Here is the complete interview, the outline with questions is on the preceding page.
Overview
Would you mind giving our readers a bit of background about yourself and your journey to Cerebras?
Andrew Feldman (AF): Sure. This is my fifth startup. For the last 13 years have been building companies with Gary Lauterbach, who’s our CTO, and one of our founders here.
Before this, I founded a company with Gary, around some of Gary’s ideas, called SeaMicro. We built that over a four year period and we sold that to AMD in 2012. We stayed with AMD for a little while and then took some time off and when we were ready to create again we got some of the band back together again. All the founders were with us at SeaMicro.
SeaMicro was Gary’s idea. The germ of this idea, I think, was also Gary’s, and we added some of the extraordinary engineering leadership that we had at SeaMicro. Sean Lie, Michael James, and Jean-Philippe Fricker each brought a different part of a vision for an extraordinarily complicated system like ours.
It’s good to have founders that have worked together for a long time. You know, we’ve been together now for many of us for 10 years. JP, this is the third company of work with JP at. Then we were able to, as we grew and needed some different skills, we were able to go back to the well to guys we’ve work with before John Turk, who’s our VP of Ops and Customer Support joined us. He’s been at SeaMicro in the same role. Dhiraj Mallick who runs hardware and ML for us had been with us at SeaMicro also.
I think one of the good parts about the valley is good engineers want to work with each other, and they remember. So some of the team I’d worked with from 1996 to 2003, and others from 2003 to 2007. And some were with us at SeaMicro. Of about the 200 people here now, maybe 65 or 70 years of work with me at some time or another in their career.
How did you and the team start down the path of “what if we built a wafer-scale chip?” This is a revolutionary approach so how did that ideation through getting to the point where you thought it was feasible occur?
AF: What if we solved an open problem in the industry that for 70 years nobody to be able to solve that? had destroyed? thought about this right?
It began first with sort of a fundamental question Gary asked, and that’s, he asked one day as we were sort of ideated as we’re meeting to think about what we should do. He said, why would a processor, that had been built and tuned for 25 years to push pixels to a monitor, why should it be good at deep learning?” Right, and wouldn’t it be serendipitous if 25 years of tuning a chip for one workload made it really good at a completely unrelated workload.
That sort of cracked the problem in a way that made tractable it showed us a path for that said, What if we studied what the artificial intelligence workload asks of the underlying compute machine? What does the GPU deliver to it, and see what the fit is and see if we do better? One of the things we observed right away was that the GPU has a problem with memory. Its memory is far away. This workload has some very unusual characteristics with regard to memory. Sure enough, when we discovered this architecturally, we also found on the customer side, the immediate ramifications of this problem and that it takes a very long time to train models. So we saw that the memory architecture wasn’t a good fit.
We continued our exploration and what we came to believe right away also was that one chip one piece of silicon few thousand cores, weren’t going to be enough to solve this problem. So as we sifted through the strengths and weaknesses of the GPU, what it’s good at what it’s not good at. We developed a set of ideas around what a machine should be if it were built for this work. Not if it was repurposed. Not if it was brought from some other market for this, but if it was absolutely tuned for this. We came up with four or five tenets, and one of them was, it should be purpose-built. Every square millimeter of your chip needs to be tuned for this work.
We came up with the observation that the hard part of this work was moving data, moving data from memory to the core moving data from core to core. That is because there’s a feedback loop and in a feedback loop data moves.
That led us to sort of the nut in your question. If the problems are going to be big, and you’re going to build a feedback loop, and your chips can only do so much work, how do you get the benefit of a huge amount of memory, and not suffer this historical penalty that you suffer when you try and tie chips together? Because when you communicate on a chip, you’re 1000 times faster than when you communicate from one chip to another chip. And you use roughly 1000 times more power to do it.
So we said well, with this workload needs is a huge amount of calculation, hundreds of thousands, of cores, and it needs those cores to be able to communicate unbelievably quickly and needs a ton of memory. That said, build a really big chip. So once we had the technical foundation, why it made sense, and the returns to success and building a big chip were much bigger than in some other work, that emboldened us.
