The NVIDIA Titan V is officially out. Although it has four video outputs, it is not intended for gaming. Instead, the NVIDIA Titan V is intended for workstation development environments that need floating point precision beyond FP32. The real target audience is very easy to see: the deep learning / AI crowd. Like the NVIDIA Tesla V100, the Titan V has the 640 Tensor Core array along with the 5120 CUDA cores. This is the technology those involved in deep learning want.
Why the NVIDIA Titan V is a Watershed Moment
If you wanted to use 1-2 Volta class GPUs in a system, the NVIDIA Titan V is going to be the hot commodity. Tensor performance is rated at 110 TFLOPS versus 112 for the Tesla V100. Memory is down to “only” 12GB per card v. 16GB and the bandwidth is 653GB/s v. 900GB/s on the Tesla V100, but it is relatively close. The L2 cache has also been cut from 6MB to 4.5MB so it is correct to think of this as a lower memory bandwidth and capacity version of the Tesla V100.
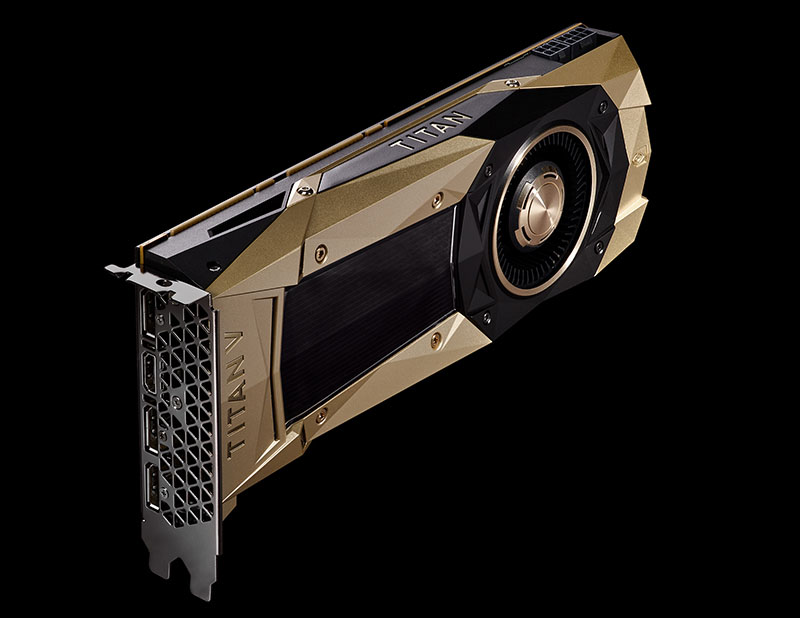
One of the biggest changes here is pricing. Whereas the Pascal-based Titan Xp sold for around $1300, the new Titan V is around $3000. NVIDIA is clearly pricing these chips at a premium as it knows there is a gaggle of Kaggle developers who will flock to the new architecture.
At the same time, the part tells us two other tidbits about future products signaling why this is a watershed moment.
First, since the number of CUDA cores and Tensor cores match the Tesla V100, these are not GV100 dies that are binned down for compute. That means that NVIDIA is likely to have a pile of chips for upcoming products based on binning. Undoubtedly, NVIDIA will sell through a production run or two of Titan V before announcing lower-end products.
Second, the Tensor Core is something NVIDIA is signaling it is going to charge a premium for. Instead of holding the $1300 or a $1500 price tag, NVIDIA more than doubled the Titan card price point in a generation. While AMD has raw compute performance, they do not have the accelerators like the Tensor Core for deep learning with this generation. As a result, NVIDIA can charge more for its deep learning parts.
One of the questions we posit often is whether NVIDIA needs Tensor Cores in its consumer GPUs. NVIDIA has flourished partly because one could use a gaming GPU for CUDA applications. One part of NVIDIA clearly is pushing to price deep learning acceleration at a premium. The other part is trying to keep this key competitive advantage intact. The more silicon devoted to Tensor Cores means a costlier consumer gaming part so at some point one would expect gaming parts without that silicon.
Final Words
We have some results of NVLINK and 8x Tesla V100 v. P100 training in our queue. The new Titan V has a huge customer base that is flush with VC / large corporation money and ready to jump onto a new architecture at the $3000 price point. We are excited to see how the Titan V performs.
Nice card to learn how to work with tensor cores(ai) and software that uses fp64. No NVLink so small systems only and yes some will use it for gaming.