MLPerf 0.7 results are out, and there is something that was immediately obvious: we need more diversity in results. For the record, I believe MLPerf has great goals, and it is still early in the process, but this release highlights one of their biggest challenges. As we would expect, we see strong results with the new NVIDIA A100 which effectively dominates the data set. Beyond NVIDIA, there are a few notable additions from others that we wanted to point out.
MLPerf 0.7 Results
First, we simply wanted to provide the results so that we can have a discussion around them.
MLPerf 0.7 Closed Division Results
First, we have the cloud results:

Despite having a recent Google Cloud NVIDIA A100 Instance Launch we do not have the Google Cloud A100 instance here. Instead, we still have the NVIDIA V100 using the older 16GB model results. The Google TPU V3 again makes an appearance here. Perhaps the most interesting is the Huawei Ascend 910. We detailed that chip previously, but it is an effort by Huawei to provide an NVIDIA alternative for training. These cloud results are a bit hard to compare since they are of different instance sizes. For example, we get 512 Huawei Ascend 910 cards which number about 64x more than the older 16GB NVIDIA V100 on Google Cloud result, and offer around 56x is the performance. On one hand, that would seem to imply that the Huawei Ascend 910 on ImageNet ResNet is slower than the older generation V100’s, but Huawei is scaling higher so it is hard to compare. These four results are actually the most competitive results in the entire data set.
Next, we wanted to discuss the Preview division for hardware coming out soon.

There are a few bits worth noting here. All of these results are using 28-core Intel Xeon Platinum parts, including the Platinum 8380H and pre-production parts. Although the preview is for upcoming hardware, we covered the 3rd Generation Intel Xeon Scalable Cooper Lake Launch last quarter, and also have our 3rd Gen Intel Xeon Scalable Cooper Lake SKU List and Value Analysis. Effectively, these are the bfloat16 results that Facebook is counting on. The value proposition is twofold. Adding the instruction to CPUs means that while these may not be fast, they offer the performance effectively “for free” since it is in existing infrastructure. Also, if you have workloads that require a lot of memory, CPUs have much more memory than GPUs have with HBM2.
