Lenovo ThinkStation P920 Tower Deep Learning benchmarks
While many workstations are designed for CPU acceleration or engineering and media creation tasks, a new and popular field is deep learning. Also, more professional applications are using AI inferencing to augment classical mathematical models. We wanted to see how this system performs.
ResNet-50 Inferencing in TensorRT using Tensor Cores.
ImageNet is an image classification database launched in 2007 designed for use in visual object recognition research. Organized by the WordNet hierarchy, hundreds of image examples represent each node (or category of specific nouns).
In our benchmarks for Inferencing, a ResNet50 Model trained in Caffe will be run using the command line as follows.
nvidia-docker run --shm-size=1g --ipc=host --ulimit memlock=-1 --ulimit stack=67108864 --rm -v ~/Downloads/models/:/models -w /opt/tensorrt/bin nvcr.io/nvidia/tensorrt:18.11-py3 giexec --deploy=/models/ResNet-50-deploy.prototxt --model=/models/ResNet-50-model.caffemodel --output=prob --batch=16 --iterations=500 --fp16
Options are:
–deploy: Path to the Caffe deploy (.prototxt) file used for training the model
–model: Path to the model (.caffemodel)
–output: Output blob name
–batch: Batch size to use for inferencing
–iterations: The number of iterations to run
–int8: Use INT8 precision
–fp16: Use FP16 precision (for Volta or Turing GPUs), no specification will equal FP32
We can change the batch size to 16, 32, 64, 128 and precision to INT8, FP16, and FP32.
The results are in inference latency (in seconds.) If we take the batch size / Latency, that will equal the Throughput (images/sec) which we plot on our charts.
We also found that this benchmark does not use two GPU’s; it only runs on a single GPU. You can, however, run different instances on each GPU using commands like.
```NV_GPUS=0 nvidia-docker run ... &
NV_GPUS=1 nvidia-docker run ... &```
With these commands, a user can scale workloads across many GPU’s. Our graphs show combined totals.
We start with Turing’s new INT8 mode which is one of the benefits of using the NVIDIA RTX cards.
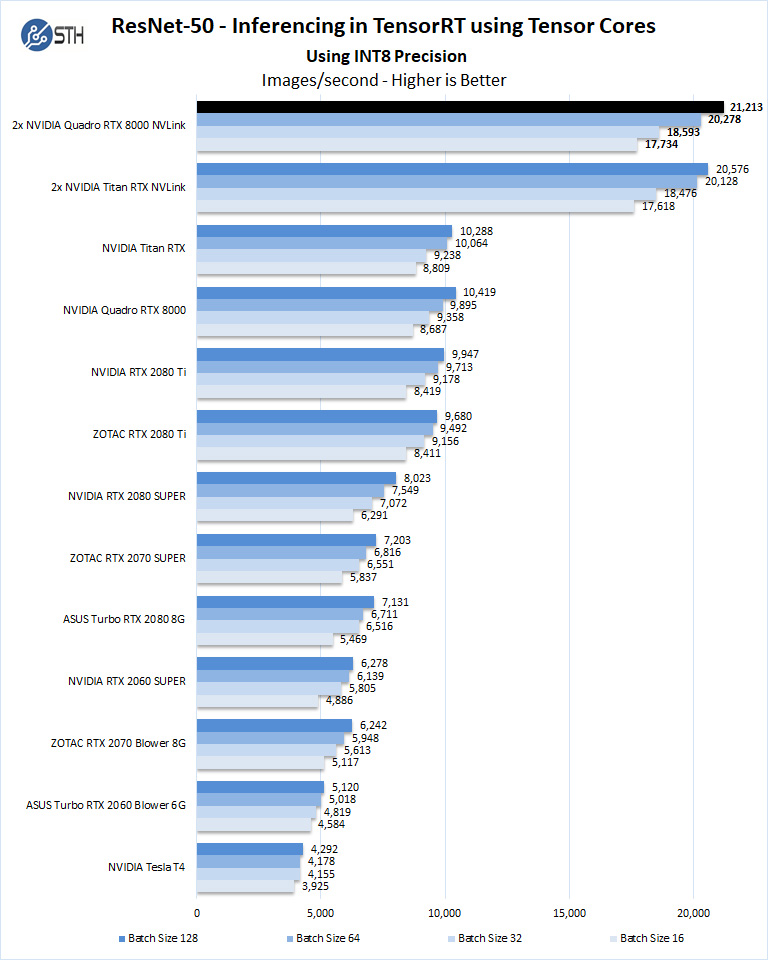
Using the precision of INT8 is by far the fastest inferencing method if at all possible converting code to INT8 will yield faster runs. Installed memory has one of the most significant impacts on these benchmarks. Inferencing on NVIDIA RTX graphics cards does not tax the GPU’s to a great deal, however additional memory allows for larger batch sizes. The NVIDIA Quadro RTX 8000 could easily do batch sizes of 500+.
