Recently we came across a presentation that put some numbers around a server application that all of STH’s readers will be impacted by either personally or with family or friends: digital pathology. We have been hearing about AI-enabled assists for doctors for years. The question then is, what is required to make that happen? It turns out, many PB worth of storage and lots of GPUs.
What it Takes to Handle Digital Pathology PBs of Storage and GPUs
The idea of AI-powered digital pathology is one that has been around for some time. We saw this from a PowerPoint presentation from QCT and aetherAI so that is why you will see them here. Since this is their PowerPoint, and not everyone has the PowerPoint, we can say they are sponsoring this. The idea is that slides are scanned using a pathology scanner searching for things like breast cancer, gastric cancer, lymph node metastasis detection, and so forth. Unfortunately, something that hits close to home so that is why I wanted to write this up for STH. Early detection of cancer tends to lead to higher survival rates so I want to see this technology deployed in more places.
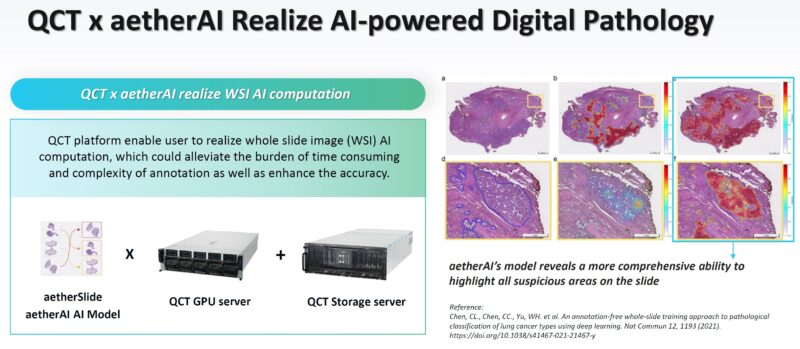
Once the slides are scanned, the scan needs to be stored, and then AI inference is done to detect possible trouble spots. This is a slide that gives some numbers to things that we do not often see. For example, the AI inference takes over 100GB of GPU memory, so a multi-GPU system is usually used.
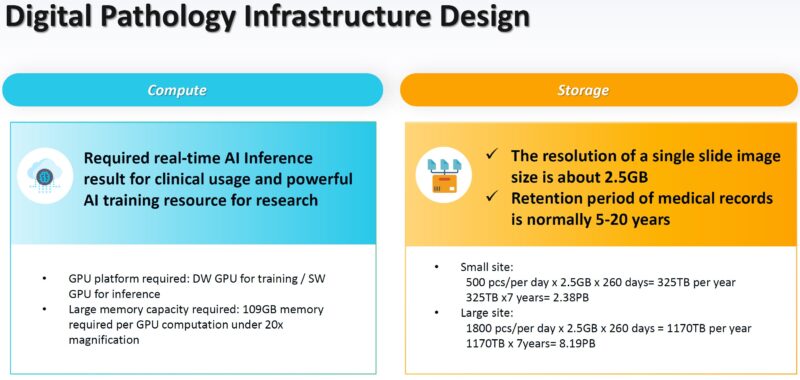
On the storage side, there was more. A single slide scan is around 2.5GB, and it is retained for many years. The case study in this PowerPoint was for ~25 pathologists with 9 high-throughput scanners that would generate around 1800 slides/ day. That is the “large” site below generating around 1.1PB/ year in data that needs to be stored for 7 years. As a result, the data is tiered to lower cost and lower performance storage the older the scan is.
