At Hot Chips 35 (2023) SK hynix is applying its expertise in memory to the big computing problem of today, AI. At the show, it is showing its work on Memory Centric Computing with Domain Specific Memory. The company is looking at ways to alleviate one of the biggest challenges with AI compute today, memory capacity and bandwidth in relation to the compute resources available. Let us get to the talk.
Please note, we are doing this live at the conference. Please excuse the typos. It is a crazy rush of a conference for coverage with back-to-back presentations all day.
SK hynix AI Memory at Hot Chips 2023
Here is SK hynix’s problem definition. Generative AI inference costs are enormous. It is not just the AI compute. It is also the power, interconnects, and memory that also drives a lot of the costs.
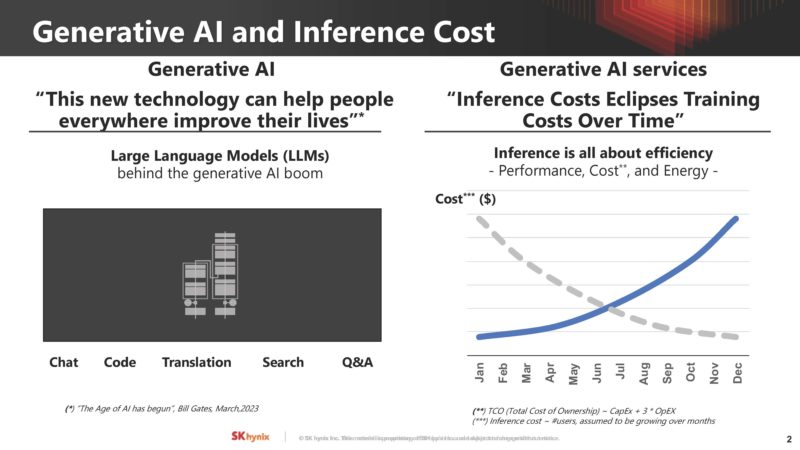
With large transformer models, memory is a major challenge. These models need a ton of data, and therefore are often memory capacity and bandwidth bound.
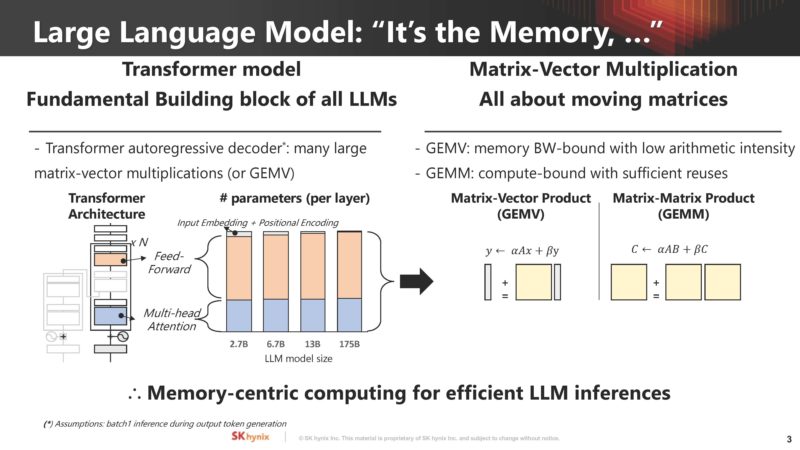
SK hynix is setting up that the industry needs more than just memory, it needs different types of memory including domain-specific memory with compute built-in. Both Samsung and SK hynix have been working towards becoming in memory compute providers because that is how they move up the value chain.
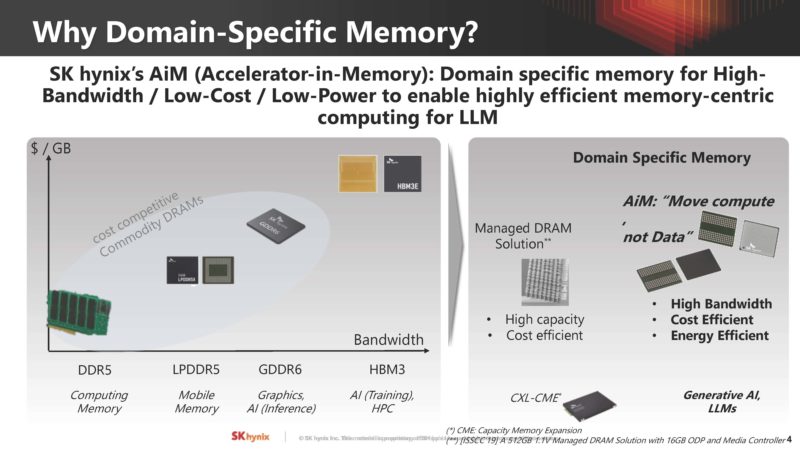
On that note, we are going to hear about Accelerator-in-Memory or SK hynix AiM.
