As we pen this article, the NVIDIA H100 80GB PCIe is $32K at online retailers like CDW and is back-ordered for roughly six months. Understandably, the price of NVIDIA’s top-end do (almost) everything GPU is extremely high, as is the demand. NVIDIA came out with an alternative for many AI users and those running mixed workloads in the enterprise that is flying under the radar, but that is very good. The NVIDIA L40S is a variant of the graphics-oriented L40 that is quickly becoming the best-kept secret in AI. Let us dive in and understand why.
We just wanted to thank Supermicro for supporting this piece by getting us hardware. Since they managed to get us access to so many hard-to-get NVIDIA parts, we will say they are sponsoring this piece.
We also did a video since many folks saw this piece. You can find that linked above.
NVIDIA A100, NVIDIA L40S, and NVIDIA H100
First, let us start by saying that if you want to train foundational models today, something like a ChatGPT, then the NVIDIA H100 80GB SXM5 is still the GPU of choice. Once the foundational model has been trained, customizing a model based on domain-specific data or inferencing can often be done on significantly lower-cost and lower-power parts.
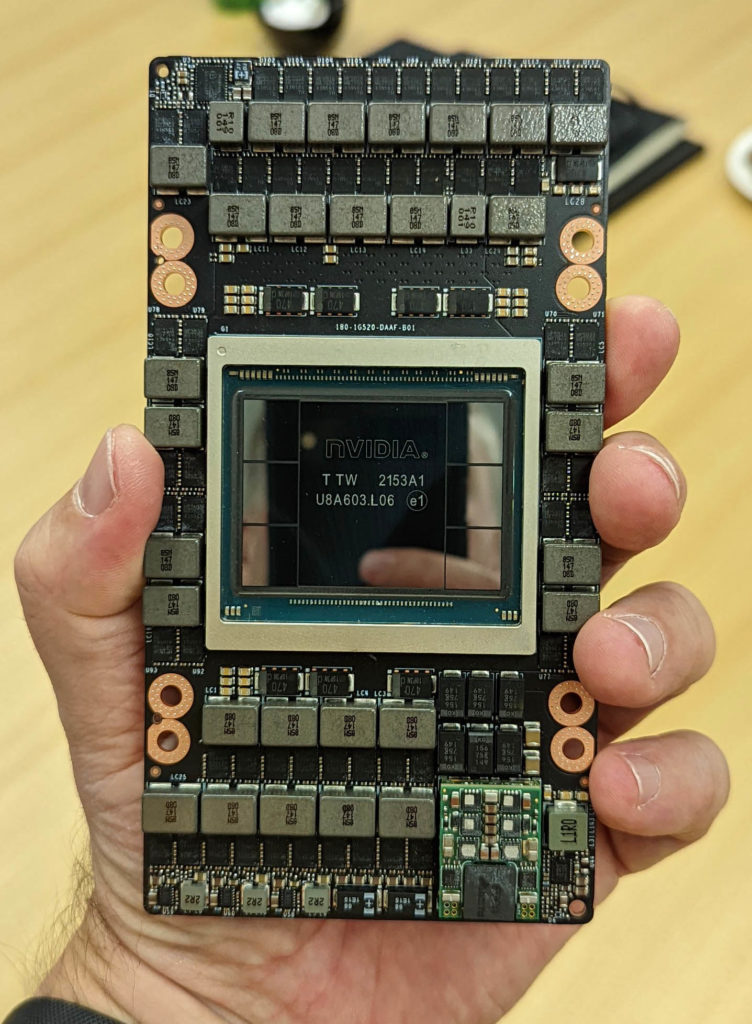
These days, there are three main GPUs used for high-end inference: the NVIDIA A100, NVIDIA H100, and the new NVIDIA L40S. We will skip the NVIDIA L4 24GB as that is more of a lower-end inference card.
