NVIDIA L4 Key Specs
The NVIDIA L4 is a data center GPU from NVIDIA, but it is far from the company’s fastest. Here are the key specs of the new GPU:
GPU | NVIDIA L4 |
---|---|
FP32 | 30.3 teraFLOPs |
TF32 Tensor Core | 120 teraFLOPS* |
FP16 Tensor Core | 242 teraFLOPS* |
BFLOAT16 Tensor Core | 242 teraFLOPS* |
FP8 Tensor Core | 485 teraFLOPs* |
INT8 Tensor Core | 485 TOPs* |
GPU memory | 24GB |
GPU memory bandwidth | 300GB/s |
NVENC | NVDEC | JPEG decoders | 2 | 4 | 4 |
Max thermal design power (TDP) | 72W |
Form factor | 1-slot low-profile, PCIe |
Interconnect | PCIe Gen4 x16 64GB/s |
The 72W is very important since that allows the card to be powered by the PCIe Gen4 x16 slot without another power cable. Finding auxiliary power in servers that support it is easy, but what made the T4 successful was not requiring that support.
NVIDIA L4 nvidia-smi Output
Here is a quick example of what the NVIDIA L4 looks like from nvidia-smi.
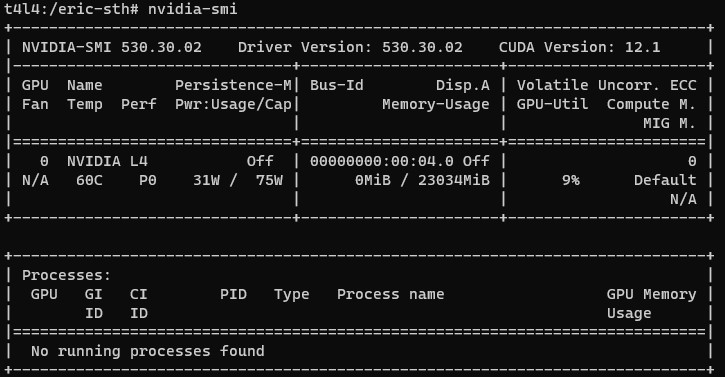
We know folks like to see that.
NVIDIA L4 Performance
On the L4 side, we grabbed containers to run some MLPerf 3.0 “style” workloads. These are not official submissions, but here is what we saw trying to replicate what the server vendors showed.
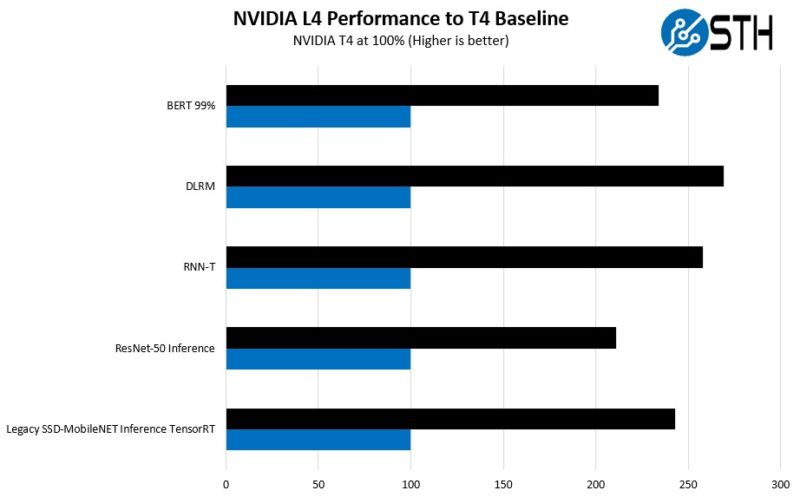
Again, directionally, we are similar in terms of the generational increase between the T4 and L4. While the exact values vary, our simple guidance is to think about the L4 as a 2-3x improvement in performance per card over the predecessor. What is interesting, is that data center CPUs are increasing AI inference performance at a faster rate than this, but GPUs still have a big place in AI inference since one can simply add cards to scale out.
NVIDIA L4 Power Consumption
In terms of power consumption, we were getting typical power consumption in the 50-65W range under use. We saw nvidia-smi cross 70W being used but we were also not using all GPU memory so there was likely a bit more. The spec sheet says the card can do 72W. We say 75W just to stay safe.