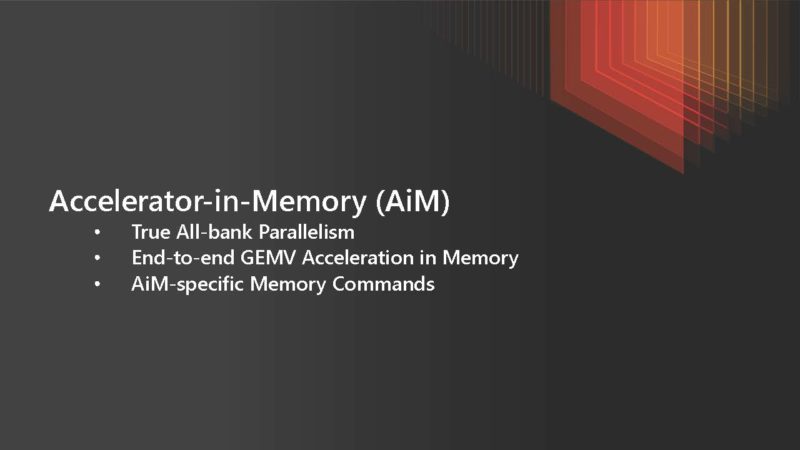
Here is a look at the GDDR6 memory where there are banks of memory each with its own 1GHz processing unit capable of 512GB/s of internal bandwidth.
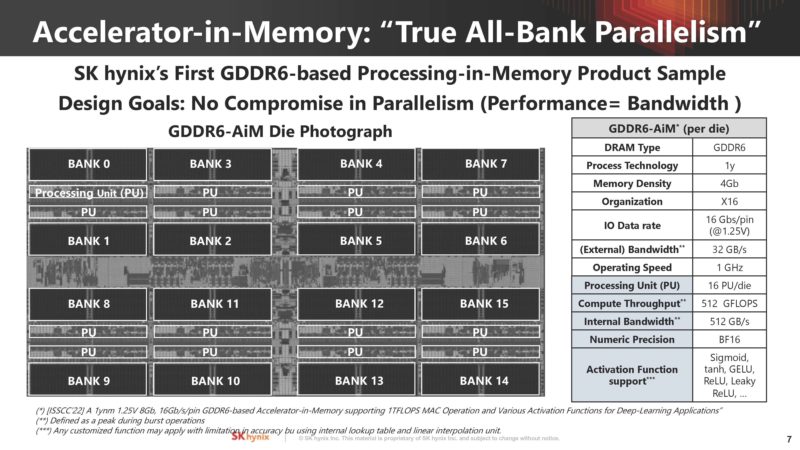
SK hynix discussed how it plans to do GEMV in memory for AI compute. Weight matrix data is sourced from banks while vector data comes from the global buffer.
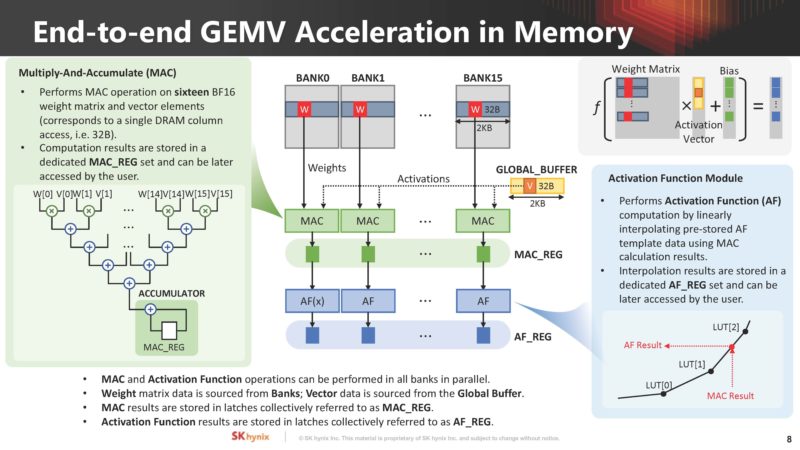
There are specific AiM memory commands for the in-memory compute.