We studied the previous failures. We met people who’d been at Trilogy when Amdahl failed. We talked to people in in the military and intelligence community who’d worked on large chips, we talked to people who were building satellite chips, which were sometimes bigger than traditional chips. We felt that we had some solutions for the problems that had hampered previous efforts before and that crippled them.
We are engineers who are not afraid. We like doing work that other people shy away from because it’s either too hard or they’re afraid or they can’t raise the capital to do it. It excited us the way solving an open problem in an industry excites you that other people couldn’t do it. We found extraordinary investors. We found other like-minded entrepreneurs who wanted to join us and be part of a company that wasn’t doing something a little bit better. It wasn’t doing something 1.3 times faster but set out to do something profound and transformative. Then we set a goal that we wanted to be in the Computer History Museum. We wanted that when they told the history of the first 50 years of the 21st century of compute that there was a chapter on us. These guys did things that other people tried and failed, they made it work.
What was it like pitching this to investors? What happened when you said, “we’re going to build a chip bigger than any of these other guys can go to.
AF: That wasn’t exactly my pitch.
I think it would have been very, very hard if this had been your first time. We had a world-leading team. We had a team that had built. Gary had been the chief architect of the UltraSparc III and the UltraSparc IV, and a big supercomputer for DARPA. His inventions are now on every chip, every processor used. We were coming off a very successful previous startup. We didn’t look like we had just fallen off the back of a turnip truck. We look like a team that had decades of experience and could attack really hard problems.
We visited top firms and every one of them put term sheets down. You know, ours is not an industry where founders are in their 20s. In the making of chips and systems, these are 60, 80, 100 million dollars before you ship one unit. That’s different than software where you get feedback really quickly. When you put your software out there, you can get feedback. We have huge sunk costs. We were able to put forward a credible team. We were able to talk to some of the pioneers in the industry. Guys like Andy Bechtolsheim, and Pradeep Sindhu, Lip-Bu Tan, Mark Leslie, Jeff Rothschild, Nick McKeown, and the guys who founded some of the most important companies in the last 50 years. They all jumped on, they saw that holy cow, this is hard. These guys are great. This is bold. Imagine what it can do.
So we were able to bring together a consortium that was behind us. They’ve been exceptional with advice along with our venture capitalists at Benchmark, Foundation, Eclipse, Coatue, and the others. Those are the guys who were with us from the beginning and early. It hasn’t been easy. There were months when we couldn’t solve the problem. Not just that we were making progress on getting traction, but we had no idea how to solve a problem. That’s a tough board meeting. Right? When over two month periods, you’ve made zero progress. I think they had faith in us and we’ve justified it a little bit.
How have your investors and advisors helped beyond just providing capital?
AF: I think there’s a lot of capital out there. The valley is awash in capital. Wise counsel and advice are much rarer. I think the venture capitalists at Benchmark and Foundation and Pierre Lamond at Eclipse has been building chips for 70 years now. It’s unbelievable. We’re able to dig down into the technology and bear with us and then the next group of investors who were, sort of financial investors. They had patience and they realize that we were working as hard as humans could work and that more jumping up and down or histrionics wasn’t gonna help us.
One of them sent cookies one day to the whole company. They said, we don’t know how to help but here it is, can’t hurt. They were supportive of me at the board level. They were supportive of our constantly seeking to not do a hack but to solve this problem at the fundamental level. The individuals you know, Mark Leslie has been an advisor and a counselor for me for many years. One of the great CEOs the valleys had. Pradeep Sindhu who founded Juniper. Andy Bechtolsheim. These are guys who have so much to teach. If you meet with them once a quarter, you set your ego at the door, and you listen and you’re not afraid to say, look, I’m not sure if I’m on the right path here, what do you think? That’s how you get good advice.
[Editor’s note: Andy Bechtolsheim is used as a complete sentence in the above paragraph. After reading the transcript and listening to the recording, everyone seated at the table took that as a complete sentence. This is even without the rest of what would normally be required for a full English sentence.]
