MLPerf Training v1.0 results are out. While we still see about 70%-80% of the results are NVIDIA results, we see perhaps a few interesting bits here. There are also a few changes to the benchmark suite this time. Let us get into some of the insights.
MLPerf Training v1.0 New Workloads
MLPerf Training v1.0 retired the GNMT and Transformer benchmarks to make room for new workloads.
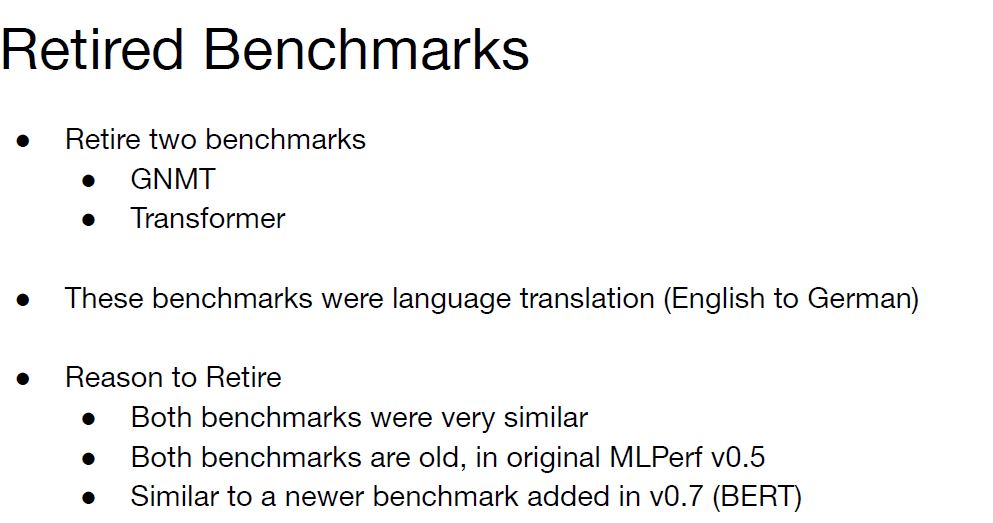
Specifically, the RNNT benchmark is a speech-to-text workload that has been added.
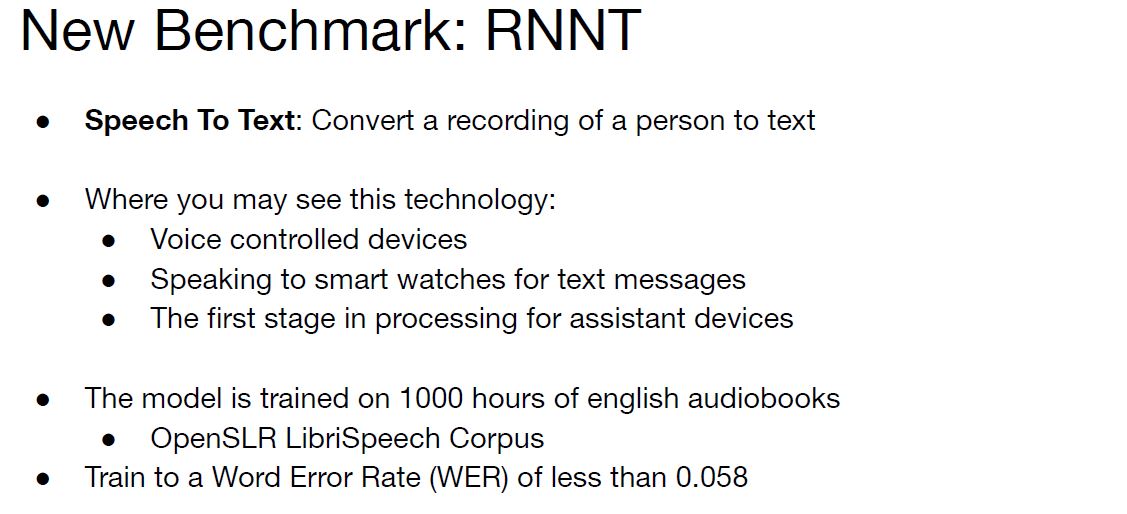
The 3D-UNet bechmark was added as part of showing a medical AI benchmark use case.