Let us look at FP16 and FP32 results.
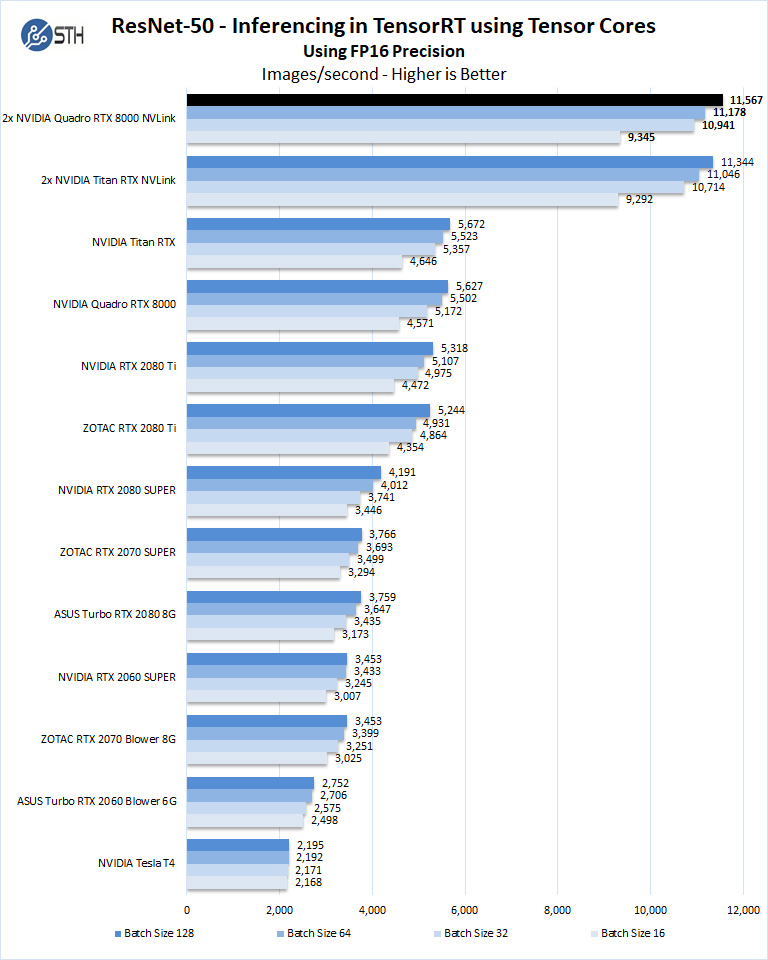
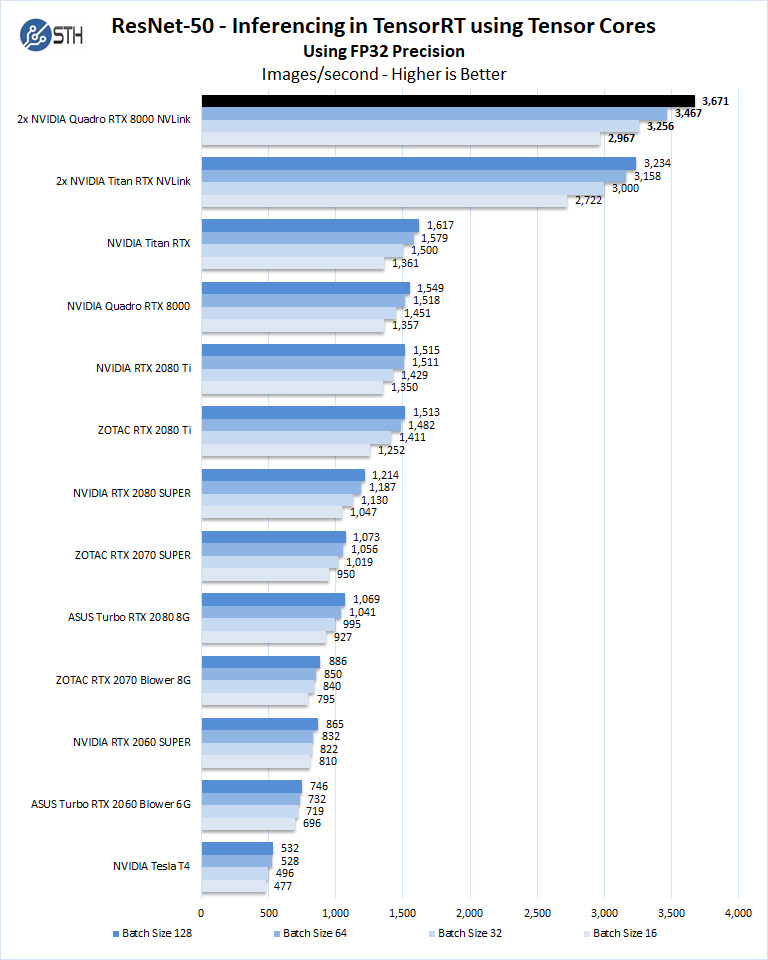
As you can see we get a big boost using the Quadro RTX 8000 cards here.
Training with ResNet-50 using Tensorflow
We also wanted to train the venerable ResNet-50 using Tensorflow. During training the neural network is learning features of images, (e.g. objects, animals, etc.) and determining what features are important. Periodically (every 1000 iterations), the neural network will test itself against the test set to determine training loss, which affects the accuracy of training the network. Accuracy can be increased through repetition (or running a higher number of epochs.)
The command line we will use is:
nvidia-docker run --shm-size=1g --ipc=host --ulimit memlock=-1 --ulimit stack=67108864 -v ~/Downloads/imagenet12tf:/imagenet --rm -w /workspace/nvidia-examples/cnn/ nvcr.io/nvidia/tensorflow:18.11-py3 python resnet.py --data_dir=/imagenet --layers=50 --batch_size=128 --iter_unit=batch --num_iter=500 --display_every=20 --precision=fp16
Parameters for resnet.py:
–layers: The number of neural network layers to use, i.e. 50.
–batch_size or -b: The number of ImageNet sample images to use for training the network per iteration. Increasing the batch size will typically increase training performance.
–iter_unit or -u: Specify whether to run batches or epochs.
–num_iter or -i: The number of batches or iterations to run, i.e. 500.
–display_every: How frequently training performance will be displayed, i.e. every 20 batches.
–precision: Specify FP32 or FP16 precision, which also enables TensorCore math for Volta and Turing GPUs.
While this script TensorFlow cannot specify individual GPUs to use, they can be specified by
setting export CUDA_VISIBLE_DEVICES= separated by commas (i.e. 0,1,2,3) within the Docker container workspace.
We will run batch sizes of 16, 32, 64, 128, and change from FP16 to FP32. Our graphs show combined totals.
