Power Tests
For our power testing, we used AIDA64 to stress the NVIDIA RTX 3090 FE, then HWiNFO to monitor power use and temperatures.
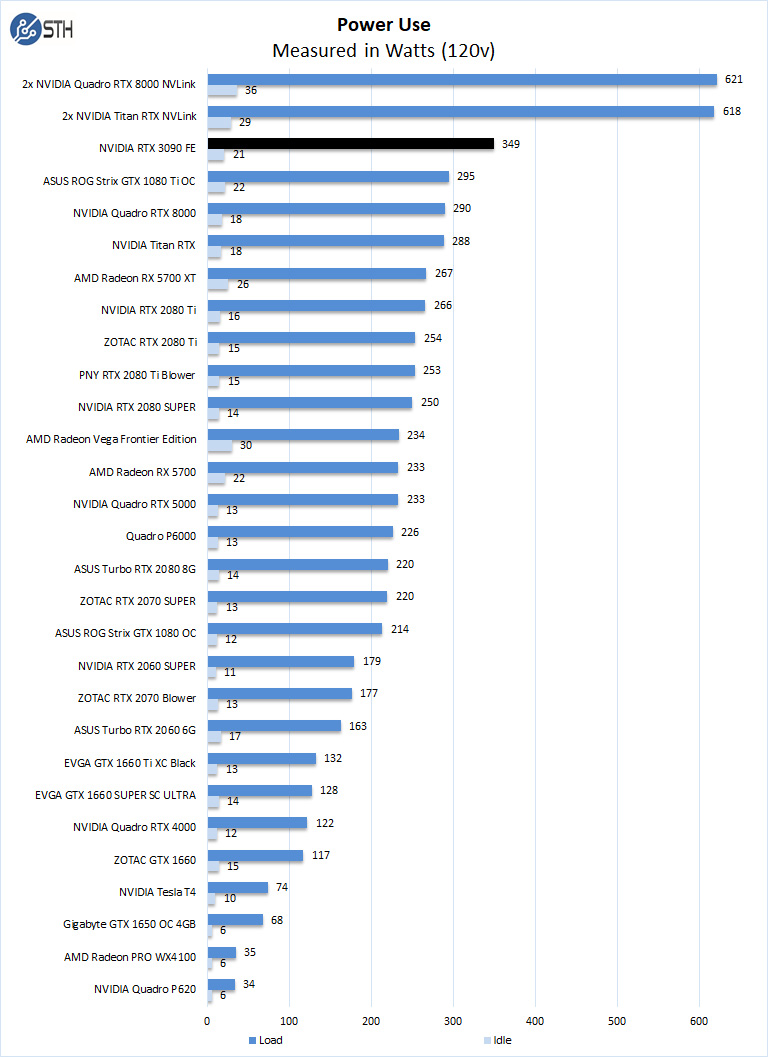
After the stress test has ramped up the GeForce RTX 3090, we see it tops out at 349Watts under full load and 21Watts at idle. While that extra 50-60 watts may seem important in the context of single GPUs, as we showed in our compute performance testing, users that are buying these cards for compute performance will see a massive performance per watt benefit.
Cooling Performance
A key reason that we started this series was to answer the cooling question. Blower-style coolers have different capabilities than some of the large dual and triple fan gaming cards.
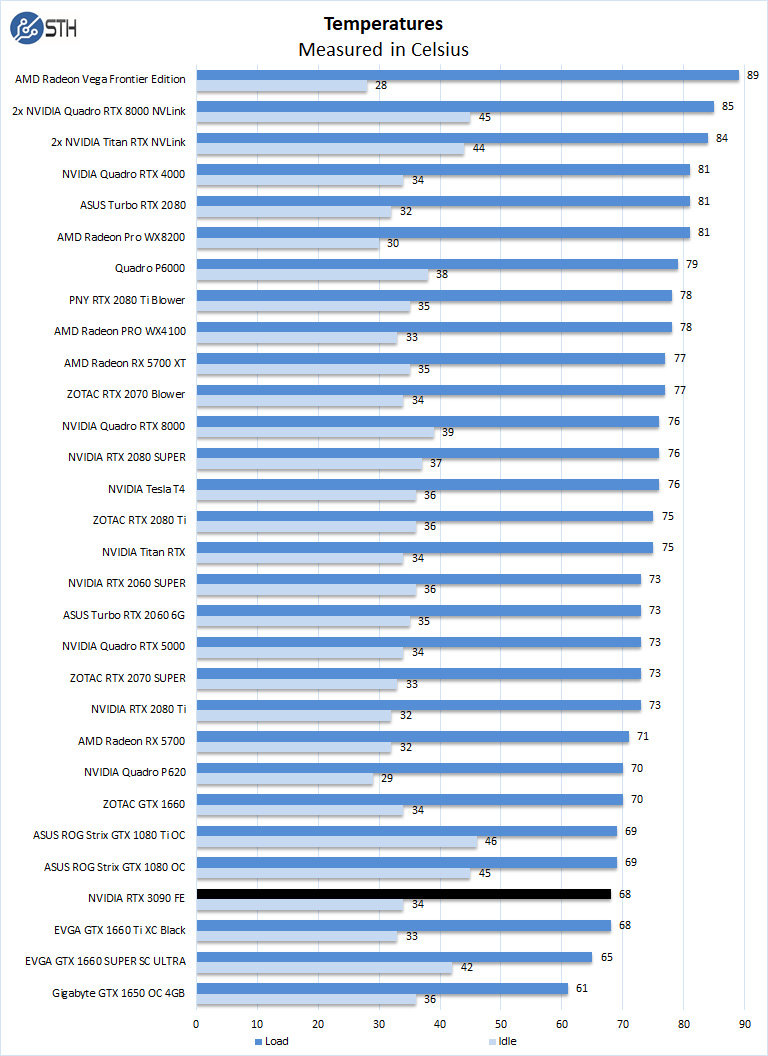
Temperatures for the GeForce RTX 3090 ran at 68C under full loads, which shows NVIDIA’s new cooling solution performs very well and makes very little noise during compute workloads. Idle temps we saw were 34C, which is also excellent for a GPU of this size.