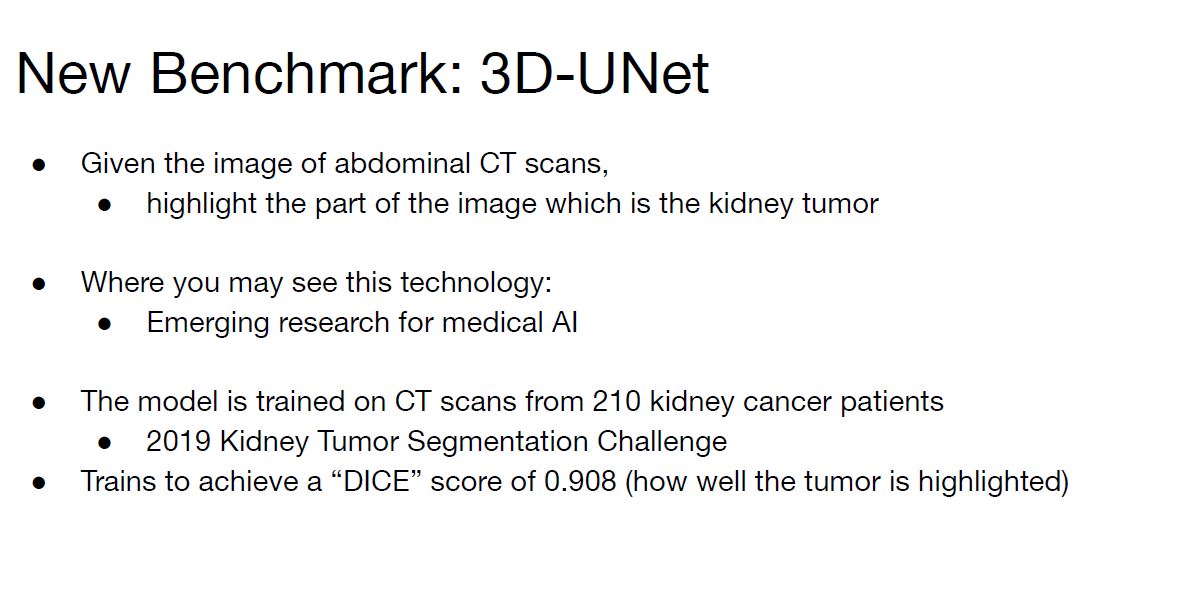
Overall, these seem to be good updates to the suite that makes sense.
MLPerf Training v1.0 Closed Division
One can look up the results, but there were 82 closed division configurations benchmarked. NVIDIA, as is customary, made up the vast majority of submissions either directly or through partner OEMs. NVIDIA powered configurations accounted for around 70% of the submissions. MLPerf historically has been almost a NVIDIA benchmark just due to the number of submissions made by the company relative to others.
This round saw a few interesting bits. Intel’s Habana Labs submitted a SYS-420-GH-TNGR system. We found that this was made by Supermicro and is an OAM system with Intel Habana Gaudi HL-205 processors.
