A few weeks ago at CES, we covered Kioxia AiSAQ. This is software that looks to replace memory in the RAG AI stack with SSDs to allow larger models at a lower cost. The other goal is minimizing the impact of using the lower cost per TB device on performance. Now, that software is open source.
Kioxia AiSAQ SSD-backed RAG for Larger Scale AI Models Goes Open Source
For folks who are new to RAG, or retrieval augmented generation, the idea is simply that a LLM can access data sources to provide context that did not exist in the training set. For example, if you have a model, and a set of constantly refreshing business data. That tends to take a lot of memory and storage. Kioxia’s AiSAQ is that storing the vector data and index in storage, rather than a giant memory pool, can cost a lot less.
As we discussed in the last piece, accessing the information happens using an approximate nearest neighbor operation or ANN that is often done on CPUs. Microsoft has its DiskANN that moves some of the index and vector data out od DRAM, but keeps product quantization vectors in DRAM. With Kioxia AiSAQ, the idea is to move all of that data onto SSDs.
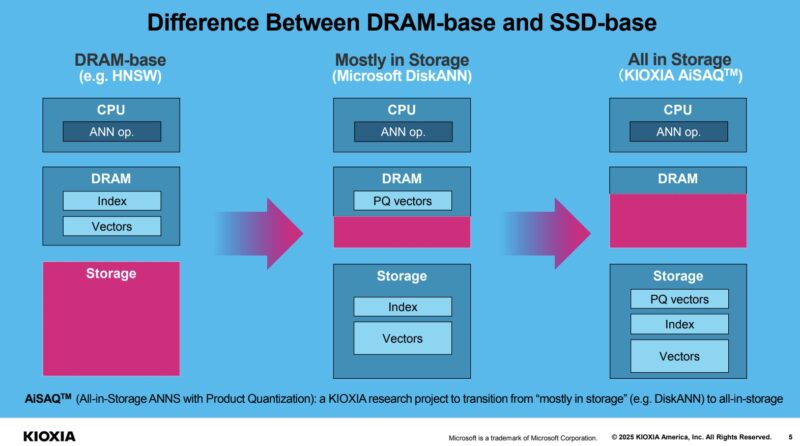
Using flash instead of DRAM decreses costs, especially at the TB scale. So Kioxia is saying that it can store enormous vectors with minimal DRAM footprint by moving everything to SSDs.
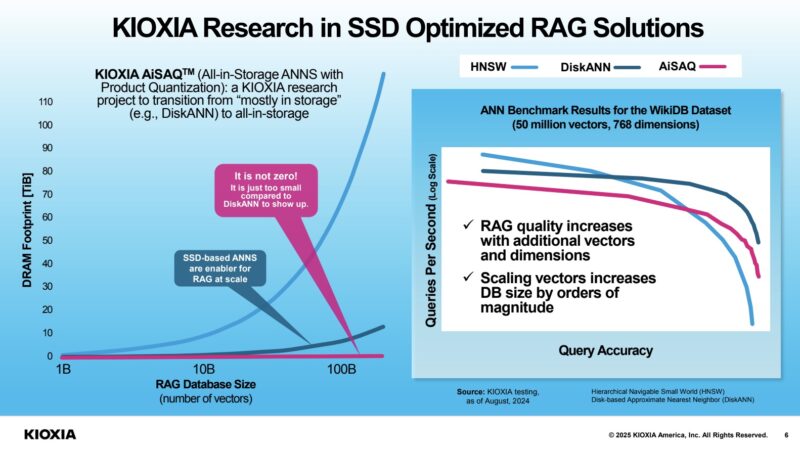
A disadvantage of this is that it can be slower than using all DRAM but the benefit is that it can cost less to hit a given scale. Higher scale can mean higher quality or more accurate results.
Final Words
This is a new tool that folks may want to use. Now, that AiSAQ has been open sourced and is on Github, it is availabe to use. Maybe this is something that folks find useful. Maybe it is not. At least it is out there.
If you want to try it out, you can find it on Github here.
I wonder how this compares to Phison’s aiDAPTIV+.
They’re actually pretty different. AiSAQ is all about speeding up RAG performance for inference, making things run faster and more efficiently. Meanwhile, aiDAPTIV+ lets you fine-tune large language models without needing a massive GPU cluster, solving the usual VRAM limitations that come with training.