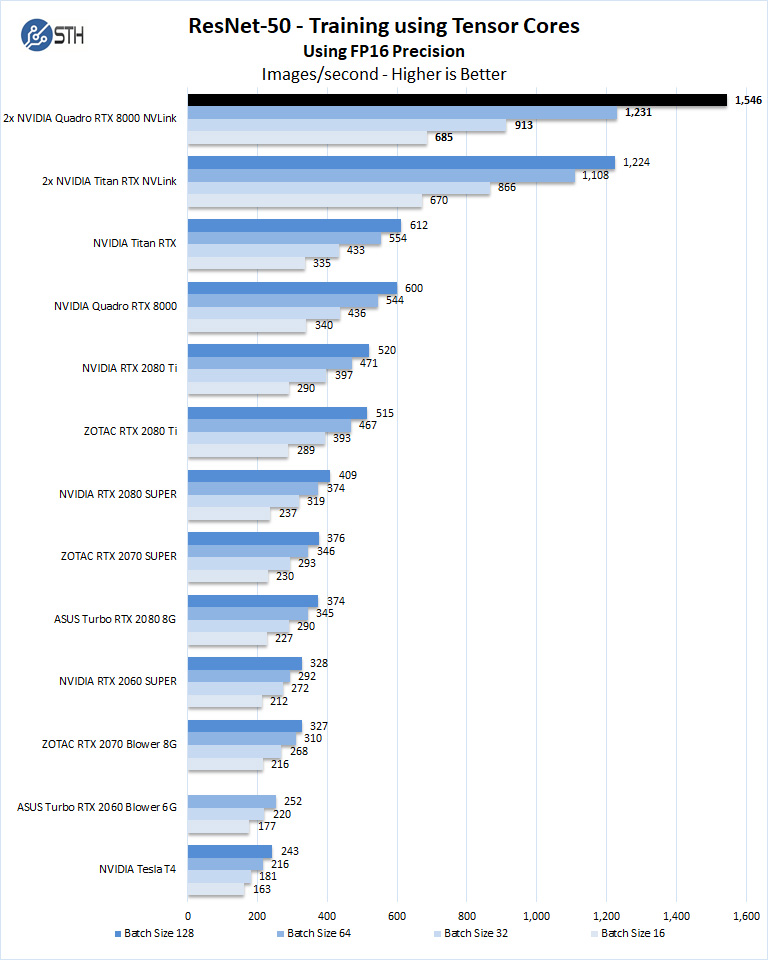
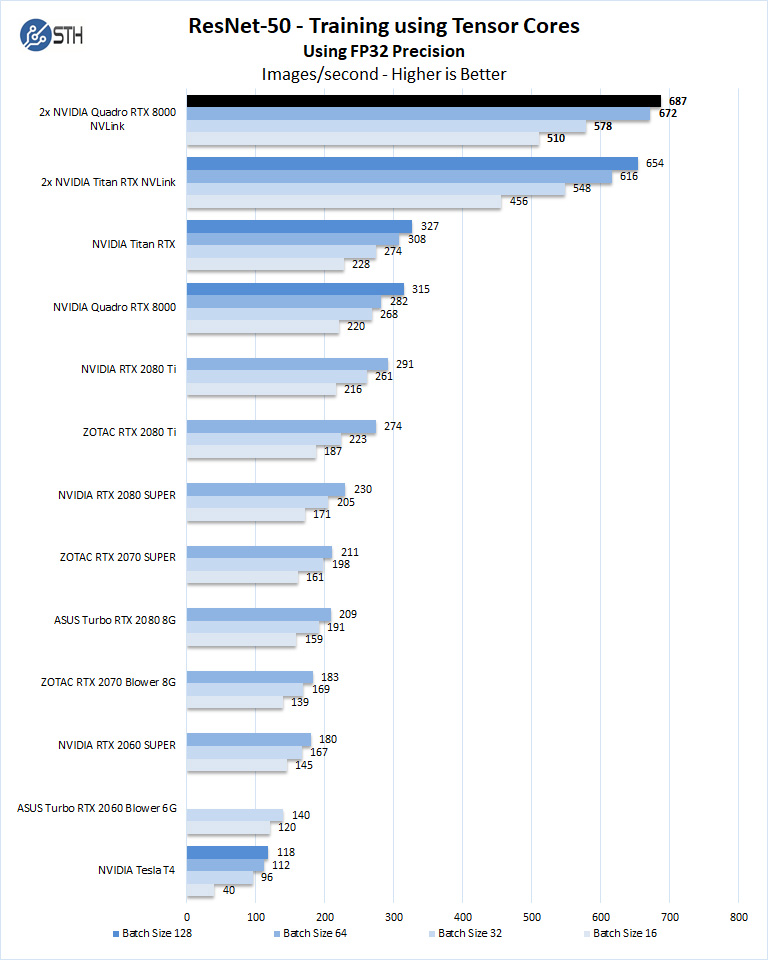
ResNet-50 Training is greatly improved when using NVLINK configurations. The two Quadro RTX 8000’s in NVLINK match Titan RTX NVLINK but can go far deeper in batch sizes with the expanded memory the RTX 8000’s offer.
Deep Learning Training Using OpenSeq2Seq (GNMT)
While Resnet-50 is a Convolutional Neural Network (CNN) that is typically used for image classification, Recurrent Neural Networks (RNN) such as Google Neural Machine Translation (GNMT) are used for applications such as real-time language translations.
The command line we use for OpenSeq2Seq (GNMT) is as follows.
nvidia-docker run -it --shm-size=1g --ipc=host --ulimit memlock=-1 --ulimit stack=67108864 -v ~/Downloads/OpenSeq2Seq/wmt16_de_en:/opt/tensorflow/nvidia-examples/OpenSeq2Seq/wmt16_de_en -w /workspace/nvidia-examples/OpenSeq2Seq/ nvcr.io/nvidia/tensorflow:18.11-py3
We then open the en_de_gnmt-like-4GPUs.py and edit our variables.
vi example_configs/text2text/en-de/en-de-gnmt-like-4GPUs.py
First, edit data_root to point to the below path:
data_root = "/opt/tensorflow/nvidia-examples/OpenSeq2Seq/wmt16_de_en/"
Additionally, edit the num_gpus, max_steps, and batch_size_per_gpu parameters under
base_prams to set the number of GPUs, run a lower number of steps (i.e. 500) for
benchmarking, and also to set the batch size:
base_params = {
...
"num_gpus": 1,
"max_steps": 500,
"batch_size_per_gpu": 128,
...
},
We also edit lines 44 and below as shown to enable FP16 precision:
#"dtype": tf.float32, # to enable mixed precision, comment this
line and uncomment two below lines
"dtype": "mixed",
"loss_scaling": "Backoff",
We then run the benchmarks as follows.
python run.py --config_file example_configs/text2text/en-de/en-de-gnmt-like-4GPUs.py --mode train
The results will be Avg. Objects per second trained which we plot.
We should note that other GPU’s we used to like the RTX2060, RTX2070, RTX2080, and RTX2080 Ti could not complete this benchmark due to the lack of memory. To enable this benchmark to finish on these GPU’s one might need to lower the batch size to smaller values like 32, 16, 8. We tried this but had no luck, using a batch size 4 could be run but it was decided that this was not a very usable size.