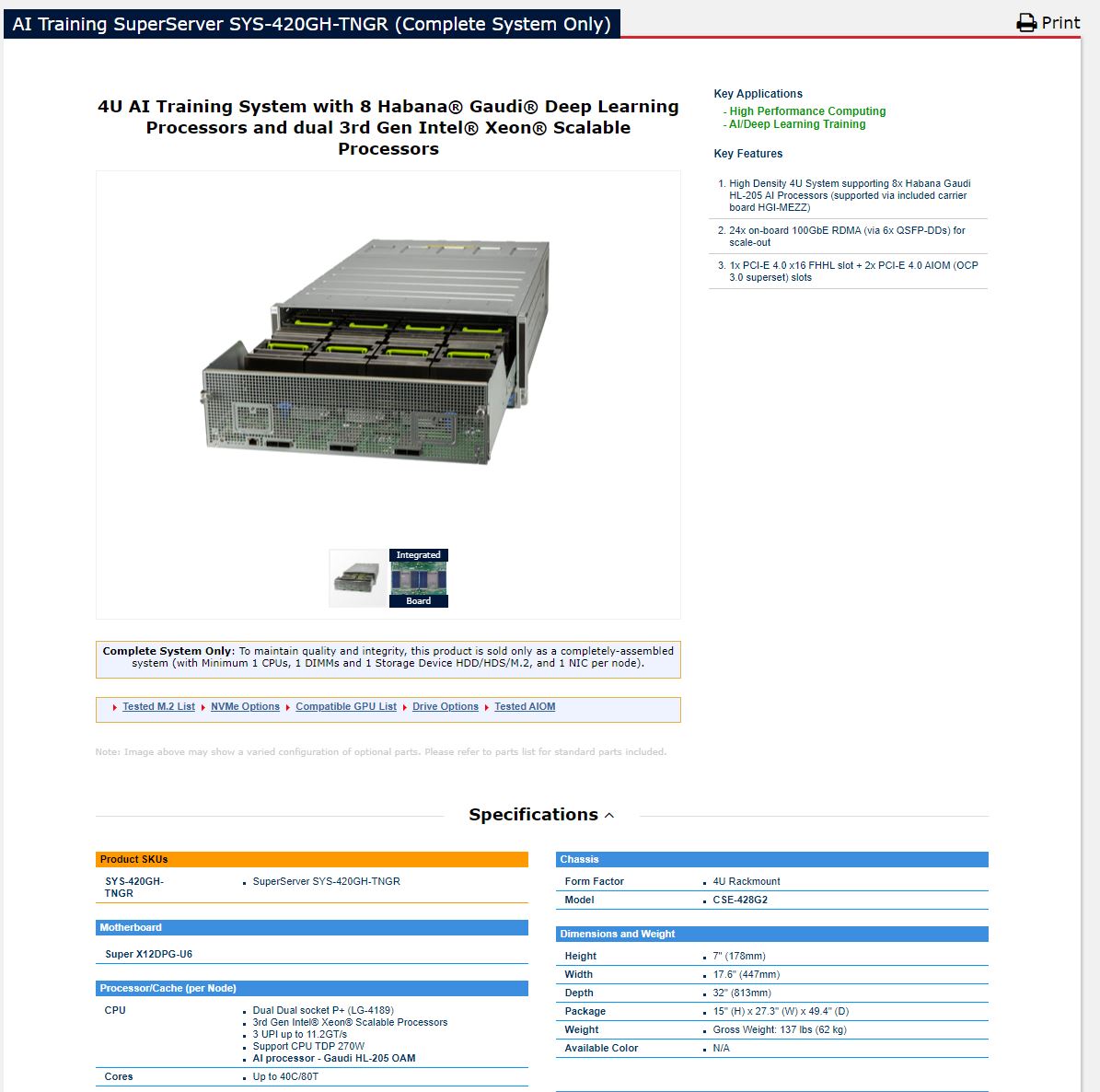
This system will be used in the San Diego Supercomputing Center’s Voyager system with 42 of these 8x AI accelerator systems.
