacking the flexibility to be tuned with customer hardware, custom precision, custom memory hierarchy.
Application libraries are key, of course. They can be used to accelerate popular development frameworks (e.g. TensorFlow, FFMpeg, GATK, Apache Storm…), they abstract the complexity of the hardware for SW developers creating accelerate-able applications. In the last year, cloud based developer resources have dramatically increased the accessibility of FPGAs. Both approaches will be used for gaining broad reach and fast adoption for ACAP development.
Our University programs and community boards and solutions like Pynq, or Cloud based FPGA system like the Xilinx-based AWS ECS F1 instance, are critical to train and foster the next generation of problem solvers. Xilinx is stepping up its presence in open source communities, hackathons, research projects, etc to lead innovation and attract a thriving ecosystem.