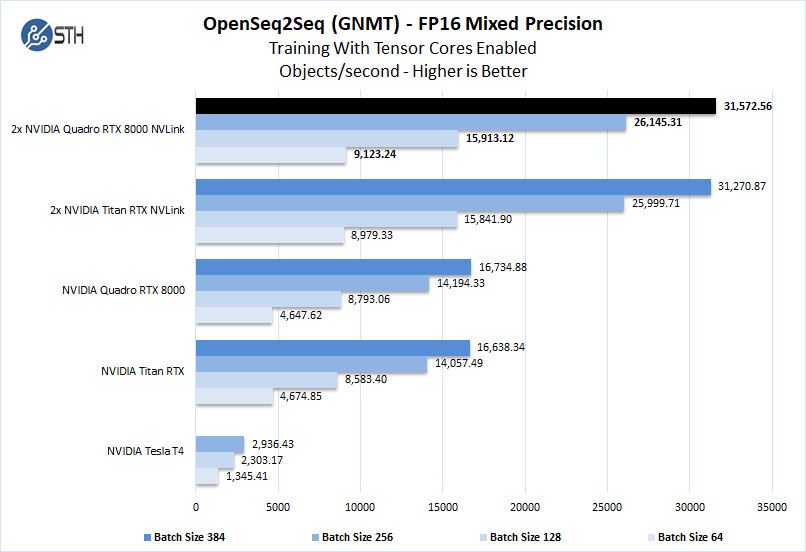
As the NVIDIA Quadro RTX 8000 has 48GB of installed memory, double that of the Titan RTX. The Quadro RTX 8000 is easily equal to the Titan RTX but offers larger batch sizes on a single GPU.
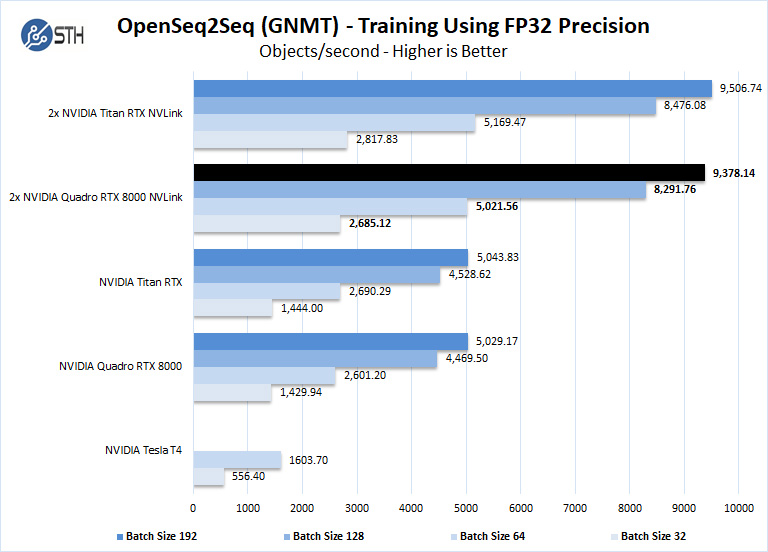
With OpenSeq2Seq (GNMT) Training users have limited choice in GPU’s to select from as it requires large amounts of installed memory. We find the Quadro RTX 8000 at the top end with 48GB of installed memory. Using two of the Quadro RTX 8000’s gives one a massive 96GB of memory to work with, enabling very deep batch sizes possible.
Of course, you may use different networks that will perform differently, but we wanted to give some sense of the inferencing and training performance of a machine like this.
Next, we are going to look at the AIDA64 CPU Benchmarks.
P620 looks to be the better, cheaper, faster option with a drawback that’s it’s not available at the moment.
Looks to be a swan’s song of xeon/lenovo workstation especially after p620 was already announced. The only question is if p620 will be half or one third of the p920 price.
Anyway, pity AMD is saving this for OEMs since if it would be in general market, man would be able to build even cheaper WS.
So sorry, why is the P620 not rubbing shoulders with the P920?
40k !!! No way
Everybody blaming Apple for their pricey workstations.
But, simply put, horsepower is expensive.
When you go for professional workstation the order of magnitude is well above 25-30k.
Apple, although more expensive seems not too far from this price tag.