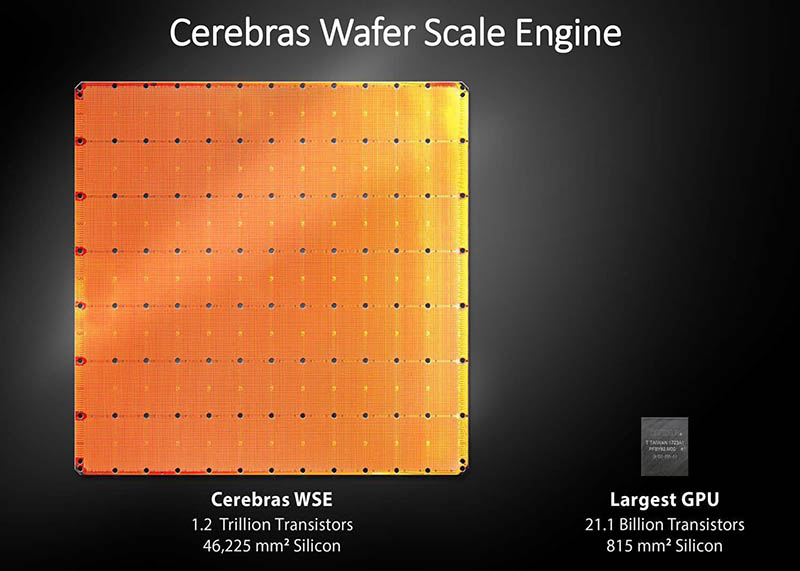
Cerebras Wafer Scale Engine
Can you give us a brief overview of the 1.2 Trillion transistor WSE with the assumption that they can read more detail online?
AF: Sure. Traditionally, we made chips by taking a wafer, which is a circular disk of silicon that’s 12 inches across in diameter, and cutting it up into little tiny pieces. And there was an upper bound on how big those pieces could be at about 815 – 830 square millimeters. Sort of like we took Humpty Dumpty and we broke it apart and we sold eggshells or fractions of eggshells.
In our work though, what we wanted to do is put Humpty Dumpty back together. So we did it with glue and with all this glue to try and get a big egg. We said, “That’s silly. Why don’t break it up in the first place?” So we built the biggest chip ever made. It’s called the Wafer Scale Engine and it’s the whole wafer as one chip. In comparison to the previous best which was 830 square millimeters. Ours is 46,225 square millimeters. The previous largest number of cores was on the order of 5,000. We’re 400,000. We have 3,000 times more memory on-chip in the competition. We have 10,000 times more memory bandwidth and 33,000 times more fabric bandwidth. We didn’t try and do something a little bit faster. We tried to do something fundamentally different. That involves solving problems that had broken others, and some that others never even got a chance to look at.
I tell people, it’s a little bit like the first guys who tried to climb Everest, they got halfway up and said, “Well, this is really hard.” They came back down and they’re in a bar, and they’re talking to some new guys who want to climb Everest. They said, “it gets really hard at this spot halfway up.” The new guys say, “alright, we’ll worry about that.” These new guys, they get all the way up to the top and come back down and they’re back in the same bar. They meet the guys who only got up halfway and they say “the first half wasn’t the hard part. It was really hard near the top.”
All the problems that had broken previous efforts, we solved quickly. But, there are always new ones. How do you deliver power to it once you’ve yielded it, once it works? How do you cool it? How do you write software for it? Those have been enormously challenging and that is why we are a system builder. We don’t just sell the chip, we make the whole solution. It’s a 15 rack unit, 26-inch tall computer, and we make it all. Its engine is this giant chip, called the Wafer Scale Engine.
What it was like the first time you had one that worked?
AF: It took my breath away.
I think that’s the truth. I mean, the founders stood there and we were awed. We were 3+ years into every ounce of our abilities. We were watching something that nobody had ever seen before our industry. For 70 years, people were trying to build bigger chips and everybody had failed. And there it was in bad real estate. In a pieced together lab with nothing new. With benches, we bought at garage sales. There it was. Something that companies with 25,000 engineers couldn’t do. And there we were 100 of us.
Usually, first-generation designs make trade-offs to make the initial release. Where do you go from here?
AF: Bigger, faster, better.