The Research, Development, or Internal division is next:

Here we see 512 Huawei Ascend 910 cards offering solid performance somewhere around 256 Google TPU V4’s. We do not have information on the Google TPU V4, so it is hard to judge if this is good off of a single result where we only know the number not the scale of each solution (nor the power consumption and cost.) Still, that is the point of this division.
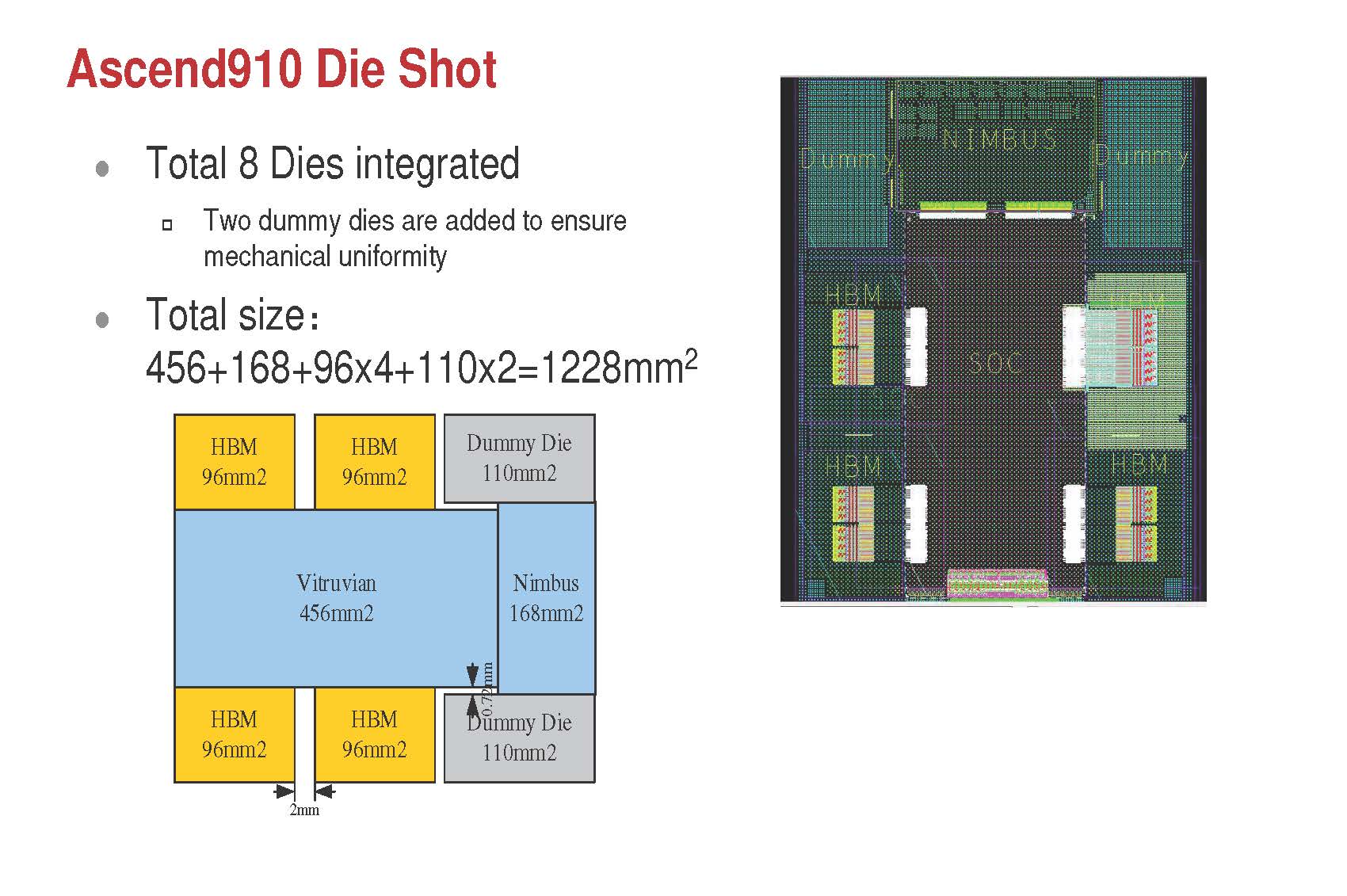
Finally, we wanted to cover the On-Prem division:
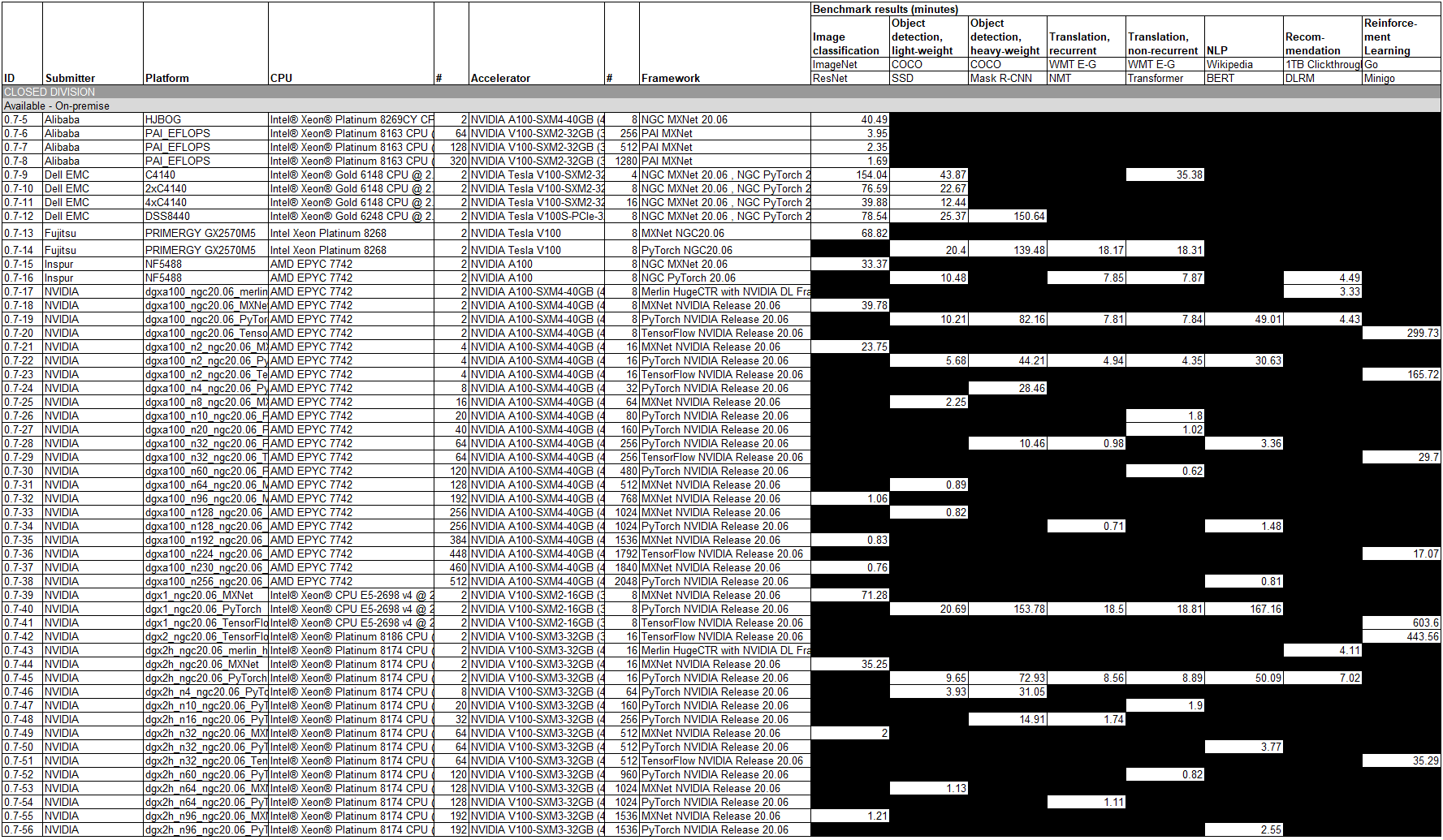
All 52 results used NVIDIA GPUs either the V100 or the newer A100. Effectively this can just be renamed the NVIDIA GPU division at this point.
Dell is still using the old “Tesla” branding that was retired by NVIDIA. Perhaps the most notable result is that the Dell DSS8440 has the only result here using the NVIDIA V100S. Of note, this was the same machine we saw launched with the Hands-on With a Graphcore C2 IPU PCIe Card.
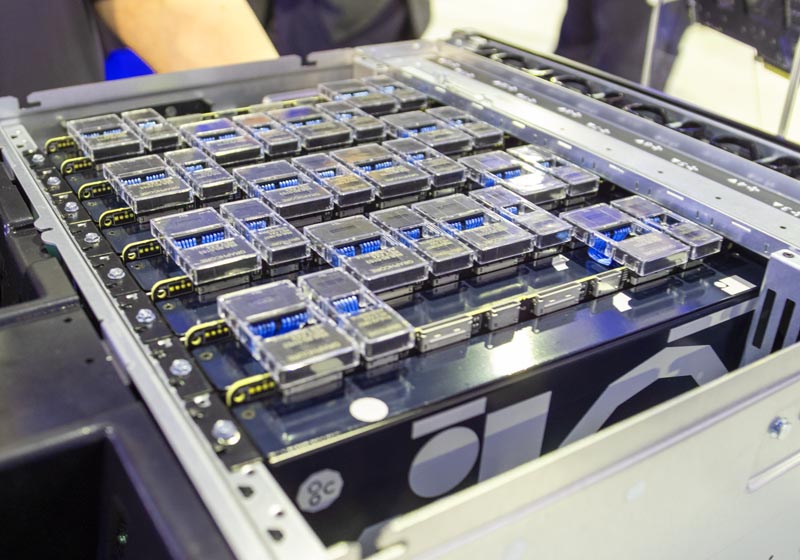
Alibaba is showing on-prem servers. The NVIDIA A100 Alibaba offering is paired with the Intel Xeon Platinum 8269CY which should be a 26-core chip with a 2.5GHz or so base clock.