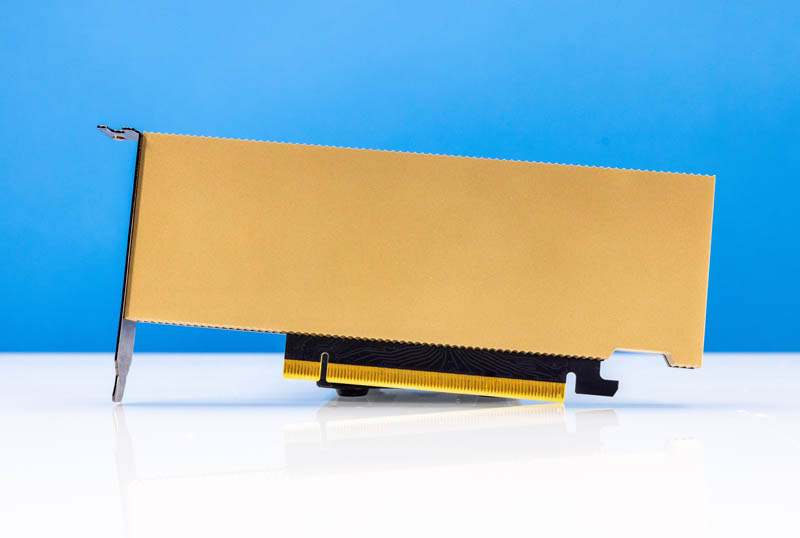
The sub-75W power consumption allows the card to be PCIe slot powered. It also means that the card can often fit in server configurations without having to increase chassis fans for cooling or power supplies for power.
Final Words
While much of the focus today is on the NVIDIA H100 and Generative AI inference at large scale, there is an existing, and rapidly growing AI inference market in general purpose servers. The NVIDIA L4 is NVIDIA’s secret weapon to allow organizations to add CUDA hardware to just about any server, even smaller edge-focused servers.
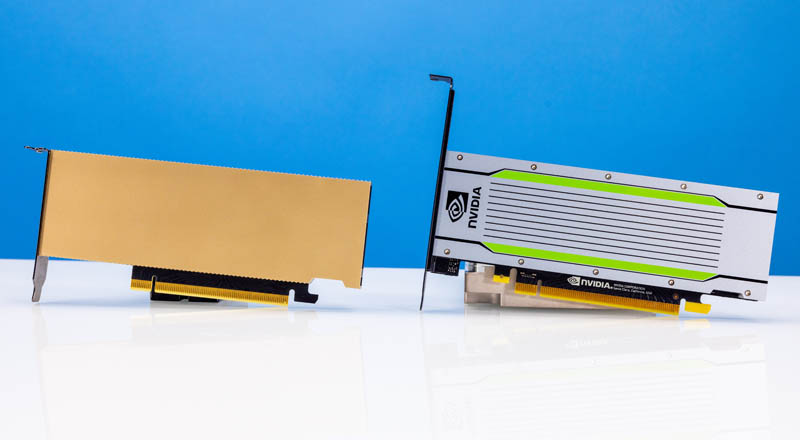
The NVIDIA T4 was a very popular card, and we expect the NVIDIA L4 will be as well, especially given the environment for AI hardware.
Thanks again to PNY for helping get us a card to review.
I believe this card is sized just about perfectly to fit into a TinyMiniMicro node. I just can’t justify spending thousands of dollars extra on a GPU like this for home use when I could use a consumer-oriented 3090 or 4090 for a fraction of the cost in a different system. That datacenter markup annoys me, but Nvidia certainly loves it. Obviously, the main problem would be cooling the card outside of a server chassis… so it would probably be thermally throttled, even if you pointed a fan at it, but for hobbyist use, it would probably still be better than anything else you can fit into a TMM node.
Josh – there were 3D printed 40mm brackets for the T4. Those do not fit the L4 due to the size of the inlet side. My sense is there will be a new round for the L4 as they become more popular.
On the GF/W metric it’s excellent. Also the GF/slot metric.
On the GF/W metric it’s excellent. Also the GF/slot metric.
Josh – why would you want to do that kind of thing in a tiny mini micro form factor? You could not find a form factor with worse thermal management challenges.
Patrick Kennedy – Do you think you’ll ever see an Intel Data Center GPU Flex 140 to review? They supposedly don’t need a license to unlock SR-IOV capabilities. This will be interesting to compare against an L4 for VDI stuff.
I agree with prox, getting a look at those intel datacenter GPUs would be really cool
Who knows prox. Good question though.
@Josh “That datacenter markup annoys me”
Nvidia forbids to deploy Geforce to datacenter with their license agreement. IMO the existence of those products, its only raison d’être is fabricated from this licensing restriction. There is no reason to buy these in hobby usage.
@emerth @yamamoto The reason to buy these in hobby usage and such a form factor is the same reason this site focuses on TinyMiniMicro. For home use, 1L PCs are an excellent size for a server. Using something massive is possible but unappealing, and it is extremely hard to find HHHL GPUs with any significant amount of VRAM for experimenting with ML at home. Each person’s use cases are different.
I’m interested in a card like this precisely so that I can avoid needing a 3090 (or multiple) to get the need VRAM for ML inference on large models. Where the the workstation equivalent that has video output and a fan? The RTX 4000 Ada SFF comes close but still has less VRAM.
@Josh – home use, home lab, all great. I do it too. Just, you will find it a lot easier to cool them in a case where you have the room to build ducts and fan mounts. If you just point a fan at them you will fail, they require real forced air.
A card like this would be a slam dunk for the Thinkstation P360 Ultra. It’s bigger than TinyMiniMicro, technically, but it’s on the same class as the Z2 Mini G9 and Precision 3260. Plus, the P360 Ultra has a dedicated fan for MXM/CPU2; this means there’s a Lenovo design blower that can be adapted to this GPU!