MLPerf Inference v4.1 is out. In this generation, there were only 40 edge results submitted which is not a lot. Instead, this turned into primarily a data center inference exercise. MLPerf is typically an NVIDIA affair, but a few others joined this round and provided some exciting results.
MLPerf Inference v4.1 NVIDIA B200 Whallops AMD MI300X
We found two results for single SXM/OAM accelerators. First though, let us get to the AMD provided slides.
AMD was quick to note the Llama2-70B inference results scaled well from one to eight MI300X, the company’s top-end GPU right now.
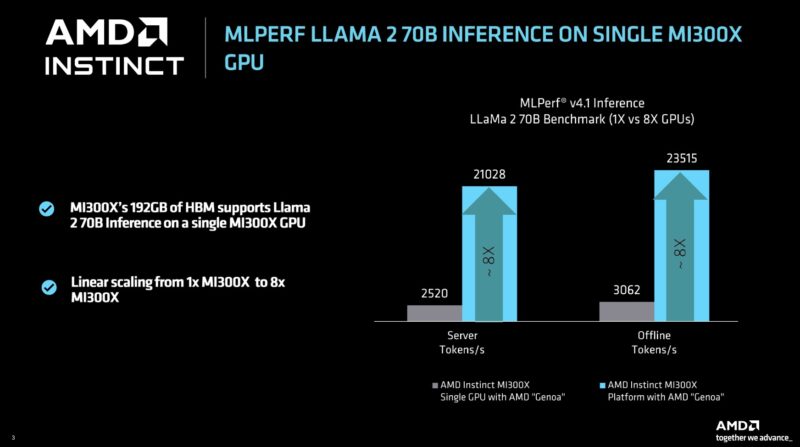
Likewise, it is very competitive against the NVIDIA H100 platform and using AMD EPYC “Turin” expected to release in Q4 it will see further gains (the NVIDIA DGX H100 uses an older processor, but other vendors offer AMD CPUs and NVIDIA GPUs.)
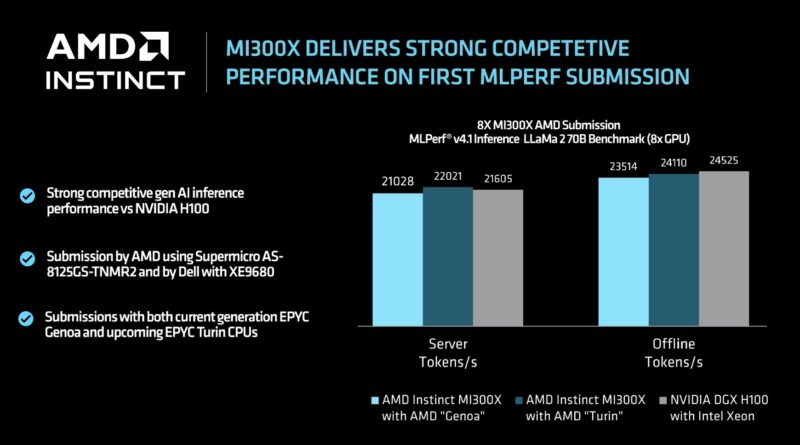
That seemed very exciting until we started looking at the data. Here is a look at the top AMD MI300X GPU at 750W versus the NVIDIA B200 at 1000W:
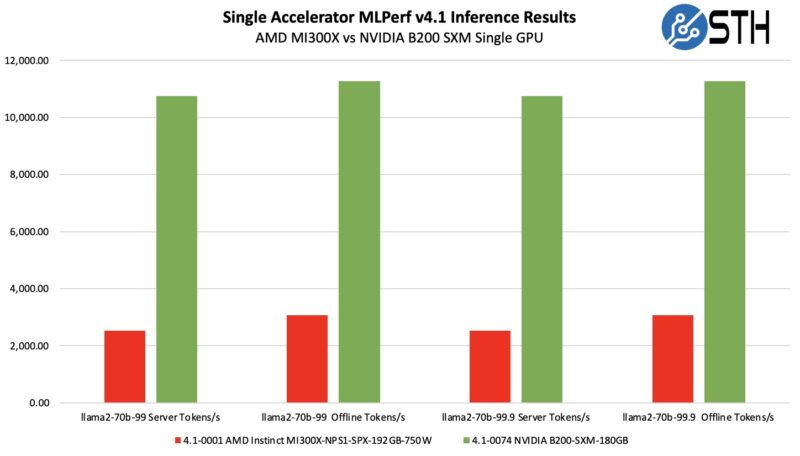
We also found a single GPU result for the NVIDIA H200, and it appears as though NVIDIA is picking up a lot of performance here, at least in the single GPU results.