You know, it’s a great question. The artificial intelligence workload is enormously hungry for compute. As we shrink, the amount of time it takes to train a model, from a month to a minute from a week to a second, the ML practitioner can test 1000 new ideas in the same amount of time.
Now, we gotta do better. Now we need to make it yet another order of magnitude faster so that we can invent new models and run them blisteringly fast. So we can not be constrained by the previous 25 years of AI, which now we’re getting a chance to really run. But rather look forward and say, Well, if we had this huge compute landscape, what could we build? It’s our belief that the universe of algorithms that learn is very large. The portion of which of that universe that we’ve tested today on GPUs very small. We have all this unexplored area to run on bigger, faster machines, and I think we will get better at all the existing AI work and we will be able to do things that we’ve never been able to do before.
Cerebras has said that designing material to keep the ~1 million power delivery posts attached thereby solving thermal expansion challenges was a significant effort. Will that pose a significant challenge moving WSE to new process nodes?
AF: So I’ll take a step back and if you have lots of little chips in a cluster, you end up spending a huge amount of power, time and together. In the putting Humpty Dumpty back, there are tremendous inefficiencies in getting them to work together. So you use an aggregate a huge amount of power.
Our approach was not to break Humpty Dumpty up. While we use vastly less power in aggregate, it was all on the equivalent of “one sheet of paper.” It was localized. That had pros and cons, the cons were you had to deliver a lot of power to a small space. That was hard.
The pros were, you could invest in a more complicated cooling structure. You could use water, which had historically been inefficient for one at a time servers. By consolidating increasing your thermal density, it allowed you to use a more complicated, more expensive cooling system because it was amortized over a very large number of compute units. That’s part of the benefit you get as a system builder. If you just sell chips. You don’t get to play with that. We saw that as an opportunity. Now we knew that delivering power to a chip that delivered more performance than 100 or 1000 GPUs was going to be a very big challenge. That took a long time to solve.
It took collaboration with vendors.
It took going back to basics.
It took good engineering methodology where you try something and you learned but it didn’t work all the way. You sit back and you thought about how to run two more experiments. You did this again and again and to solve these problems.
When we are done, not just did we know how to cool and deliver power to one chip, but we know the challenges the materials, the manufacturing techniques, and have developed equipment to deliver power to and cool the most power-hungry chips on Earth. Larger and smaller. We’ve invented materials that allow us to mitigate some of the challenges that you described of thermal expansion. We have collaborated with partners and built an ecosystem that allows us to solve this systemically. It’s not a one-off. We know how to cool twice the power. We had a cool half the power. We know how to cool eight times the power. As we go bigger, faster, better we know both the techniques that have worked the things and the “bugaboos” to avoid.
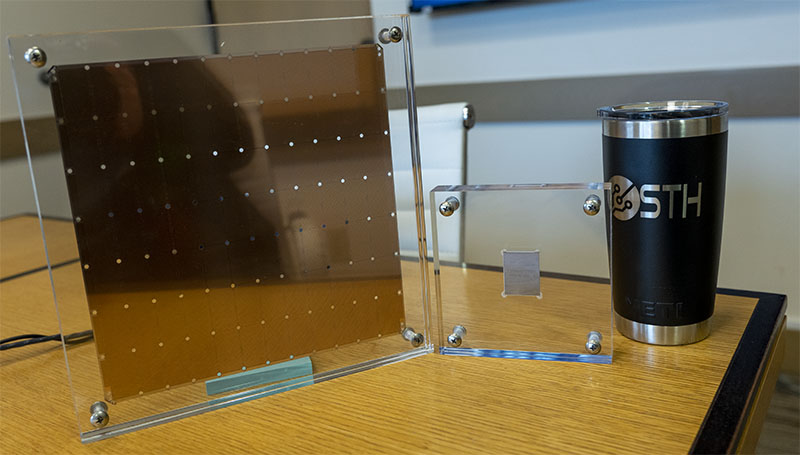
Cerebras CS-1
What were the top 3 hardest design challenges designing the CS-1?
AF: Building the packaging was was all three.