Inspur has an ultra interesting result. The result lists the “NF5488” server but did not have the generational tag. As an example, we recently reviewed and did a video on the Inspur NF5488M5 Review A Unique 8x NVIDIA Tesla V100 Server. That is an Intel Xeon solution, yet the result here is an AMD EPYC solution with the new NVIDIA A100. Inspur has traditionally been an Intel and POWER CPU vendor, but never AMD. As the last major server OEM to not have an AMD EPYC platform, this could be the first official sign that that is changing.
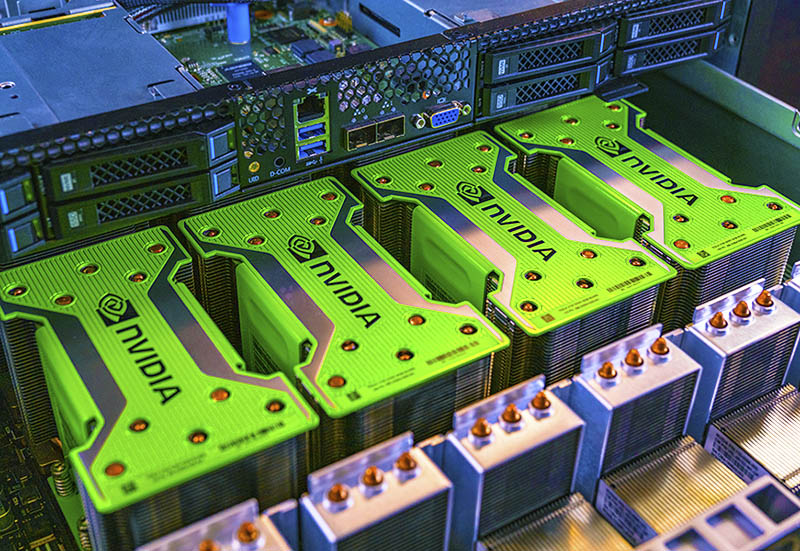
The rest of the results are all NVIDIA DGX systems. Of the 76 configurations across all categories submitted, 40 are NVIDIA DGX systems. This is the first chance we get to see the NVIDIA A100 v V100 so that makes it interesting. The results were not exactly the same in terms of frameworks and quantities, so we distilled the result sets into the most directly comparable configurations:
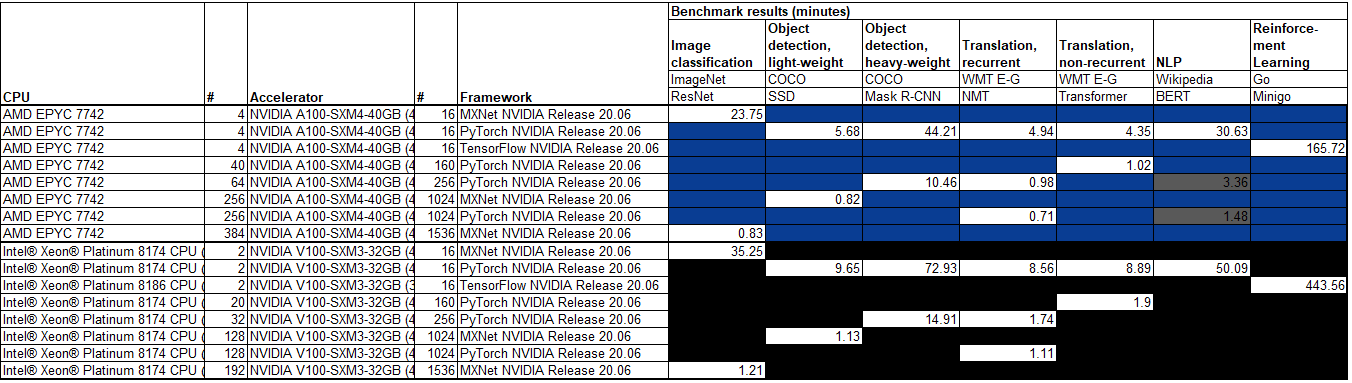
Those are still great numbers for the NVIDIA A100.