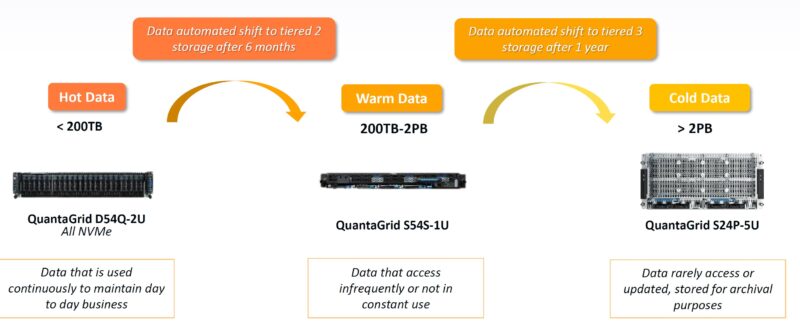
In the slide, there was the QuantaGrid D54Q-2U all NVMe system using 2.5″ drives. We also saw a version of the D54Q-2U with all E1.S drives that you can see here:
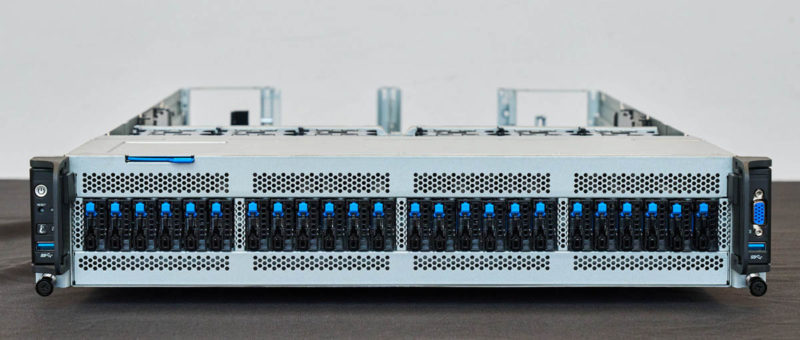
This is a diagram of the architecture for the aetherAI solution for those who want to see the overall layout.
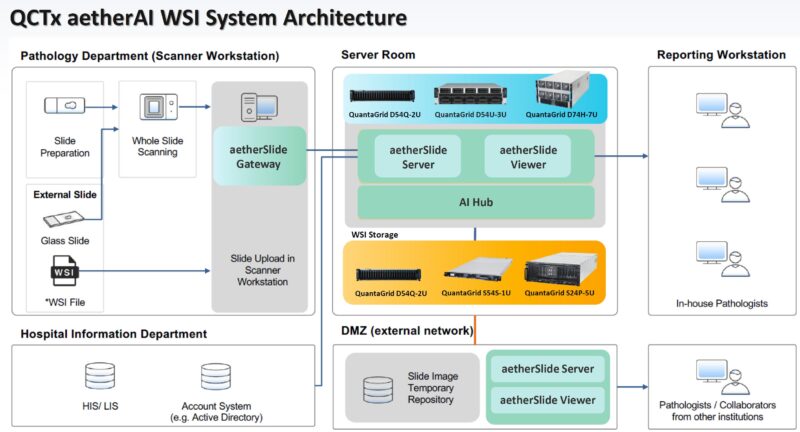
It was interesting to see that not only is the QuantaGrid D74H-7U SXM5 GPU server used for AI inference, but also solutions for PCIe GPUs like the QuantaGrid D54U-3U that we saw at Intel Innovation 2023.
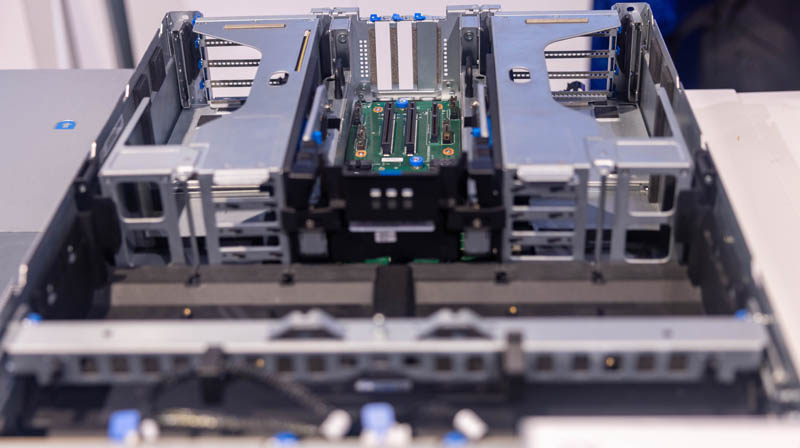
In case you are wondering, the same motherboard is being used with different PCIe layouts for many of the solution’s AI inference and storage servers.
Final Words
I have probably seen a hundred presentations on how AI will change the medical field. This was the first presentation I saw with actual numbers on image sizes, typical throughputs, and the storage and GPU servers needed to actually run a setup doing the work. Since this is a part of the medical field today, and it is something that I imagine many others have either benefitted from or might in the future, I thought it was worth showing off. We also have many architects reading STH who might find something like this interesting as well.
Of course, there is a lot of room to scale up and down this kind of solution, and different instruments create different size data sets. Still, it is an architecture that I did not fully appreciate the data challenges of before seeing this presentation.