The package is usually something that you don’t think about at all in chip design. In the last 20 years most people who say they do chip design, only do two, sometimes three of the five or seven steps in making a chip. That’s the rise of fabless semiconductors. Companies like TSMC have allowed small companies, and big, to not own their own fab. How we can get away with that is we do the architecture, and we do the logic. Sometimes we do the physical design. Then we hand that to TSMC. They’ve got a factory and they convert that into a chip. Then another company does a package for it and it’s delivered back to us as a finish tested part. That’s the way the fabless semiconductor industry works.
We had expertise up and down that stack. We had to invent a package. We had to invent the way this giant chip would sit on a motherboard. So not only do we have to invent how to make a chip this big, which was bigger than any chip and bigger than any equipment had ever been designed, but then we had to say, all right, how do we put it on a motherboard when there wasn’t even equipment that could pick it up? At the simplest level, the machines that put chips on a printed circuit board couldn’t pick up chips this big. How do we deliver power to it? How do we cool it? Those were extraordinarily challenging problems because they cut across areas that are obscure in the extreme. Surface mount technology manufacturing equipment are the machines that put chips on printed circuit boards. The materials that sit between the chip and a printed circuit board are called the package. There are a very small number of companies in the world that have any expertise in that. We had to build it because they didn’t want to take a flyer on a tiny startup. Now we have world-leading expertise there.
These were problems, that truth be told, we didn’t know were as hard as they were when we started. The onion just kept producing more onion and as you peel. It seemed like the onion would never stop. But we believed in our methodology. We believed in a disciplined engineering approach where each failure is an opportunity to learn. You step back and you documented what worked well and what you did better. You move one variable at a time. You debugged and you thought about it. You did this again and again and again and again. You test and you automated your collection of data so that your data could be compared across hundreds of such experiments. We got better and better and better, then it was a, “Holy cow. We’re doing this.” Now it’s not are we doing it, it’s working every time now. Now we do it like chicken dinners. Just knock them out.
What do typical CS-1 deployments look like? Are customers buying one system, ten systems, more?
AF: First, this is a big fast machine. It’s not for everyone, right? If you’re happy with one or two, or four, or six, GPUs or a few CPUs or you are using them in the cloud, that’s great. You should do that to prove the power of AI.
As you go to make meaningful deployments, especially in very large companies, whether it’s in spaces such as pharma, large enterprises more generally, pharmaceuticals, the finance space, heavy manufacturing, oil and gas, or more generally these large Global 500 type companies, there’s an opportunity there. There’s an opportunity in the mega data center guys. These are the guys who are gathering data every day. These are companies like Google, Facebook, Rakuten, Yahoo! Japan, and their equals in China and in Latin America, it’s another segment.
The supercomputer guys have these giant computer clusters that are doing traditional work. Tying that together with the fastest AI computer allows you to do a giant experiment to link together and allocate to the supercomputer what it’s good at allocate to the AI accelerator what it’s good at. We have deployments there. Finally, there are military applications such as looking at satellite data. There is a collection of such applications. We have customers there as well.
A typical deployment begins with one CS-1. Often they are contractually committed to buy more than one at the start after a proof of concept and acceptance.
Can we talk price?
AF: You can ask and I am going to say no. We don’t share price publicly.
PK: Conceptually, it is my guess that the price of a CS-1 is somewhere less than if I were to go by a cluster of DGX-1’s and the Mellanox networking to put it together.
AF: Exactly right. That’s the right way to think about it. You know, we are a tiny fraction of what you would spend to deliver this much compute any other way. We take minutes to deploy. You roll it in. You plug it into a rack. You hook together 100GbE links and you’re good to go. It can take a month to deploy a big cluster of GPUs. Then you have to modify your software and you have to tune it. Not only are we vastly faster, but per unit of compute we are vastly lower power, use less space, and are faster to deploy.
What is the GTM strategy? Is this going to be selling boxes, or is Cerebras developing an as a service model?
AF: We have a subscription offering where we put the hardware on your site, but it comes under a subscription license. We are working on a set of ways where academics and graduate students could get it. We intend to work with with with the largest cloud guys so they can offer it as a service.