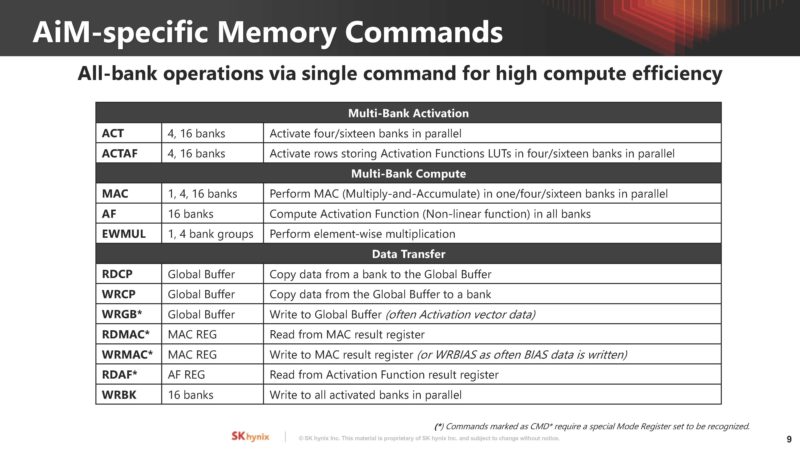
SK hynix is showing how memory scales and the need for AiM in-memory compute resources are required for large language models.
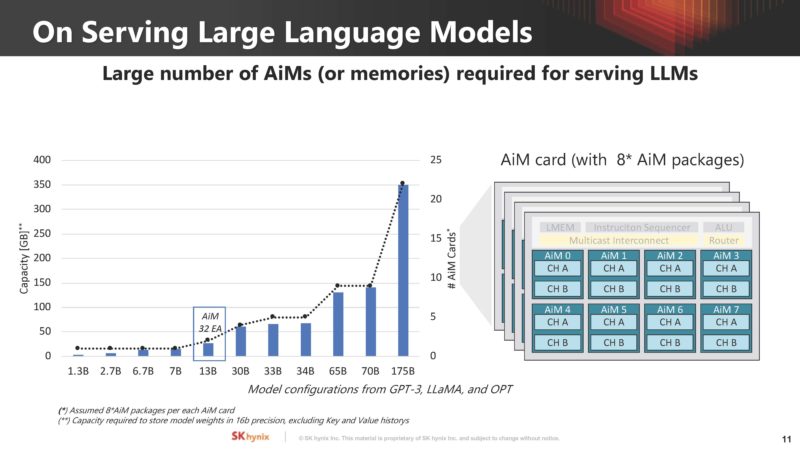
Here is how these scale out for large language models (LLMs):
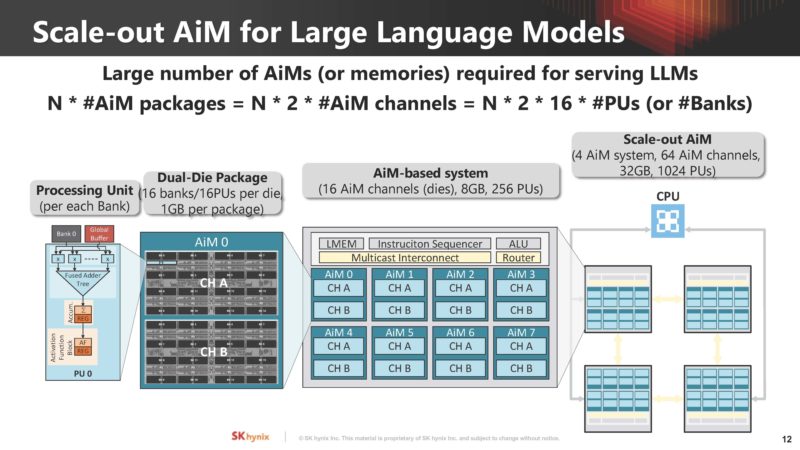
One of the big challenges is that using this type of AiM is that it requires mapping from the software side, hardware architected for AiM, and then an interface. This is one of the other big barriers for adoption.