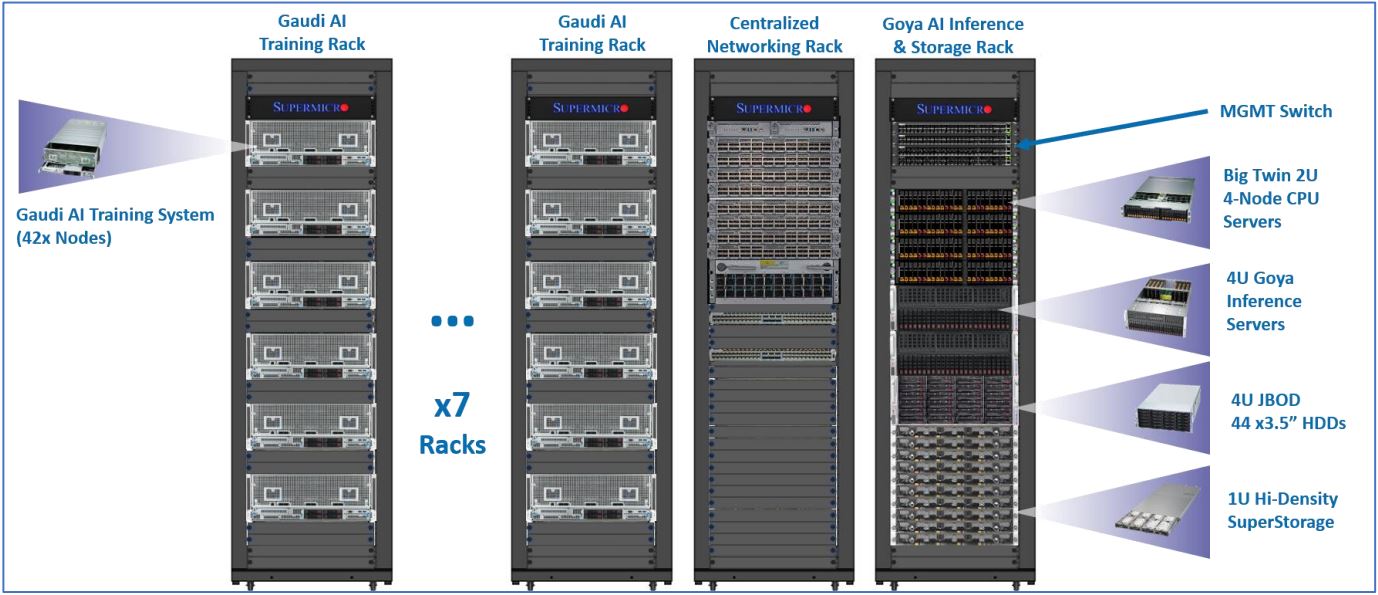
Google also entered the TPUv4 which it says is faster than NVIDIA, although it is in a preview mode. The company says it is using TPUv4 internally and will be available publicly later this year.
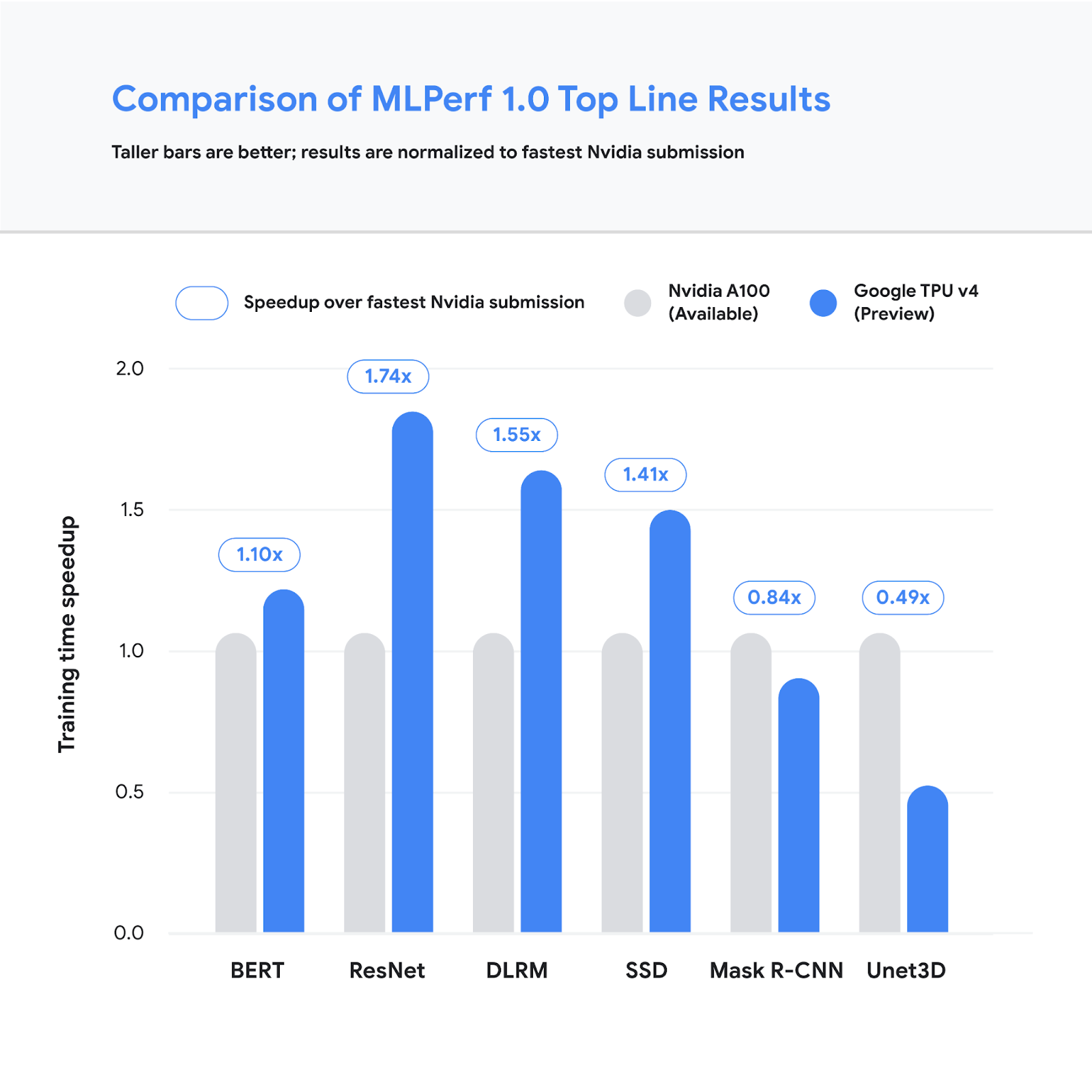
The only results in the R&D and Internal category were based on Huawei’s Kunpeng 920 Arm CPU and its Ascend 910 AI accelerator. This is the technology we were covering at least two years