Yesterday we focused on the Intel Xeon Sapphire Rapids Support of features such as HBM. Today we have another update. Intel is officially resetting expectations for the next-generation Sapphire Rapids Xeon family.
Intel Sapphire Rapids Xeon Expectations Reset to Q2 2022
Prior to today’s announcement, Intel had been guiding for a Q4 2021/ Q1 2022 timeframe. With today’s announcement, it is expecting that it will have some initial production in Q1 2022, but the volume ramp will occur in Q2. If we compare this to the Ice Lake Xeon series, this is similar to the wording used for those products and realistically we saw significantly more chip availability in May 2021 than we did at the early April launch.
This is a push that we have expected to happen for some time. Once Ice Lake was pushed to Q2 2021, the immediate question emerged as to whether some customers would wait for Sapphire Rapids which was slated for less than a year in the future. If we go back to the summer of 2020, and our The 2021 Intel Ice Pickle How 2021 Will be Crunch Time piece, we had the 2017 to 2023 roadmap drawn and one can see basically a two-quarter slip which makes sense.
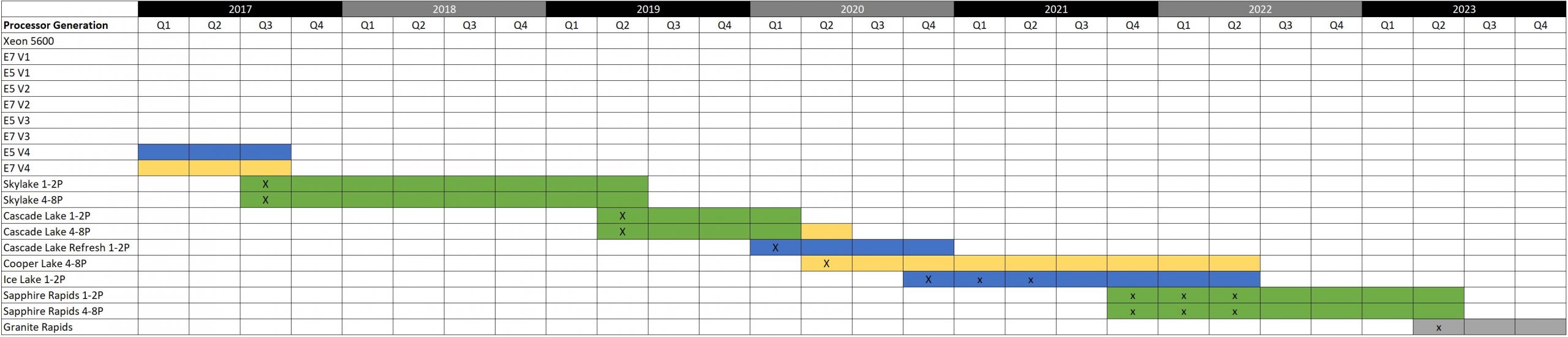
Here, one can see that the transition from Ice Lake to Sapphire Rapids was set to happen very quickly. While the target dates were pushed, it looks like Intel is trying to maintain at least four quarters of spacing between generational launches.
Final Words
Overall, it is great that Intel is at least getting out ahead of this one. The Ice to Sapphire timeline compression was one of those points that most in the industry have known Sapphire would slip, but there was no official confirmation. That led to a case where Intel’s public roadmap would make customers look at the timeline between the current-gen of pre-CXL parts and the CXL/ PCIe Gen5/ DDR5 future generation only a few months away and take pause considering delay options. With this new guidance, both customers and OEMs have a bit more time to do validation and get a better launch organized. OEMs also get a bit more time with Ice Lake platforms in the market before the option of Sppahire Rapids becomes available.
We just want to say this is a great direction by Intel starting to get further ahead of the news cycle. The industry is enormous and there are too many folks involved to keep secrets long.
Once again you are way too apolegetic when it comes to Intel:
1. Intel definiteley knew months ago how bad their 10 nm is. Instead of being honest like a big company should be they acted like a startup that’s hoping for a sudden breakthrough and waited until they couldn’t keep silent.
2. They are still not honest about Optane. Probably just using all the wafers from micron inventory and then saying goodbye.
3. Still no details about their new “simplified” 7 nm. No update on transistor density? Will it even be competitive with TSMC N5?
4. Intel is not honest about AMX, VNNI, and partially AVX-512. They made a mistake years ago by trying to do everything through additional instructions but by now they know that dedicated accelarator units on CPUs or even dedicated cards are much more effective and performant. It’s obvious that Intel is approaching dedicated AI inference and training (on- and off-chip) but they are still fooling customers and developers to believe that there is a future in these instruction set extensions.