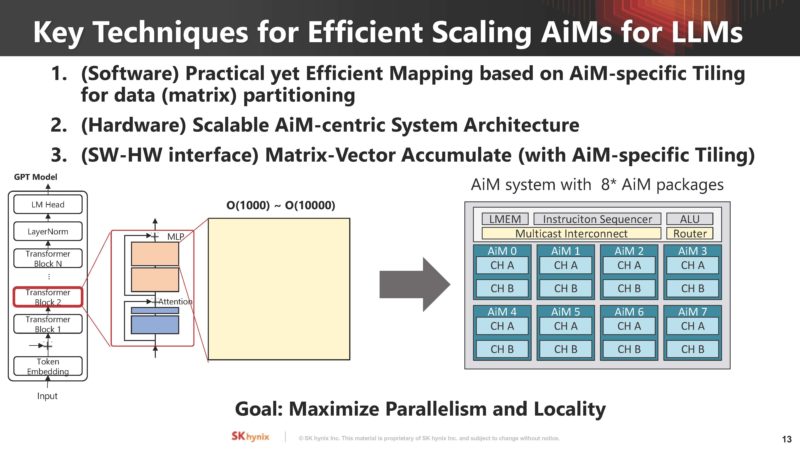
Here is how SK hynix is looking at doing the mapping from problems to AiM.
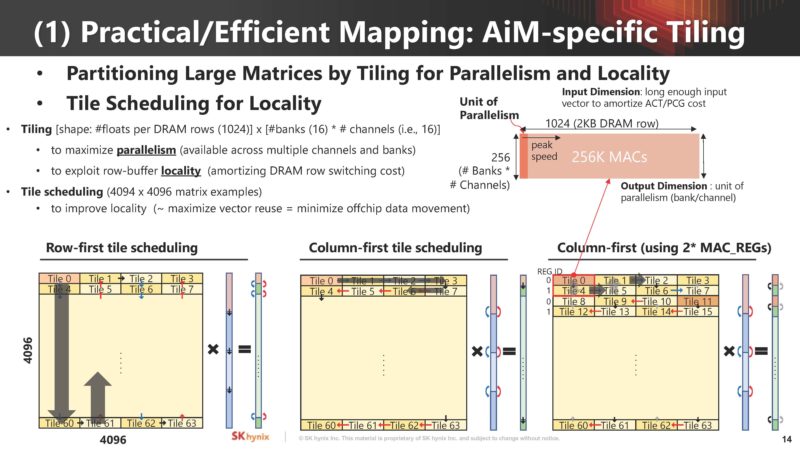
The system architecture needs to handle scale-up and scale-out.
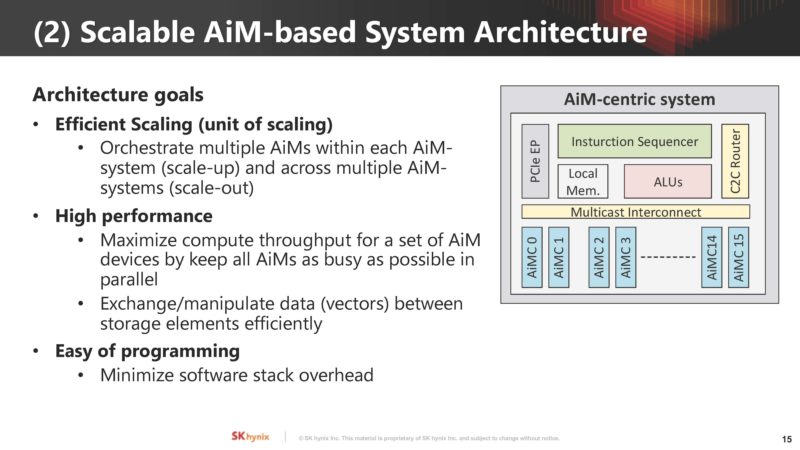
Key components of the AIM architecture are the AiM controller, Scalable Multicasting Interconnect, Router, Compute Unit (ALU), and an Instruction Sequencer.
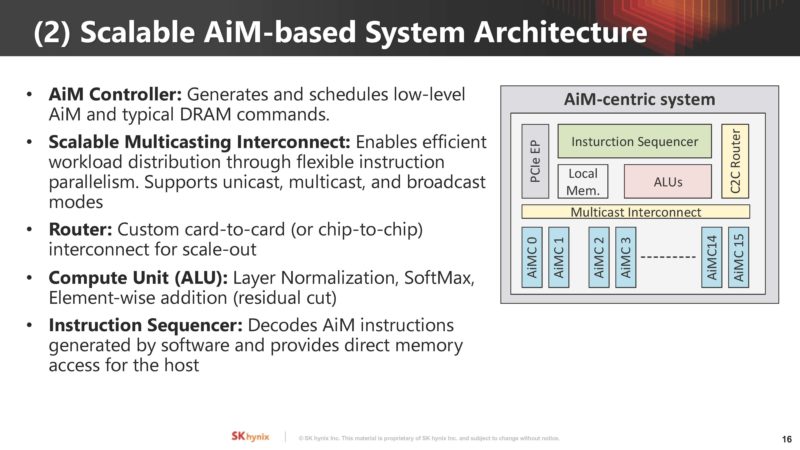
Matrix vector accumulate functions are key to AI workloads. SK hynix AiM uses a CISC-like instruction set to manage this.
