One of the leading efforts to establish standardized machine learning benchmarks is MLPerf. Today, MLPerf Inference v0.5 has been released. The group behind MLPerf represents some of the largest customers and suppliers of deep learning gear.
Why Inference Matters
Although we have spent years reviewing GPU servers for AI/ deep learning training, the inference is where the money will eventually be. You can see some of the servers we have reviewed as examples here:
- Inspur Systems NF5468M5 Review 4U 8x GPU Server
- Gigabyte G481-S80 8x NVIDIA Tesla GPU Server Review the DGX1.5
- Supermicro 4028GR-TR 4U 8-Way GPU SuperServer Review
- DeepLearning11 10x GPU Server Three Quarters Later Pricing Updates
- Tyan Thunder HX GA88-B5631 Server Review 4x GPU in 1U
These servers have generally used NVIDIA GPUs to power training networks. Training is great, but if one develops a method to solve a problem, one also needs to actually solve problems. That is where inference comes in.
Inferencing hardware is designed to quickly take a series of inputs, run them through the trained network, and generate the appropriate response. This can be telling an autonomous IoT device to move in a certain manner. It can mean identifying who someone is and how they are feeling in a video feed. The key is that this is how trained models will result in actions in the field.
Why MLPerf Inference Matters
The industry needs standard ways to determine what solution is the best for a certain application. This can range from standalone accelerators to SoC inferencing.
With the world of inference, there is an enormous caveat. The speed of the solution is only one component to whether it is the best. It also matters how that solution is priced, packaged, and delivered to users and developers. For example, a 75W GPU may work great in a server, but not necessarily an edge server. A M.2 device may work great in a 5G edge box, but not in a delivery drone. A SoC may have built-in inferencing hardware, but use too much power for an intelligence camera and so forth. MLPerf Inference answers a portion of the question, what is the fastest for their workloads. There is a power working group also dedicated to getting to a power number.
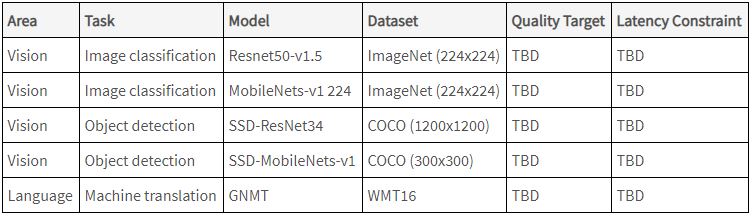
Currently MLPerf Inference is still in a working stage. There are four vision and one language test, but undoubtedly there are other workloads organizations will want to see. Quality Targets and Latency Constraints are not yet finalized. Benchmark results are due next quarter.
If you want to learn more, check out the MLPerf site here.