This week, we saw El Capitan landing another HPE and AMD system atop the Top500 list. Through a packed meeting schedule, the constant questions involved the impact of AI. There was one discussion that made folks uncomfortable: The potential impact of the new administration on the procurement of large-scale high-performance computing systems. We can point to AI hardware and HPC mixed precision algorithms, but perhaps the incoming Department of Government Efficiency or DOGE is the more interesting angle. I figured it would be worth at least jotting some thoughts and viewpoints I heard this week, and starting the discussion.
US HPC and the DOGE Impact on Next-Gen Supercomputers
In our recent xAI Colossus tour, I mentioned that the 100,000 GPU Phase 1 stood up in 122 days was much faster than public supercomputers. Given the performance of FP64 calculations is different for AMD and Intel GPUs versus NVIDIA, still in approximate system size, a number of nodes/ accelerators gives us some sense of scale.
- Frontier: 1 CPU, 4 GPUs, ~9400 Nodes (2022)/ ~9800 Nodes (2024)
- Aurora: 2 CPUs, 6 GPUs, ~9000 Nodes
- El Capitan: 4 APUs, ~11,000 nodes
Those three systems combined should use just over 100MW with ~140K accelerators. The xAI team has already stated a goal to grow this number quickly to 200,000. At least from an order of magnitude perspective, I would not be surprised if xAI Colossus surpassed the number of CPUs accelerators Frontier, Aurora, and El Capitan had combined within a year. At 200,000 GPUs it should easily be a bigger cluster than those three combined.
Networking is really interesting. Many of the HPC folks will point to AI clusters and say they are less good. On the other hand, we showed xAI Colossus’s Supermicro liquid-cooled systems, and you will see HGX H200 reviews this quarter on STH, all with one 400GbE NVIDIA BlueField-3 SuperNIC for each accelerator and one 400GbE BlueField-3 DPU for each of the NVIDIA GPUs. Even in its Phase 1, 100,000 GPU form this was over 110,000 NICs. The switches are also much newer and bigger than Rosetta 1 based HPE Slingshot 10/ 11 (100G/200G) switches deployed in the three current Top500 Exascale systems.
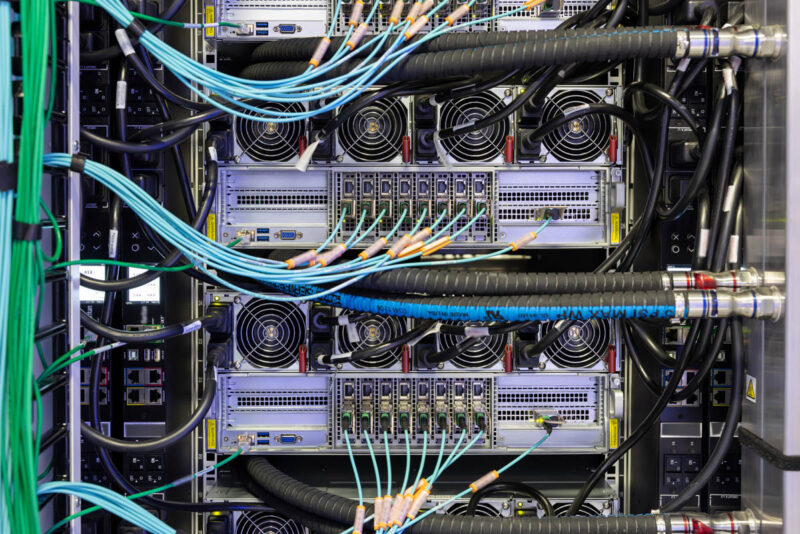
If we use a 2019–2020 start date the systems took perhaps 3-5 years to deliver after the procurement contracts were announced, and there is generally a procurement process that happens before eventually the system is announced, the US DoE effectively built three very different systems in 5-6 years that Elon Musk’s xAI team will do in well under a year, and got reasonably close to in 122 days.