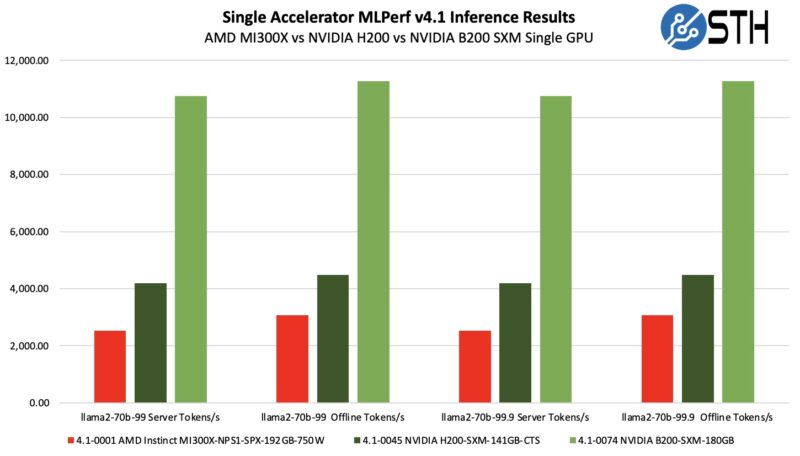
Single GPUs may not be overly interesting for high-end inference, although AMD touts being able to fit entire inference models on a single GPU. At the same time, those are some fairly shocking results. These days lower power is good, but data centers are going to see a result like the MI300X and B200 and see a clear winner. AMD says that the MI300X is a competitor to the NVIDIA H100 while the forthcoming MI325X is a competitor to the H200. Still, in a power limited world, Blackwell is looking like a beast.
UntetherAI Rises Against NVIDIA
UntetherAI showed some great results. In the power tests, it used six accelerators to NVIDIA’s eight H200s. NVIDIA may have led 480K to 310K in the resnet Queries/s and 556K to 334K on the Offline Samples/s side. At the same time, NVIDIA used around 5kW in each case, and UntetherAI used around 1kW. That means the performance per watt results were stellar for the startup.
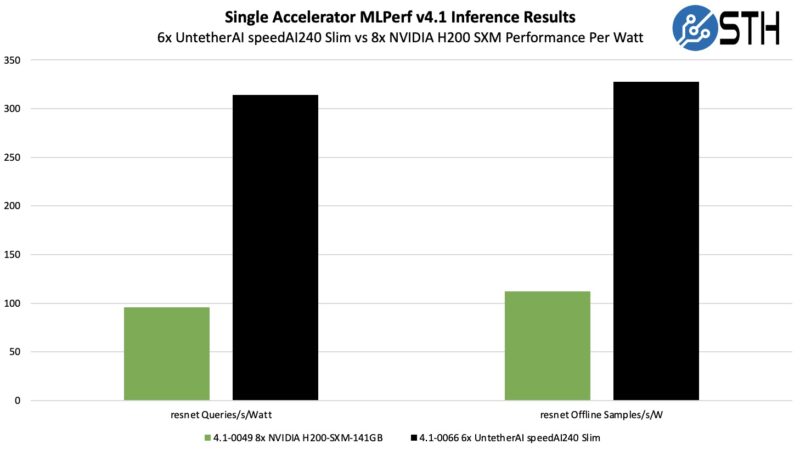
Those are really surprising results for the startup we covered two years ago in our Untether.AI Boqueria 1458 RISC-V Core AI Accelerator Hot Chips coverage.
Final Words
It was a bit strange to have a MLPerf Inference series without many edge devices. Real applications are running every day with computer vision, and other AI applications, so it feels a bit strange that the focus was primarily on data center. At the same time, data center spending is huge, so perhaps that makes sense.
There was quite a lot of new hardware in this, including AMD EPYC “Turin”, Intel “Granite Rapids” 6980P, Google TPU v6e / v5e, and NVIDIA Grace.
My understanding is the B200 is much faster than the H100 and H200 on the low-precision tensor arithmetic used in MLPerf, but the differences for double precision scientific workloads are not so much. I also believe the MI300X was originally focused on science rather than generative AI.
As AI and science have different requirements, it’s not surprising there are trade-offs when designing hardware. As a follow-up, it would be interesting to compare the same accelerators using a benchmark that reflects scientific workloads.
Performance from FP4 to FP8 has doubled (x2).
The die size has almost doubled, from B200 to B100/H100/H200 (~x2).
A fourfold increase (x4) in performance is a reasonable trade-off considering the hardware design.
In terms of die size efficiency, Blackwell simply converts FP64 units to lower precision for AI computing. However, from a power consumption standpoint, this represents a step forward.
H200 vs MI300X seems to be mainly due to the tdp difference. mI300x to B200 seems to be tdp+ lower precision.
I am gonna guess that MI350x is going to be in the similar ballpark as B200 with fp4, but might arrive a bit later (or not given Nvidia remaking masks?)