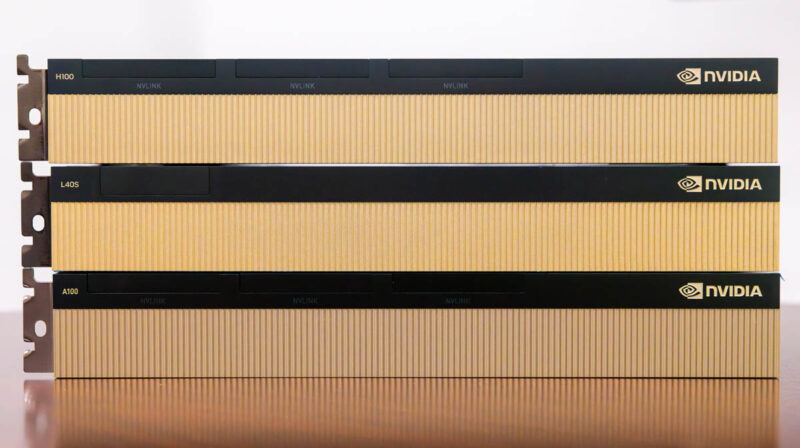
The NVIDIA A100 and H100 models are based on the company’s flagship GPUs of their respective generations. Since we are discussing PCIe instead of SXM modules, the two most significant differences between the form factors are NVLink and power consumption. The SXM modules are designed for higher power consumption (roughly twice the PCIe versions) and to be interconnected via NVLink and often NVSwitch topologies in multi-GPU assemblies. You can see an example of one of the highest-end systems available in our recent Supermicro SYS-821GE-TNHR 8x NVIDIA H100 AI server piece.
The NVIDIA A100 PCIe was launched in 2020 as the 40GB model, and then in mid-2021, the company updated the offering to the A100 80GB PCIe add-in card. Years later, these cards are still popular.
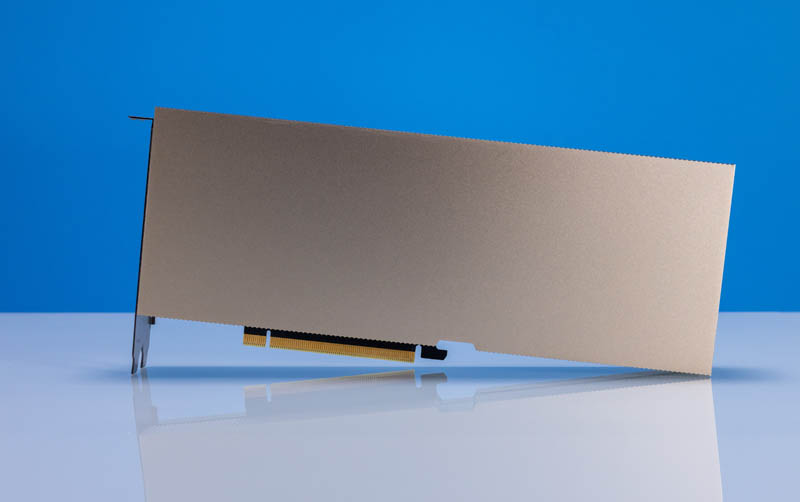
We first got hands-on with the NVIDIA H100 SXM5 module in early 2022, but systems started showing up in late 2022 and early 2023 as PCIe Gen5 CPUs became available.
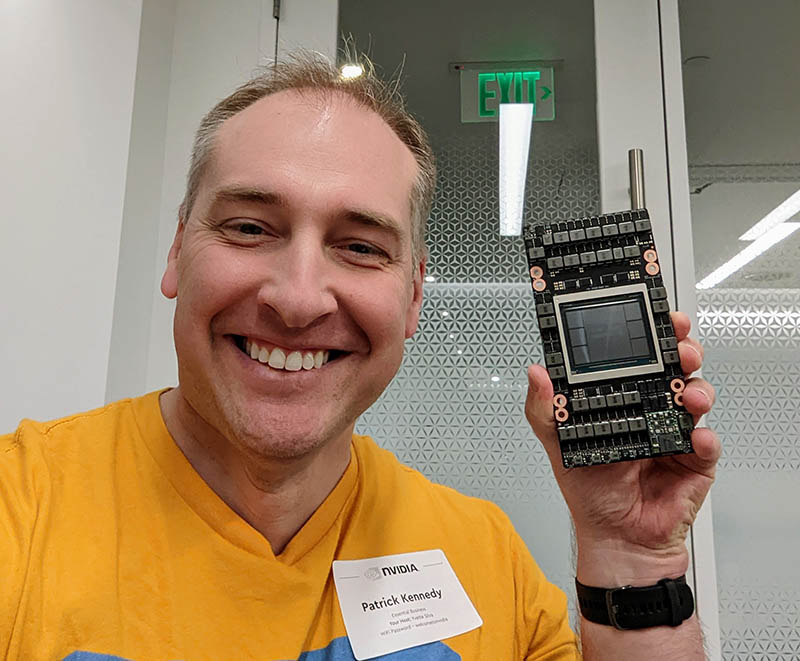
The NVIDIA H100 PCIe is the lower-power H100 designed for mainstream servers. One way to think of the PCIe card is a similar amount of silicon running at a different part of the voltage/ frequency curve designed for lower performance but also much lower power consumption.