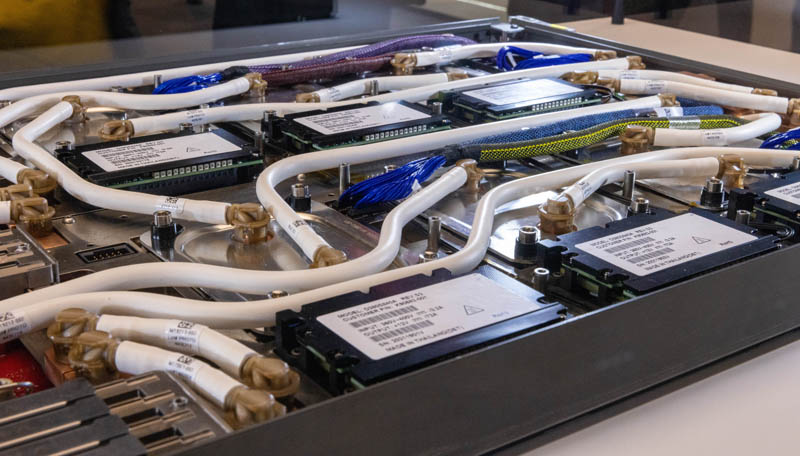
Of course, the US DoE is scrappy. It is not directly competing with companies like Microsoft, Meta, and more buying NVIDIA GPUs at high prices. Most likely the three public US Exascale supercomputers were a publicly floated budget when announced of under $2B is probably a third of what xAI Colossus costs just based on NVIDIA’s supply constrained pricing.
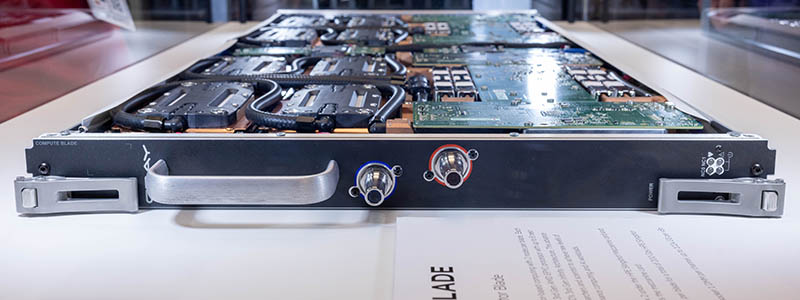
Summing this up in some rough napkin math, the US DoE managed to in 5-6 years buy and deploy three systems with roughly what Elon Musk’s team built or will have built in under a year, and at a cost perhaps closer to a third of an AI system.
Here is my law that I have been floating with hyper-scalers and many in the industry:
The value of an AI accelerator is greatest when it is first deployed, and decreases thereafter.
Some say it is when the accelerator comes off the line at the fab, but until it is packaged, then installed in a system, and then deployed into a cluster it is effectively a paperweight. If you look at GPU pricing over time, it consistently decreases as the supply of each successive (NVIDIA) generation of accelerator is deployed at greater scale. An available NVIDIA H100 in Q4 2024 is much less than it was a year ago and much more than it will be in a year.