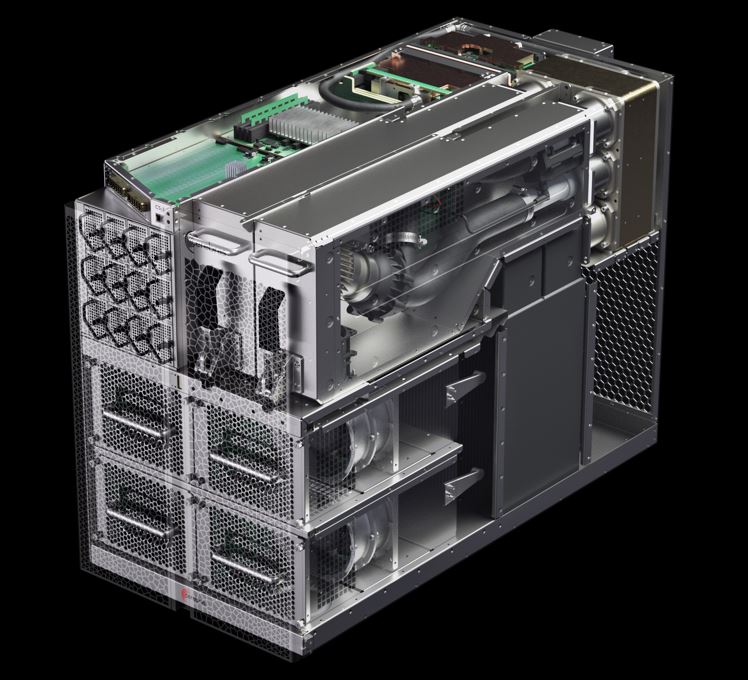
Software and Applications
What is the onboarding process like to CS-1 platform from a software standpoint?
AF: I would say we aim to make it trivial. We aim to make it so that you point your TensorFlow model at our kernel, type tf /cs1 run. Our graph compiler and our compile stack take in your work and it converts it into a config file for our CS-1. It sends back an acknowledgment that says I’m ready. Then you point your data at it. We aim to make it dead trivial.
Are there certain domain areas (e.g. computer vision or NLP) that Cerebras is better equipped to handle than others? If so, what are those? If not, why?
AF: I think you have to be when you build a dedicated piece of hardware, you have to be very honest about what you’re good at what you’re not good at. We don’t do rendering. We don’t do graphics. We don’t do 64-bit double-precision supercomputer work. There’s a lot of work that we’re not the right machine for. But if you’re doing graph compute, if you’re doing artificial intelligence and other examples of graph computation, we are the perfect machine. Every decision was made for that. No decisions were made to help other markets that we’re not good at. So we are a finely tuned dedicated machine for that market.
Are there certain domain areas that are not well suited to the CS-1, and why? For example, because they are too small.
AF: I think there are a couple of answers. One is there are problems that are are are too small for a minivan too. Right? You don’t need a minivan when you just got married, right? There are two of you.
I think building chips and building systems you tackle a problem. If you fit nicely into GPU, you ought to go to the cloud and get one.
That’s not our target. Our target is those who have some of the largest and hardest training problems and not people who want to spend 3000 or 5000 on a single chip. We’re comfortable with that. I think you can’t be the silver bullet for everybody. It’s very hard to be tall and fast and strong and smart, right? So you think about what we’re going to be good at what we are best in the world at is for the hardest and largest, most complicated of the artificial intelligence problems and at those we’re unparalleled.
Are you seeing any pipeline challenge? A college student is likely developing on their NVIDIA GPU so they grow up with CUDA-based platforms. Is there a plan to focus on expanding developers?
AF: Sure those programs are impressive. But I think in this we were very much helped by Google and Facebook. They sat back and they said, for the first part of our lives, we were limited to a single chip vendor, Intel x86 world. AMD really wasn’t a competitor there. Lisa’s doing great work. And we’re huge fans of hers. But this was the thinking over the past 10 years. And now we’re moving to GPUs. There’s no way we’re going to be in a single source environment again. There is no way that that’s going to happen.