ago on STH. Still, it appears to be only slightly slower than the NVIDIA A100 80GB option albeit hard to tell since Huawei’s options are in their own division and have very few results submitted.
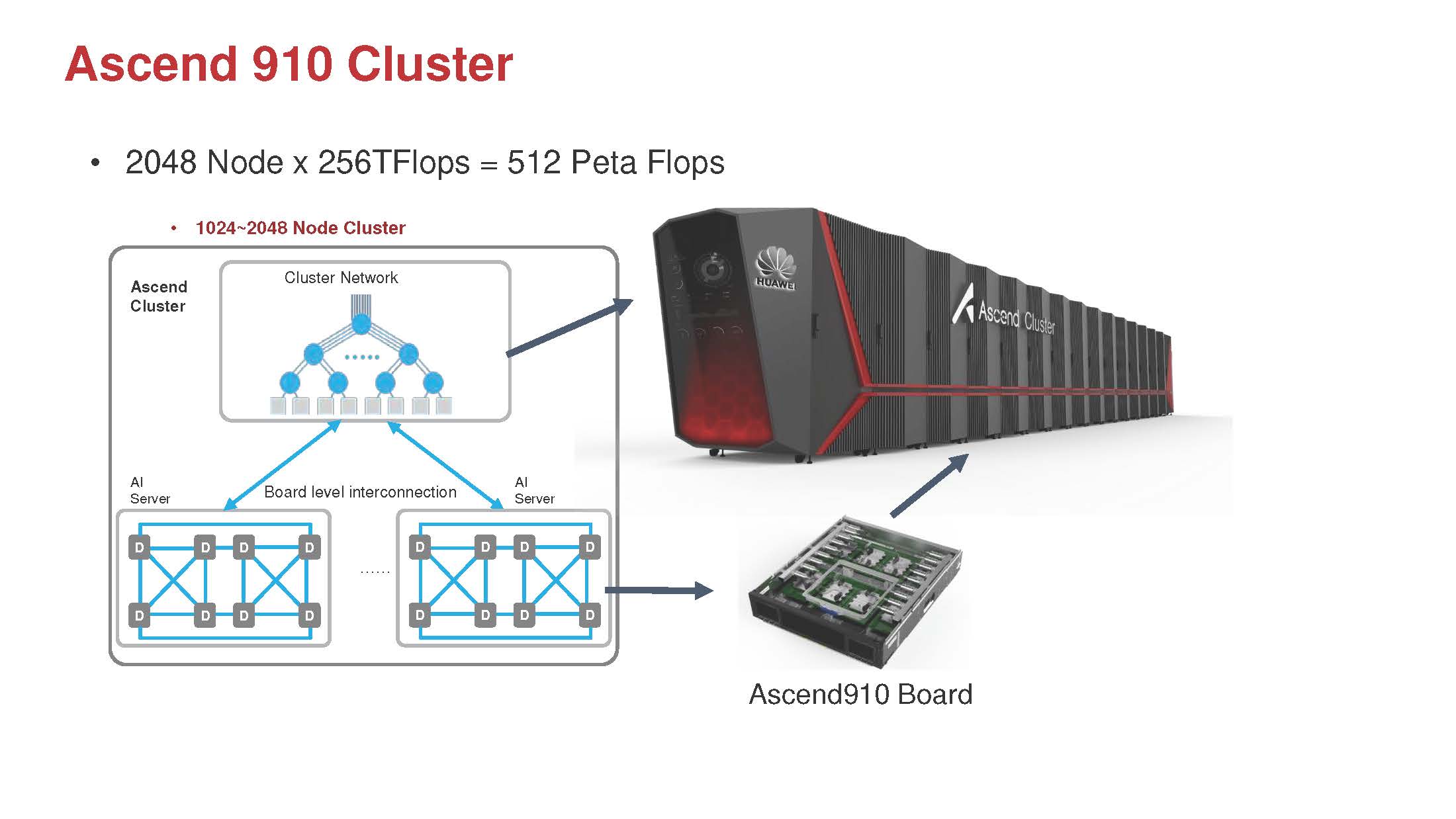
Another interesting note is that the NVIDIA A100 80GB 500W SXM4 module made a number of appearances. This 500W option is not on NVIDIA’s current spec page but we have been discussing it for several months on STH. The 500W