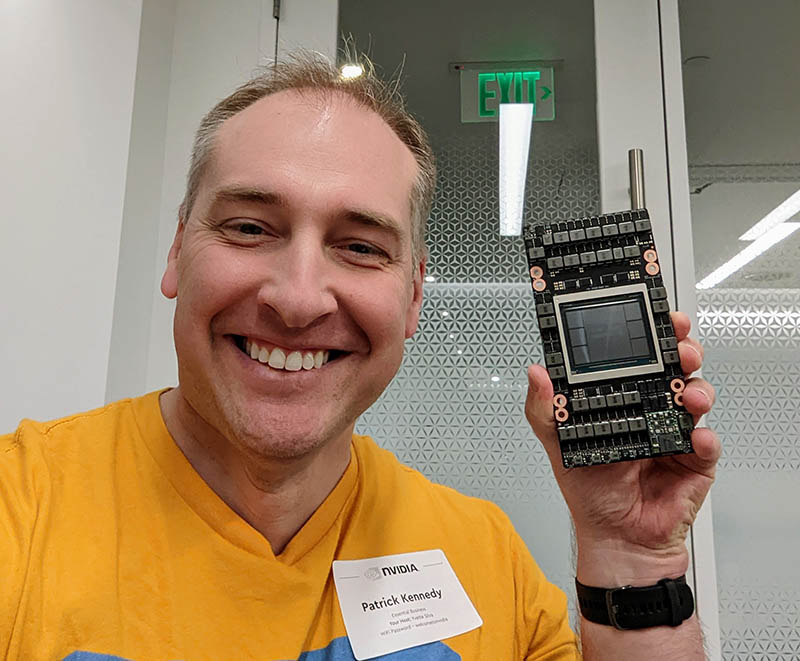
Bringing this back to the Department of Government Efficiency or “DOGE” with an aim to increase the efficiency of government expenditures, we can imagine the meeting between DOGE and the agencies involved in next-gen system procurement. Perhaps 1/6th the speed, and 1/3rd the nominal cost ignoring the time value of money building systems that are 1/3rd the size.
In theory, the US Government should be able to deploy faster than a private company. Everyone that is involved in IT hardware deployment knows that the US Federal Government can check a box on an order and legally, it jumps to the head of the line in a NVIDIA supply constrained environment. It also has broad powers and existing facilities that it could use to streamline site preparation.
Given that, it feels like before a DOGE meeting, agencies will want to re-think their speed to deliver systems. How do you tell someone who built a bigger system in a year with a private organization that it takes 5-6 times longer to build smaller systems with the weight of the US Government?
Non-Recurring Engineering Components
Of course, there is a lot more to the procurement of these Exascale systems that go beyond the hardware. The programs often include extras because they are deploying novel architectures.
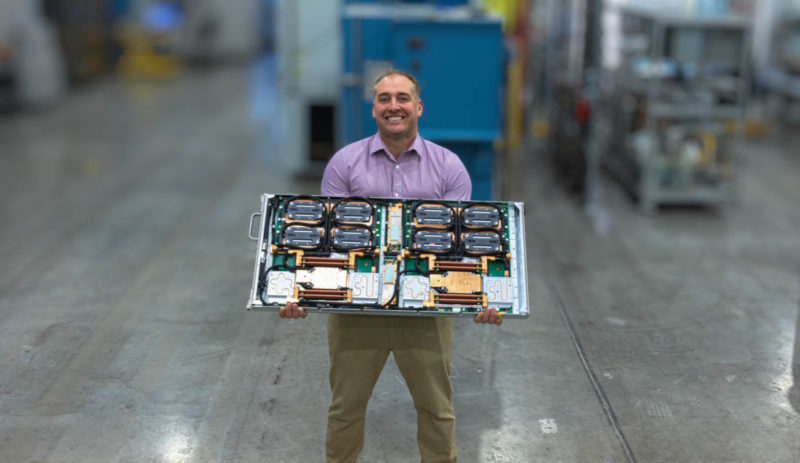
For example, under the current contracts, work has gone into porting HPC codes to GPUs, making codes work on the Intel and AMD GPU architectures as well as the APU architecture of the AMD MI300A. There is a lot of great work happening and being highlighted in forums like Supercomputing 2024. That work certainly has value.
One of the more notable impacts of this work is that it has probably helped AMD become a #2 to NVIDIA. Microsoft is deploying many AMD GPUs in its infrastructure. Without AMD and perhaps Broadcom/ Marvell working with hyper-scalers on AI chips, NVIDIA would have even more pricing pressure in the AI space. We will just say that Intel desperately needs Falcon Shores at this point. The work that went into Frontier and El Capitan has probably saved the industry billions of dollars by helping a lower-cost second source for some AI installations.