So they invented TensorFlow and they invented PyTorch to gut CUDA of its ability to create lock-in. If you’re doing ML work right now, you’re writing TensorFlow or PyTorch. In Japan, maybe you are writing in Chainer. At Baidu, you may write for Paddle Paddle. They are all as different as, I don’t know, chocolate and rocky road. I mean, they are Python-based library languages and frameworks. They were designed specifically so that you could take your model across any hardware. I mean, that was Google stated objective with TensorFlow. They could take it to a CPU, they could take to a GPU, they could take it to the TPU, and they can take it anywhere else. That created an opportunity for those of us who will build dedicated hardware to bolt on to an open-source framework designed to support multiple hardware platforms. That really weakened NVIDIA’s story around lock-in. We want kids to play games. We want more girls in particular to play games and to get fired up about the power of compute when it’s fun. Then to take that passion forward and to know that you can write software that works on many different types of hardware. That you should think about what the right hardware is when you write your software.
When are we going to see MLPerf results?
AF: I don’t spend a minute thinking about MLPerf. MLPerf is a place where Google and NVIDIA can dedicate hundreds of engineers because they have tens of thousands of engineers to tune and game individual models that make them profoundly fragile, and to do a single thing where they can run months worth of hyperparameter testing, to achieve a performance goal. That’s not what customers want. Customers want flexibility. They want a whole family. So as a startup, we’ve dedicated our time to working with our customers to tune their models to show their performance. Our customers are some of the largest owners of GPUs today. Many of our customers own tens of thousands of GPUs, and they’ve chosen not to use them for this work. They had plenty of time to test and they’re choosing something else. That’s sort of our thinking. We’ll get there eventually. But we’re not going to you know, step off the path to the pot of gold to pick up a nickel.
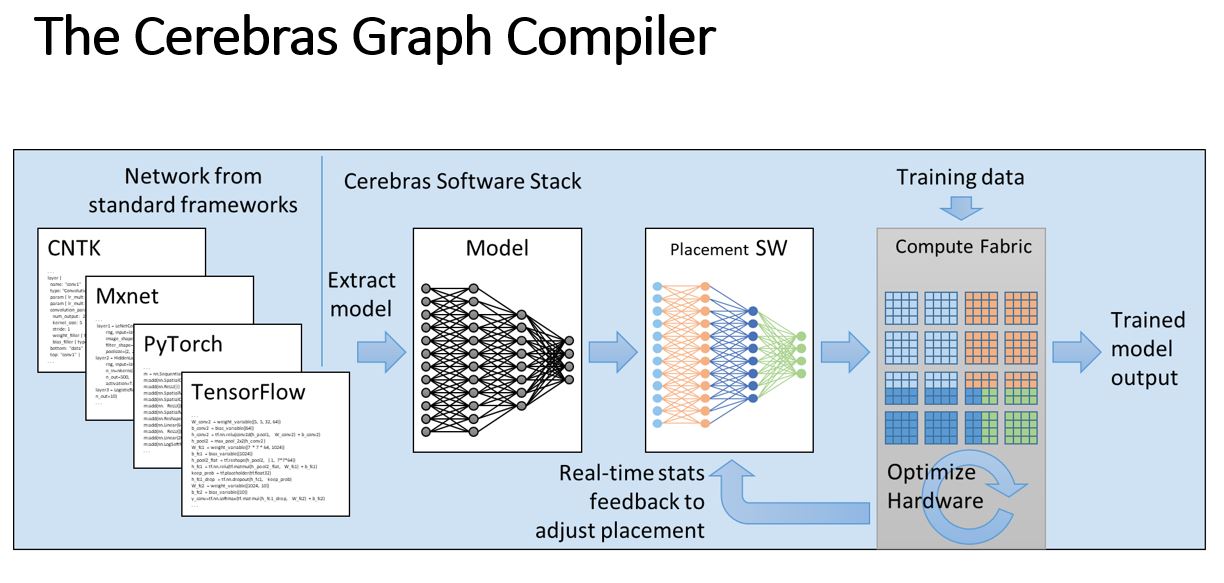
Final Thoughts
You are part of a team that is advancing human capabilities through technology. What problem that you see today are you most hopeful that Cerebras can fix, and why?
AF: I agree with that. That’s what we’re trying to do. That’s why we get up every day.