module is a case where many vendors either need low ambient temperatures in a data center to cool the module or liquid cooling is required.
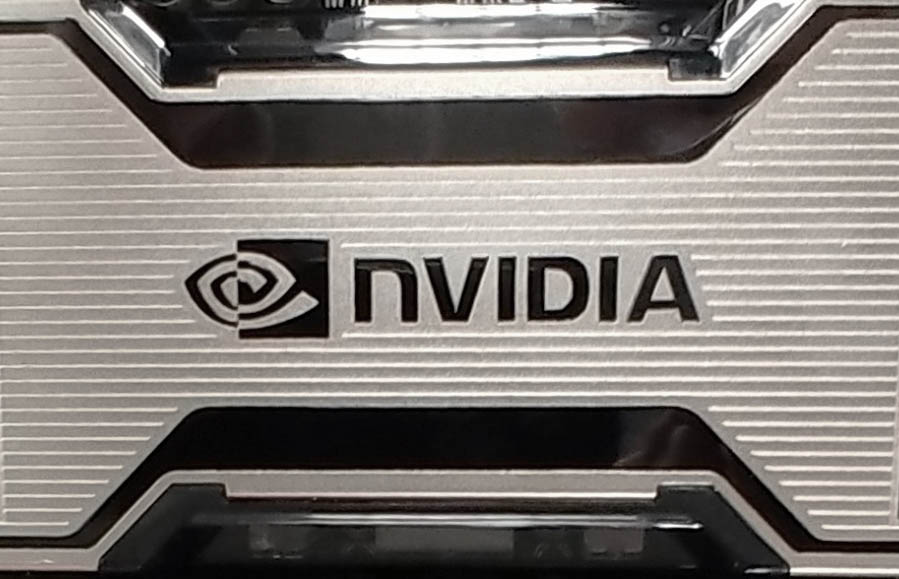
Just some stats for the closed Division:
- Overall, the Available on-prem closed division is still NVIDIA and Intel-dominated. 97% of these results used NVIDIA or NVIDIA technology. The remaining 3% were Graphcore results that it submitted Open Division results to contrast against.