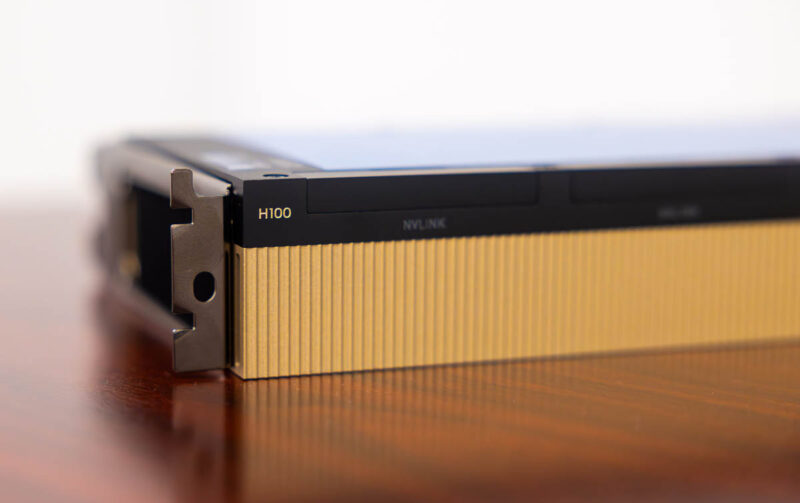
There are some differences even within the H100 line. The NVIDIA H100 PCIe is still a H100, but in the PCIe form factor, it has reduced performance, power consumption, and some interconnect (e.g., NVLink speeds.)
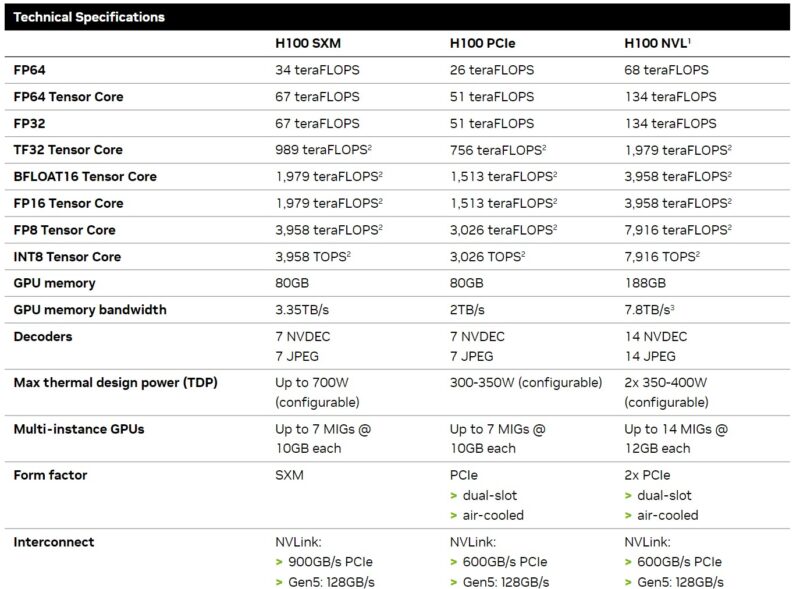
The L40S is something quite different. NVIDIA took the base L40, a data center visualization GPU using NVIDIA’s newest Ada Lovelace architecture, and changed the tunings so that it was tuned more for AI rather than visualization.
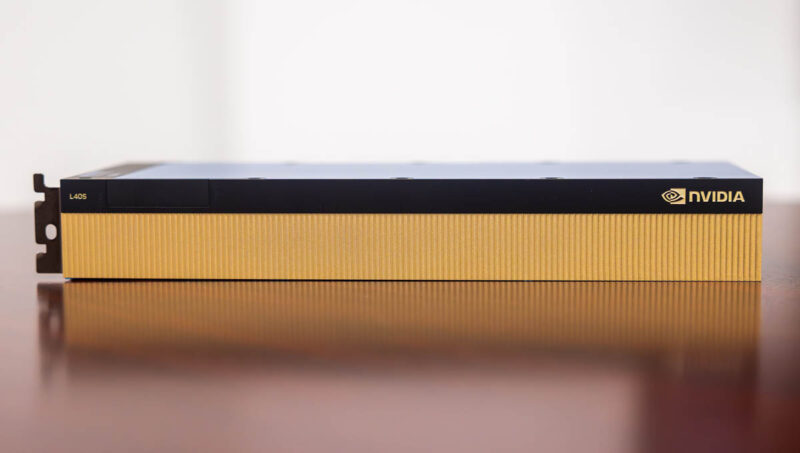
The NVIDIA L40S is a fascinating GPU since it retains features like the Ray Tracing cores and DisplayPort outputs and NVENC / NVDEC with AV1 support from the L40. At the same time, NVIDIA transitions more power to drive clocks on the AI portions of the GPU.