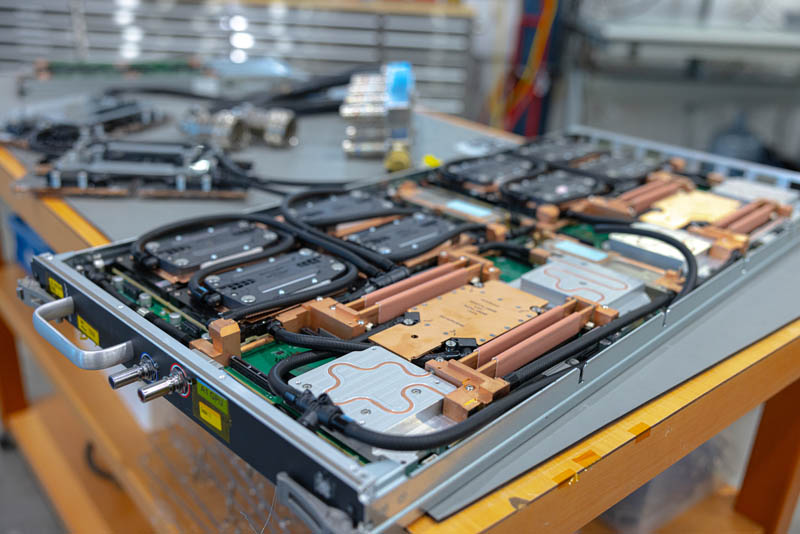
It is strange, but if AMD can quantify the impact of the Frontier/ El Capitan NRE efforts and its wins have had on NVIDIA’s pricing dominance it probably would help both AMD and NVIDIA.
If the purpose of building a supercomputer is to do as much work in a science domain as possible at the best value, then the next question will be what is the fastest way to achieve that value. A big difference between those making AI clusters today versus those making government supercomputers is the voracity in quickly achieving that value.
Final Words
Some have speculated that if xAI Colossus submitted a HPL result for a Top500 it would have easily been #1. Others think that the interconnect might have held it back. Our guess is that before being notified of their #1 Top500 submission last week, the LLNL folks with El Capitan were preparing SC24 materials for being #1 or #2. We know that there are several hyper-scalers that could have submitted Top500 results. Our best guess on why they did not comes down the law on AI accelerators above. Running HPL on an AI system when it is deployed takes the more valuable compute time than the system will have at any future point making it an inefficient use of mutli-billion dollar systems.
After filming the xAI Colossus video, and seeing it being built on a prior trip, it is immediately obvious that there is an enormous gap in the speed private companies are building large-scale systems versus the government. Since the new administration has announced plans to review large-scale government spending with DOGE, and one of the DOGE leads can legitimately say his teams build much bigger and faster, those involved in the solicitation and planning for next-generation US supercomputers need to prepare answers.
To be clear, I think the US building supercomputers is great, and I do not think this is a complete waste of taxpayer dollars by any means. It is by far not the most wasteful spending category since there are tangible science benefits and we are really discussing scale, cost, and speed deltas. Perhaps the big impact if DOGE takes up supercomputing will be the voracity of how these systems are deployed.
DOGE/Musk is more of the same – grift. I am very doubtful anything positive for the nation comes from this. How does an efficiency team have 2 leaders? Its a farce. They’ll cut regulations that make it easier for their companies to make money and put the rest of us at risk.
The whole cabinet is scoundrels – Trump, Gaetz, Fox Talking Head, Tulsi, Brain Worms Jr, WWE Lady. Seems pretty swampy to me.
Lower your expectations of what they can actually get done.
LLNL should be thanking Elon’s team for not submitting Linpack numbers. 1024 H100’s is around 45PF. If you’ve got 100 times as many GPUs, that’s 4.5EF. Running at half the efficiency scaling would still make it #1 by a great margin
There’s grift in all government contracts. I don’t think this is the first area they’re going after and we’ll probably see the next contract announced before they get to it. But you’ve made some good points.