MLPerf 0.7 Open Division Results
If NVIDIA dominated the closed division, Huawei did the same for the open division with four of the five results.

Seeing the Huawei Ascend 910 on here is great. Still, we only see the ImageNet ResNet results being submitted, no others. The Fujitsu result is perhaps showing their speedup on the same hardware as it had in the Closed On-Prem division.
MLPerf 0.7 High-Level Results Discussion
55 of 76 configurations use NVIDIA GPUs. That is over 76% of the total population using one vendor’s products. Next, we have Google with 8 configurations, Intel with 7 configurations, and Huawei with 6 configurations. All of the Huawei results were only on the ImageNet ResNet test and none of the others offered by MLPerf.
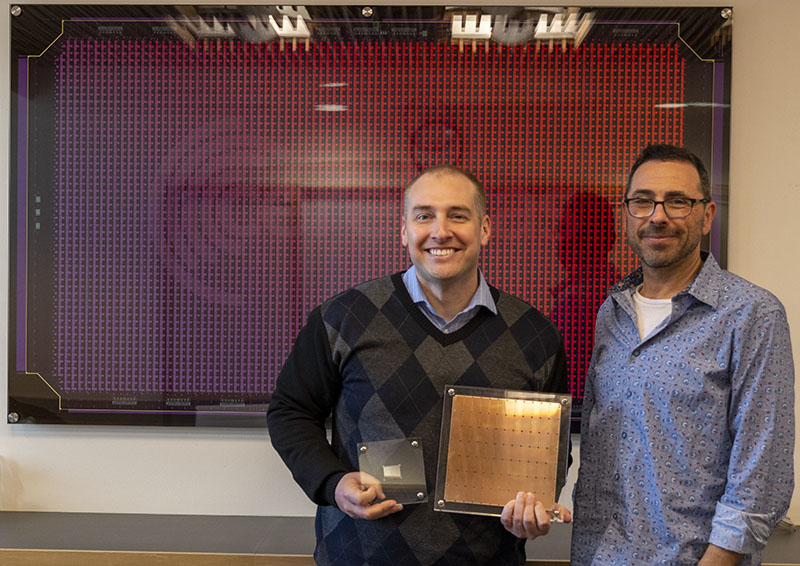
This begs the question, where is everyone else? In our interview with Andrew Feldman CEO of Cerebras Systems we asked months ago about MLPerf and he said his company is focusing on customer trials. Likewise, we just saw the Graphcore GC200 IPU Launch and it is not on this list. Even Intel, a staunch supporter of MLPerf, did not put the Habana Labs chips on this list. We know that the Habana Labs product was good enough that Facebook effectively had Intel cancel its Nervana line and purchased Habana Labs for it so Facebook and Intel think it has merit in training. Yet it is not here.