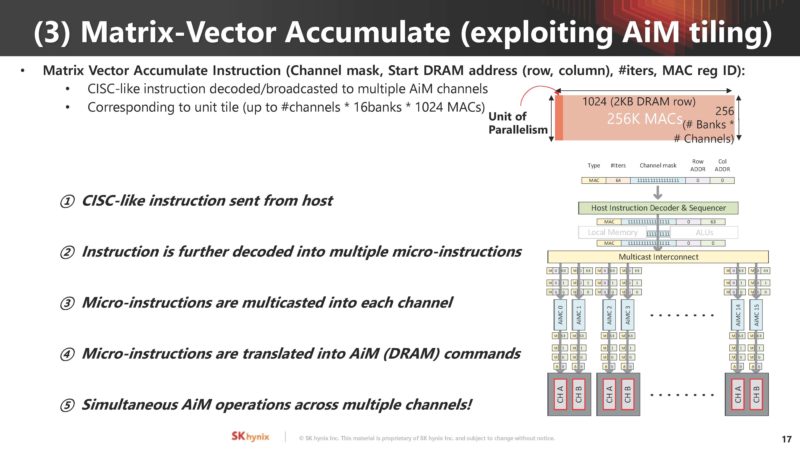
The next step of this is optimization. With a new architecture, often there are nuances that can be exploited to get better performance.
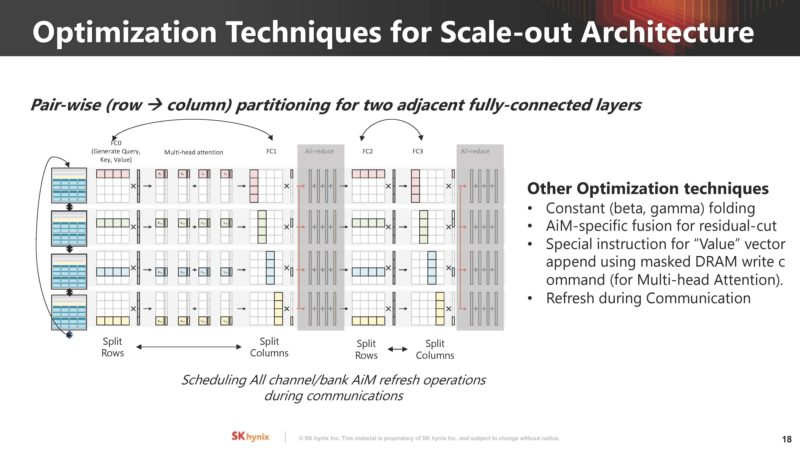
SK hynix did not just talk about AiM in the abstract. Instead, it showed a proof of concept GDDR6 AiM solution using two FPGAs.
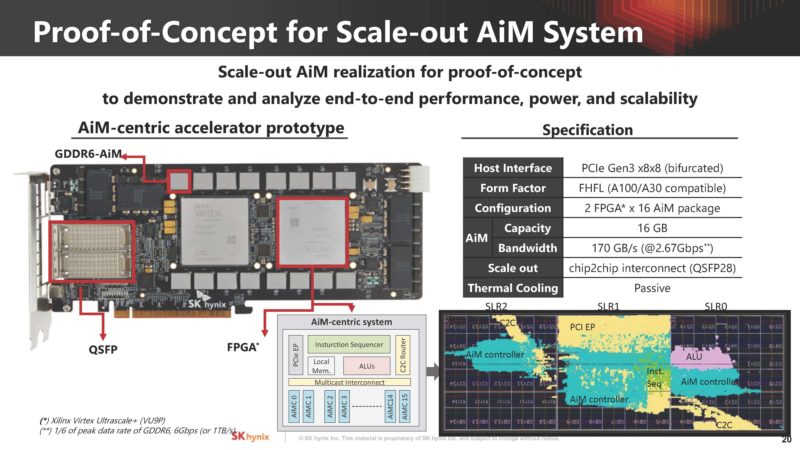
It also showed its software stack for AiM.