(writing this from my lenovo notebook which is great :-) )
Their airflow design is rather poor though, second cpu heats up quite a lot more and higher than seems reasonable, because of that the rear fan goes bonkers and it is Very loud, now imagine an office space with 30 of these
Wonder if Intel actually paid or otherwise leveraged Lenovo somehow to segment the Threadripper in the 600 series of their Workstation range. After all, to the clueless exec that is going to be signing off on these, 920 is exactly 300 more than 620, and the morer the betterer, like always.
P620 is about 5x more desirable in all real world scenarios I can think of.
Unfortunately, this doesn’t matter at all. These workstations sung their songs, and it’s game over for them anyway (willing to bet they will bleed a lot of market share in the next years). Everybody I know in my industry (Turbomachinery, heavy ANSYS users) say they got switched to mobile workstations in the last couple of years (myself included), and pointed towards local HPC cluster, or worse yet – AWS, for heavy lifting.
You can imagine with COVID and WFH trends, it’s just going to become even worse for the big ol’ boxes.
Too bad. I really like them. And no, working on a cluster can never feel* as fast, snappy and productive as working on your local beast.
*subjectively, OFC
@Turbo
That and when media outlets use “Truly Top End” Workstation as their tagline and then a muted “first” for the competitor, it really highlights the focus on one to the other.
It seems such a cold shoulder when the only reasons to not put the 620 up higher on the list are 600GB less memory and per core licensing models…
“Lenovo ThinkStation P920 Review A Truly Top-End Workstation”
A truly top-end in price, compared with P620?
Hi William,
this is pendantry, and not at all necessary for comprehension, but may I be allowed to suggest a easier scan for your punctuation of your introductory paragraph?
Thus:
“Lenovo has updated its classic ThinkStation P910 Tower we reviewed three years ago. With the latest processors and GPU’s equipped in our P920, let us jump into it and see how these new machines perform and all of the features Lenovo includes in its ThinkStation P920.”
Potentially might be more easily read, after the following very minor editing:
“Lenovo has updated its classic ThinkStation P910 Tower, which we reviewed three years ago, with the latest processors and GPU’s equipped in our P920.”
“Let’s jump into it and see how these new machines perform, and all of the features Lenovo includes in its ThinkStation P920.”
Mischa,
The last time I wrote a PO for IBM Xeon w/s we ended up getting the machines subsystem by subsystem stowed in carry on from Armonk because our tiny order languished in someone’s in tray depths for a few months unti we realised that IBM was still under a consent order to not offer for sale anything not available from stock for delivery. The legacy of the Seven Dwarves slain by vaporware deposit taking for frames still on the drawing board expired iirc in ’05 after 50 years.
I’m recalling this because – STH notices aside – the general market is increasingly disenfranchised and segregated from the state of the art in ways that I am increasingly certain are highly prejudicial to our scale competitiveness. The pecking order doesn’t even seem to confer any embarrassment upon the industry and I think this is a all round bad sign because it cools the most fervid ardor for high margin early adoption purchasing as well as the equinanimus and sanguine mind when evaluating computing strategy.
Ivan,
I really think that Apple is criticised for the empty ecosystem for components, AICs, just generally and I specifically here am uncaring for the Apple – nvidia situation which is fire, because the few people who need desktop graphics horsepower ime equip with acceleration such as the RED raw card and the high end has lots of dedicated outboard for eg TRANSCIDING, color timing, HDR conforms, and with many softwares like Flame the w/s is a package deal with little budgetary impact and you’re handing over the work flow from OS to OS via SAN or now nvme fabric only noticing the application processes. Only users without any established output pipeline into tier one distribution and single handed shops are troubled by the paucity of options. Unfortunately that’s 95% of the industry by headcount. Just wander around even stills photography and the sheer volume of Mac tweaking guides (some prominent ones very expensive drivel) is revealing that desperation.