When you have to do things like actually honor agreements with unions, not overwork your employees, not cut corners or burn cash to get ahead, planning on what you build functioning for a longer term, all these things tend to take longer.
Reworded: Doing things the right way is rarely ever the fastest way.
Government installations tend to be online for long periods of time and are expected to function across that lifespan. I seriously doubt the xAI cluster is expected to survive longer than 5 years at most before being completely replaced.
Why are you talking about Doge like its a real thing? It has no government mandate, there is no vision from its fake leaders, congress hasn’t given it any power. Its just a Billionaire vanity campaign, it will not do anything other than allow MuskRat to pretend he’s doing another fake job he doesn’t actually do.
Offtopic, can’t resist sorry
Patrick, with all due respect on the photo of yours holding Cray Node you look like a LEGO figure
If DOGE cannot achieve anything then we, as a country, are doomed. That’s not hyperbole, that’s where we are right now. $36 trillion in debt and increasing fast. Inability to secure social security for those paying in currently. I really feel that those who are speaking so harshly about it are blinded by their political tribe. We are on the precipice. All of us. Maybe it will amount to nothing but we’d all better hope it amounts to something. Because we know the Senate will do nothing to help the situation anytime soon.
The very first DOGE agency review meeting will come to a screeching halt when Elon sees two words on the slide deck that he doesn’t recognize:
“budget” and “deadline”
In all seriousness, the best way to ensure that US government agencies get their jobs done on-time and under budget is to keep Elon and his vanity AI project far away from them. That dude is the human manifestation of scope creep.
It’s amazing that there’s this many anti-Elon and Vivek people out there. Dude’s making rockets faster and cheaper than Boeing.
I don’t think this should be the first government slimming they target, but you’re right that if DOGE does take aim, they’ll need answers.
Why are you taking DOGE or Elon seriously? He’s not going to have any positive impact in anything he does from now on. DOGE is not a real thing and if you want to look at what he’s capable of now look at the absolute dumpster fire he turned Twitter into, as an organization, the tech, the business, and the actual product.
Talked himself into paying $44B and then turned it into less than $10B in under a year with no signs of ever recovering from that.
“DoGE” is an imaginary institution, laughably set up with two heads, and for the main purpose of granting token status/favor to two loyalists.
Musk has made career out of betting on smarter people and “picking good racehorses” (in terms of next-gen technology). If anything, SpaceX and Tesla have surprisingly managed to thrive and survive despite his poor stewardship in recent years. Musk has never been known for being efficient, never good at collaborating with others, never has displayed any desire to be a public servant. He is a *terrible* pick for any position within government.
If Musk has any influence on the next generation of supercomputers or datacenters, it is likely to be in the form of grift or power for his own benefit.
@Matt
On the precipice? No sir, we’ve already gone over that. The moment Trump became our President, the train blew right through the end stop and sped over the edge harder than a proverbial lemming. We had 1,000,000 feet before slamming at hypersonic speed into the rocks below. I predict we now have 800,000 feet to go and the feet are only counting down and ever faster. We are accelerating to certain destruction. Nothing is going to stop that. It’s going to hurt bad, assuming we will even survive the impact. I’m doubting we will.
These comments are absolutely deranged! Taking one less visit to CNN or Fox each day would do you folks a world of good.
@A Concerned Listener
Thanks much for your concern. I stay FAR AWAY from the likes (dislikes?) of Fox and CNN. I don’t have cable. Hell, I don’t even have a TV. MY comment isn’t based on what I don’t see on Fox or CNN. I truly do feel we have gone over the edge and we positively WILL hit bottom. This is my opinion based on what I see in the world today. I don’t need the “news” to tell me how wrong things are. It’s a sense I’m getting given the terrible political climate we are living in.
Again, your concern is deeply appreciated.
Lots of single liberal simps on here crying about DOGE. Go outside, fellas.