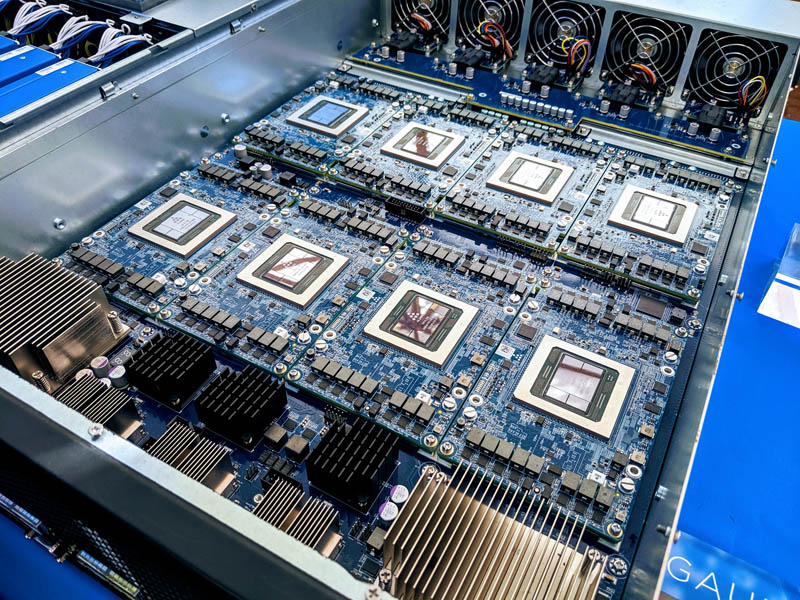
When we go beyond configurations, there were 138 results submitted for this edition of MLPerf. 46 of those results were non-NVIDIA so perhaps that is a bit better. Still, for a start-up, what is the upside of being on MLPerf at this point? A startup needs a large number of accelerators to be dedicated to this effort and has to weigh that versus taking the demo systems from sales. In that, sales efforts will win almost every time. Even if an accelerator company submits a result with a lower number of accelerators, they are going to be a small data point compared to the sea of NVIDIA results along with any other large player.
Perhaps the biggest takeaways from this MLPerf 0.7 Training list are:
- Google is making TPU V4
- Huawei Ascend 910 exists in quantity, even if it is only running a single benchmark here
- The NVIDIA A100 is a substantial jump over the V100
- Intel can do training using bfloat16, albeit not with any great haste on these benchmarks
The biggest message by far was the NVIDIA one, and for that, why does NVIDIA need to submit to MLPerf if it is mostly just their chips. It highlights that software is hard, and NVIDIA has invested heavily on the software side, but at this point, NVIDIA’s results are like being “the fastest” in a race of one where you are also a race organizer.
Final Words
Overall, MLPerf’s vision is one that we need in the industry. I have given feedback that there needs to be a smaller version to make it more accessible to other organizations that have accelerators but do not want to, or cannot, dedicate the huge amount of expense to compete with NVIDIA on a benchmark versus customer demos. Also, we need additional data such as power consumption for any on-prem solution since that is the only way one has a chance of coming up with a TCO versus the cloud options.
All this said, we have a great list to go, but there is still much work to be done.
Check out the full results on MLPerf.org.
MLPerf has a number of very talented people behind it, but I’ve been fairly disappointed by it so far. Perhaps I let my hopes get in the way of reality, but it seems to be turning into a trophy case for marketing rather than offering useful and comparable ML metrics.
As it stands now — and as mentioned in the article — it’s looking pretty inbred. Any future articles providing color around why Cerebras, Habana and other quieter architectures aren’t putting themselves out there would be appreciated. The number of Intel AVX-512 systems in inference is similarly bewildering. Will Nuvia, for instance, MLPerf their offerings? I don’t know, but I don’t have my hopes up.
MLPerf doesn’t force submitters to commit to running all tests, or at least provide notes on whether tests/frameworks are unsupported or on-roadmap. Presumably they’re cherry picked. What’s the point of digging through the table if the systems I want don’t offer the test I want?
Here’s what I wanted from MLPerf: A competitive, inclusive benchmarking comparison across a range of frameworks. I want a simple way to see where architectures shine and where they fall flat. No one architecture is going to do it all, but I was hoping MLPerf would show me — in the most comparable way possible — where a given architecture objectively shines and where it objectively struggles against all the major and minor players out there. It’s still early days and I’m hoping it achieves that in the future.
I have to agree. I don’t find what is being published useful. They’re optimizing the snot outta these benchmarks and then only posting the best. That’s like SPEC CPU but with SPEC its usually the server sellers that do benchmarks and there are 4 numbers that count in rfps on a per system basis peak and base and int and fp. It’s like they’ve spent all this time money and energy to produce these results but they’re not actionable.
STH needs to review more. Maybe that’s how this changes. You’ve got the platform, size, and readers to do it for 1 accelerator to 2 nodes and you’ve got an audience. I know you do some but you could do more.