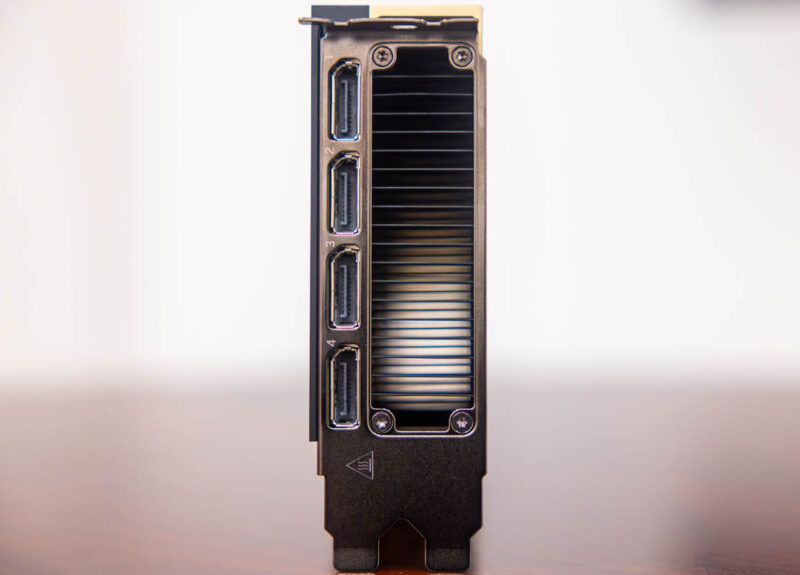
We put this on a chart to make it easier to visualize. NVIDIA’s specs sometimes vary even based on the NVIDIA source one views, so this is the best we could find and we will update it if we get an update to the specs. We also included the dual-card H100 NVL that has two enhanced H100s with a NVLink bridge between them, so treat that as a dual-card solution while the rest are single cards.
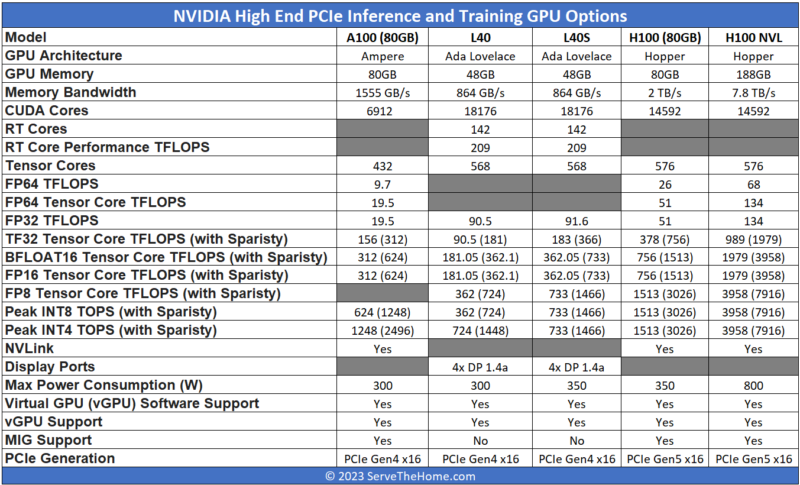
There are a few points worth looking at here:
- The L40S is a massively improved card for AI training and inferencing versus the L40, but one can easily see the common heritage.
- The L40 and L40S are not the cards if you need absolute memory capacity, bandwidth, or FP64 performance. Given the relative share that AI workloads are taking over traditional FP64 compute these days, most folks will be more than OK with this trade-off.
- The L40S may look like it has significantly less memory than the NVIDIA A100, and physically, it does, but that is not the whole story. The NVIDIA L40S supports the NVIDIA Transformer Engine and FP8. Using FP8 drastically reduces the size of data and therefore, a FP8 value can use less memory and requires less memory bandwidth to move than a FP16 value. NVIDIA is pushing the Transformer Engine because the H100 also supports it and helps lower the cost or increase the performance of its AI parts.
- The L40S has a more visualization-heavy set of video encoding/ decoding, while the H100 focuses on the decoding side.
- The NVIDIA H100 is faster. It also costs a lot more. For some sense, on CDW, which lists public prices, the H100 is around 2.6x the price of the L40S at the time we are writing this.