Well, that was one of the reasons we began with Argonne [National Laboratory] is because of the cancer project. Cancer has not been kind to my family.
It was either help sell ads or cancer. I think we care a lot about both. How people spend their life and enjoy their life is really important too. But we chose to allocate our time towards cancer models. I envision a day where you can take a photo of a lesion on your wrist with a $20 phone in the third world and send it up to the cloud and get a response about whether this looks cancerous for free at the level of the finest dermatologist today. Anywhere in the world, you get that checked.
I envision a world where our mammograms don’t vary by whether your radiologist is getting divorced or is tired and I’m not blaming them. I think we all have days that are better than other days. Right? But what a radiologist learns in Cleveland doesn’t improve what a radiologist is doing in Des Moines. Whereas, as we get better, all of the training gets better. Right, when a Google car hits a pothole in Mountain View, the entire fleet is updated. Imagine if you could update the entire fleet, of radiologists or dermatologists, or pathologists.
I think we will be able to make tests for clean water free and easy. I think there’s a huge opportunity there. I’m personally very interested in a set of innovations around hearing aids. They are sort of have been medieval to date. They’re big. They’ve got a battery and they beep. My mother-in-law takes them off so she can’t hear the beeping. I mean that the whole thing is terrible and in combination with a phone and some training, imagine if you could learn to amplify the voice loved ones, rather than sort of amplify all the background noise on top of everything.
I think those are our areas that are powerful. I think it is easy to discount the progress we’ve already made because we’re still in batting practice, not even in the first inning. But when my mother-in-law at 87 asks Alexa to play Frank Sinatra and plays a song she had forgotten she knew. She then begins telling a story about the first time she met her husband and how they were playing this Frank Sinatra song. How cool is that? I mean, it pulled out of a random library and it created a moment of joy. As we age as a population, and populations particularly if you look at demographics in places like Japan are aging, if we can use tools like this to bring moments of joy, I mean, how cool is that? I think that’s one of the great pleasures of being in this every day.
The other thing is, is that some of the guys here were with me in the 90s when we were building some of the first fast switches and routers. We didn’t know that somebody would later build an application called Skype, Viber, or WhatsApp, which would change communication completely. But we knew that if you drove down the cost of IP networking, good things would happen. We put our brick in the wall. There were a collection of other companies who sort of by building blisteringly fast switching devices, took IP networking to the next level and drove down the cost to zero. Then smart guys came in. They built on our infrastructure and made communication free.
When I grew up, my family’s from Australia, my mother spoke to her mother three minutes a week, at $4.95 a minute. What we’d say to my grandmother was “Hello,” and she’d say, “put your brother on, it’s expensive.” That that was the conversation every week with your grandmother. Before she died at 98, I have a photo of my grandmother with a headset on Skyping with her great-grandchildren all over the world for as long as they wanted. That’s why we love building infrastructure is we are very sure that others will have extraordinary ideas, things to train problems to solve that they’ll use our infrastructure to do what we don’t have to think of.
Next, we have what we saw on our Cerebras lab tour and some final thoughts around the interview and visit.
Can’t wait to be able to take one of these babies for a test drive on the cloud! I wonder how fast a BERT pre-training would take…
Great article. I have three transcription/typo errors you can correct:
We came up with four or five tenants, and one of them was, it should be purpose-built. => tenets: a principle or belief, especially one of the main principles of a religion or philosophy.
“the tenets of classical liberalism”
Often they are contractually committed to buy more than one at the start attending a proof of concept and acceptance. => attendant: occurring with or as a result of; accompanying.
“the sea and its attendant attractions” (although I admit that attending has a correct meaning for this sentence, but it is seldom used). … attendant a proof …
and at those were unparalleled => we’re
This is an superb interview! Kudos to ‘Serve the Home’ for pulling it together.
It highlights the elements that make American style innovation so effective, good engineers who know whom to rely on and financial intermediaries who understand the issues, yet are willing to trust the engineers.
Holy balls that’s properly poking the actual limits of what kind of computing humankind can currently achieve.
What kind of power can a single wafer pull? I would guess around 5-10kW? Does it require facility water cooling?