At the same time, this is a series we started years ago to look at blower cooler GPUs that could be packed densely into workstations and servers. The GeForce RTX 3090 is clearly a long way from that original vision. Since we do not have a timetable to get a blower version, we had to go with what we had access to.
Final Words
If you are a fan of small form factor GPUs, then the NVIDIA GeForce RTX 3090 is probably not for you. Likewise, if you have a hard sub $1000 limit on your GPU budget, then this is simply a more expensive card. If you want your GPU compute to have ECC memory and double precision math for high-end HPC, then this card simply has neither the form factor nor specs. If you want your compute to be on an AMD (and future Intel) GPU, FGPA, or another dedicated accelerator, then this is not going to fit your needs.
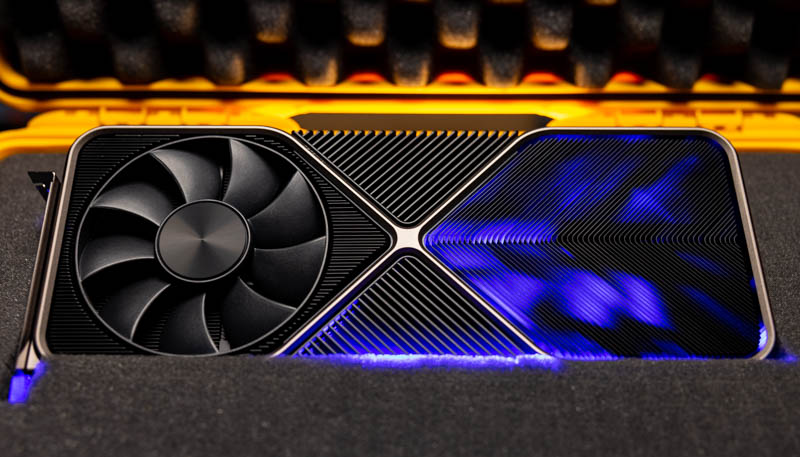
For the rest of the market, let us face it, NVIDIA has a very strong CUDA-based GPU compute ecosystem. For many applications, whether they are pro visualization/ rendering or AI inference and training, NVIDIA has spent years building a software ecosystem that has made its GPUs the defacto standard for GPU compute. In the desktop segment of that ecosystem, the GeForce RTX 3090 provides enormous performance and price/performance compared to previous generations.
If you do have the budget and are an existing GeForce RTX 2080 or Ti to aid in your work in a workstation, and you have the physical room/ power budget to fit it, then the RTX 3090 is far more than a minor upgrade. This is more of a large generational performance step increase than a minor refresh cycle on the compute side. Also, having 24GB of memory put the RTX 3090 into a class of GPUs able to handle larger models and larger batch sizes than previous GeForce cards, matching the Titan RTX and Quadro RTX 6000 in its capacity.
Although others will have covered the NVIDIA GeForce RTX 3090 from a gaming perspective and found it to be more than competent, for STH’s readers of professionals and academics that depend on compute performance this is an enormous change. There is a good reason they have been very difficult to buy since their release. This is the type of GPU launch that can yield transformational speedups in workflows.
Are you using the Tensorflow 20.11 container for all the machine learning benchmarks? It contains cuDNN 8.0.4, while the already released cuDNN 8.0.5 delivers significant performance improvements for the RTX 3090.
Great fp64 performance..
It’s not great fp64. The 3090’s AIDA64 GPGPU score of 638 is less than 10% of the 6351 FLOPS my Radeon Pro VII pulls down. https://twitter.com/hubick/status/1324203898949652480
How did the NVlinked, Titan RTXs and Quadro RTX 8000s get better than 100% scaling in OctaneRender 4.0?
Chris Hubick
‘
Misha, as well known AMD shill, is being facetious – and this is a graphics card and not a compute card like the Ampere A100 – which trades the RT cores for FP64…
Would love to see this dataset run on a A100 for comparison, is that review coming as well or are those datasets not public?
Hi,
I LOVE the GeForce and Threadripper compute reviews (especially the youtube video reviews!)
However, for our work load, we really need to know how the hardware performs for double-precision memory-bound algorithms.
The best benchmark that matches our problems (computational physics) is the HPCG benchmark.
Would it be possible to add HPCG results for the reviews? (http://www.hpcg-benchmark.org/)
Also, for some other computational physicists, having the standard LinPack benchmark (for compute-bound algorithms) would be really nice to see as well (https://top500.org/project/linpack/)
– Ron