Im gonna have to defend AVX-512 for a bit.
1. It is nor “just wider vectors”, it is a lot more than that. It has new ISNs that have no narrower counterpart.
2. It was supposed to arrive to the desktop and mobile segments ages ago, with Cannon Lake. But 10 nm shit the bed so hard and for so long that it nuked AVX-512 adoption.
3. Leaks suggest AMD is adopting it with Zen 4, or at least parts of it. Possibly by cracking it into 256 wide uops like zen 1 did with 256 wide isns.
I agree, VNNI and AMX is kinda useless in their current form, but I am not the target market.
Now if they could extend AMX to support non-useless data types, like FP32/64, the it would actually get interesting, particularly on HBM equipped CPUs.
@Koss
1. I put “partially” before AVX-512 bc it’s partially OK ;)
2. It’s not just an adoption problem: If you have so much data that you need to process in SIMD manner where AVX-512 has a big advantage vs AVX-2 you should do that on the GPU. Example: The Xe-LP GPU on Tiger lake with up to 768 FP32 multiplicators is a really nice “coprocessor” for most tasks (like adobe suite). It’s faster for FP32 multiplication than 8 CPU cores with 2 AVX512 units each. There is still the exception of some instructions missing (look at 1.) and no FP64 but I would rather see Intel extend the instructions of it’s GPUs than the CPUs. Especially since CPU and GPU can work at the same time on different tasks but filling all your AVX512 pipelines will make your CPU almost useless until the work is done.
3. Just supporting it by being compatible is OK but they shouldn’t waste too many resources/too much die area on that.
“Now if they could extend AMX to support non-useless data types, like FP32/64, the it would actually get interesting, particularly on HBM equipped CPUs.”
That would take much more die area, especially the register files and caches. Instead of doing that they are doing FP32/64 matrix multiplication on their(or NVIDIAs) new GPUs that are dedicated to those tasks. Those who need it buy an accelerator. Those who don’t, don’t pay premium for giant CPU dies (with bad Intel yield).
The AMX processing supports int8 operations for ai inference and bfloat16 operations for training. Both of these data types are efficient and useful for those applications.
In Lisa Spelman’s announcement she stated about AMX … “achieving over two times the deep learning inference and training performance compared with our current Xeon Scalable generation. “.
Appears to me there’s currently a lot of time spent copying buffers back and forth for GPU execution, so whether or not avx512 is more efficient has some threshold.
@JayN
Yes it may be twice as “good” as current Xeon Scalable but the competition is not CPUs, it’s dedicated accelerators like Nvidia A100/T4, AWS inferentia, TPU v3/v4 and even Intel’s own Habana Gaudi/Goya and the newer Intel Xe-HP/HPC.
The reason why Intel is investing billions in accelerators but taking a half-hearted approach with AMX:
Accelerators will always be more efficient and have better price/perf than the AMX approach.
+There is no datacenter use case where you do a little AI training/inference on the CPU and then do other stuff like transaction processing on the same machine.
You always use dedicated machines for training/inference and that’s why there is no downside to using dedicated accelerators..
Color me shocked, this will be the first of several slips.
“You always use dedicated machines for training/inference and that’s why ,,,”
The current state is that there is a big overhead moving buffers back and forth between CPU and Accelerators. In AI processing models the operations are very compartmentalized, and each can be evaluated for whether they will execute more efficiently on an accelerator or on a host/master cpu. The avx512 simd can be very efficient if it supports 8 bit FMA for the convolution filters used extensively in inference.
The use of cxl will reduce somewhat the overhead of using an accelerator, but there will still be a threshold overhead for the transfers. The amx processing on SPR moves the threshold back in favor of the CPU. The increased memory bandwidth from HBM stacks in the SPR will also move the threshold back in favor of CPU.
Intel’s techdecoded site has a training session, “Profiling Heterogeneous Computing Performance with Intel® VTune™ Profiler” that explores their support for evaluating time spent in execution of kernels … how much is in transfer vs execution.
There is also a simpler tool, documented in Intel Advisor Cookbook, if you just want the decision whether to run your kernel on CPU or offload to GPU.
@JayN, “The use of cxl will reduce somewhat the overhead of using an accelerator, but there will still be a threshold overhead for the transfers.”
From my reading of CXL on STH, it virtually negates the overhead.
As always there are two camps, each sees nothing of what the other does. The Big Boys will always want dedicated accelerators. The Rest will see the cost advantage of just CPU as “good enough for me”. Edge use will favor CPU. Datacenter will need DPU.