- Another big one is availability. The NVIDIA L40S is much faster to get these days than waiting in line for a NVIDIA H100.
The secret is that a new common way to get ahead on the AI hardware side is to not use the H100 for model customization and inference. Instead, there is a shift back to a familiar architecture we covered many years ago, the dense PCIe server. In 2017, when we did DeepLearning11 a 10x NVIDIA GTX 1080 Ti Single Root Deep Learning Server cramming NVIDIA GeForce GTX 1080 Ti’s into a server was the go-to architecture for even large companies like search/ web hyperscalers in certain parts of the world and autonomous driving companies.
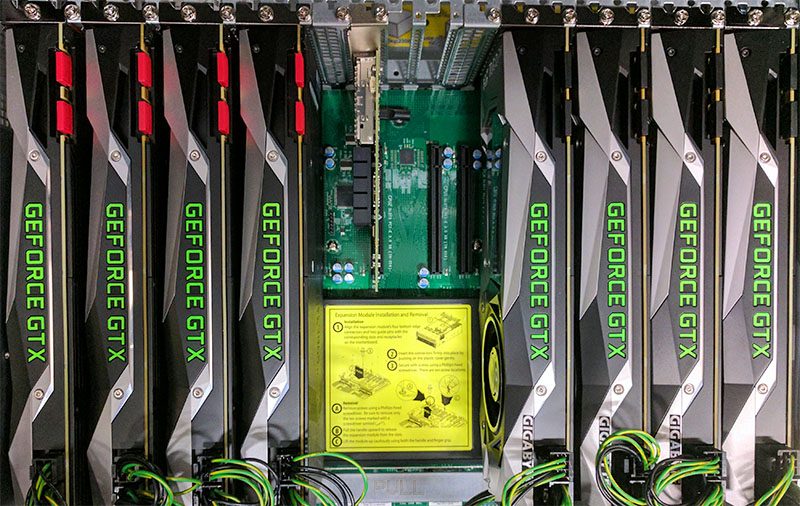
NVIDIA changed its EULA to outlaw this kind of configuration, and it is making its software focus more on the data center parts for AI inference and training, so things are different now.
In 2023, think about the same concept but with a NVIDIA L40S twist (and without server “humping.”)
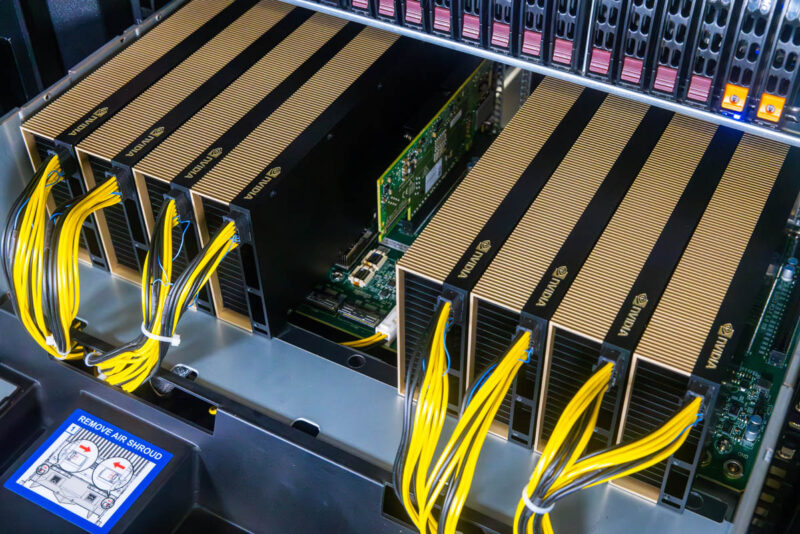
One can get similar performance, possibly at a lower price, by buying L40S servers and just getting more lower-cost GPUs than using the H100.
Next, let us dive into that a bit more.
The L40S is basically the datacenter version of the RTX 4090 with double the memory. Just shows you that Nvidia is so dominant in this segment that they can purposefully hamstring their consumer GPU to protect their datacenter cards.
That nVidia hamstrings the *102 cards for half precision is well known. They’ve been doing it since Ampere IIRC. Note how this L40S has no FP64 at all… can’t be offering anything that makes a general purpose GPGPU card for less than top dollar. nVidia has only offered this card because it can’t get enough chips for H100: if it was not supply constrained then nVidia would *never* offer this.
Perhaps AMD’s ROCm code getting knocked into shape even on Navi3 has some effect as well.
Which is better, 4x L4 cards or 2x L40S cards? Working on a budget here.