- All of the Intel results but the two results for Habana Labs were basically showing a baseline of CPU performance using Cooper Lake CPUs.
- Effectively the primary division of MLPerf was a NVIDIA-only affair again even though there was a spin to say it was multi-vendor.
- Around 58% of the Closed Division systems were using 2019-era CPUs
- The average system submitted results for less than 25% of the workloads in the closed division.
MLPerf is still basically three points:
- NVIDIA is the dominant participant now that it has leveraged its ecosystem with other AI vendors not wanting to spend resources competing in NVIDIA’s benchmark
- Google will compete to show off its TPUs at scale
- Intel generally just shows a CPU baseline for results
That is OK, but it is hard to have so little diversity compared to what we know is out there and say this is an industry effort.
MLPerf Training v1.0 Open Division
There is not too much to say on this one. There are five results. Each result was a single submission where only one benchmark was submitted out of eight possible workloads.

It seems like there was little interest here. Our sense is that, for example, with the first two Graphcore entries, Graphcore was trying to show that with additional tuning it was 19.5%-21.5% faster than the closed division results.
On one hand, it was good to see Graphcore on the list.
Final Words
It seems like Graphcore perhaps was the loser in this round. Graphcore probably would have been better off not submitting results here instead of taking the strategy other AI chipmakers have and focused on customer engagements rather than using MLPerf. Even if it can claim better performance in some ways of counting accelerators, NVIDIA had faster results.
The contrast to this is the Habana Labs – Intel example. We know Facebook, a big AI shop and infrastructure buyer, decided to select Habana Labs over NVIDIA (and Intel Nervana) causing Intel to buy Habana Labs. What is strange here is that either Facebook made a poor choice with a staggering sum of money, or Gaudi is actually very good. Still, we do not see it represented except with a single configuration and two results.
MLPerf Training v1.0 has made significant strides. At the same time, it feels like this is primarily an effort by NVIDIA to show its AI dominance, with Google showing it can compete at scale, and Intel saying it is still here. That narrative is pervasive in MLPerf and has been for some time. This somewhat makes sense given NVIDIA’s GPUs are the defacto standard in the industry, but then the question is what the purpose of MLPerf Training is other than a server OEM tool to create and meet RFP requirements for NVIDIA system purchases. Still, SPEC’s CPU benchmark results are primarily an Intel v. AMD tool with other vendors rarely participating, so perhaps this is not too different.
“SPEC’s CPU benchmark results are primarily an Intel v. AMD tool with other vendors rarely participating, so perhaps this is not too different.”
That is a fair point.
Still… surely there’s some way to incentivize more non-Nvidia participation?
>>the question is what the purpose of MLPerf Training is other than a server OEM tool
The tool was created by the industry including research and academia as well as hardware companies. The intention is to provide a transperant and evolving way to evaluate the performance of AI hardware and solutions. While I appreciate Nvidia submissions have dominated to this point, I do think, like any emerging technology solution, a central, well quantified and fair tool is useful to everyone (OEMs, customers, and manufacturers). We should not conclude because one vendor dominates, no one else gains utility from it.