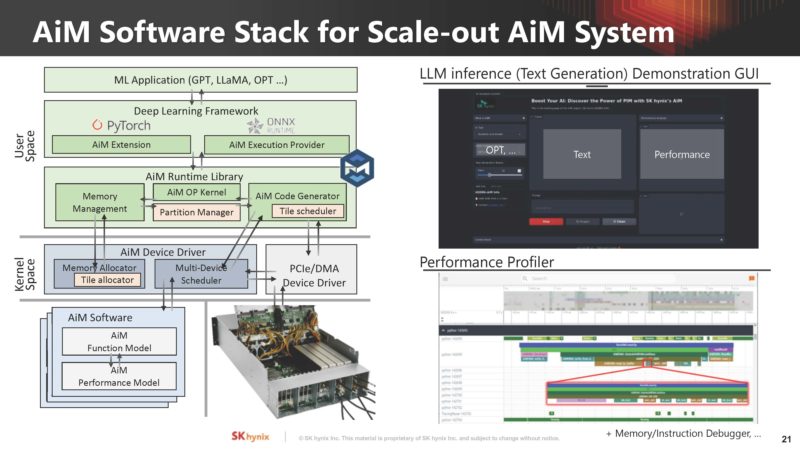
It does not sound like SK hynix is looking to sell these cards, instead, these seem like they are being used to prove out the concept.
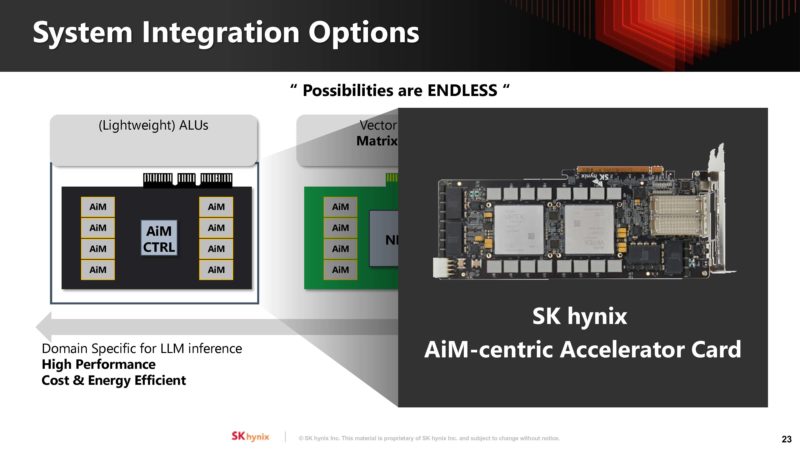
SK hynix is still in the evaluation stage doing different types of analysis on the solution versus more traditional solutions.
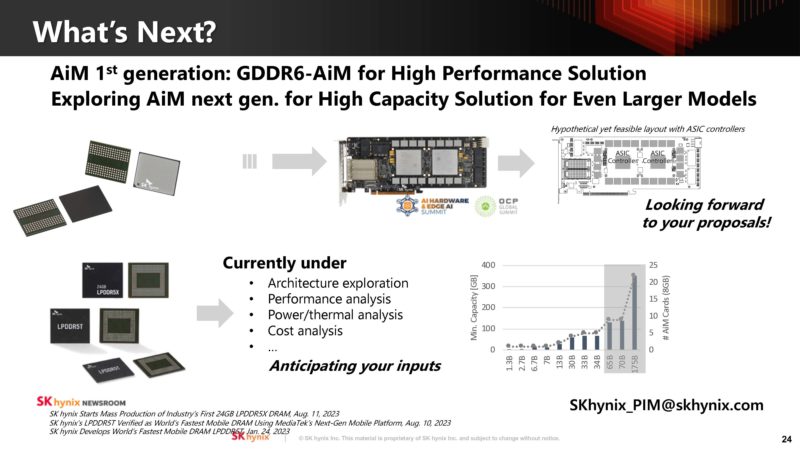
So this is being looked at for the future.
Final Words
Both SK hynix and Samsung have been talking about in-memory compute for years. It will be interesting to see if a big customer adopts this in the future. For now, it seems like the next generations of AI compute will be more traditional in nature, but perhaps this is one of the areas that will take off in a few years.