Inspur NF5488M5 Performance Testing
We wanted to show off a few views on what makes this different than other GPU compute servers we have tested.
Inspur NF5488M5 CPU Performance to Baseline
In the original draft of this piece, we had a deep-dive into CPU performance. Since we already have more in-depth CPU reviews, and CPU performance is not the focus of this system.
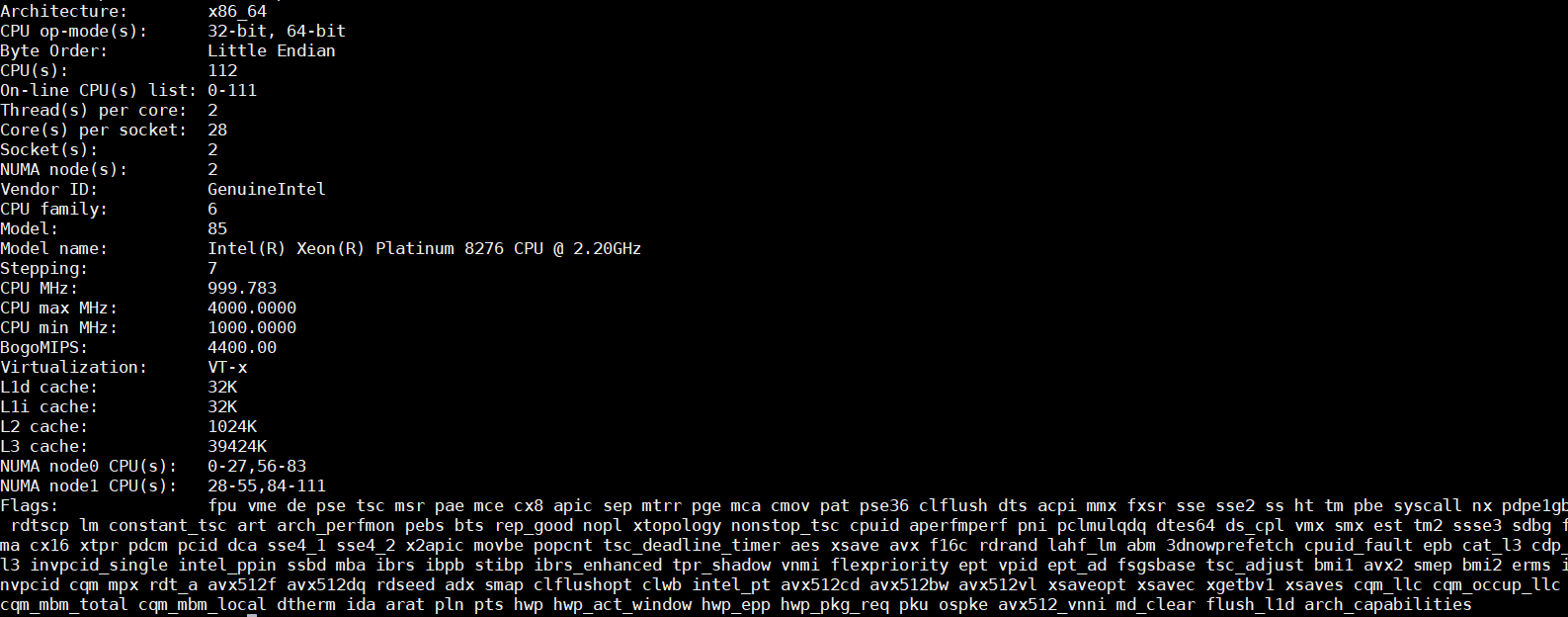
We instead are just going to present our baseline Platinum 8276 performance versus the same performance in the Inspur NF5488M5.
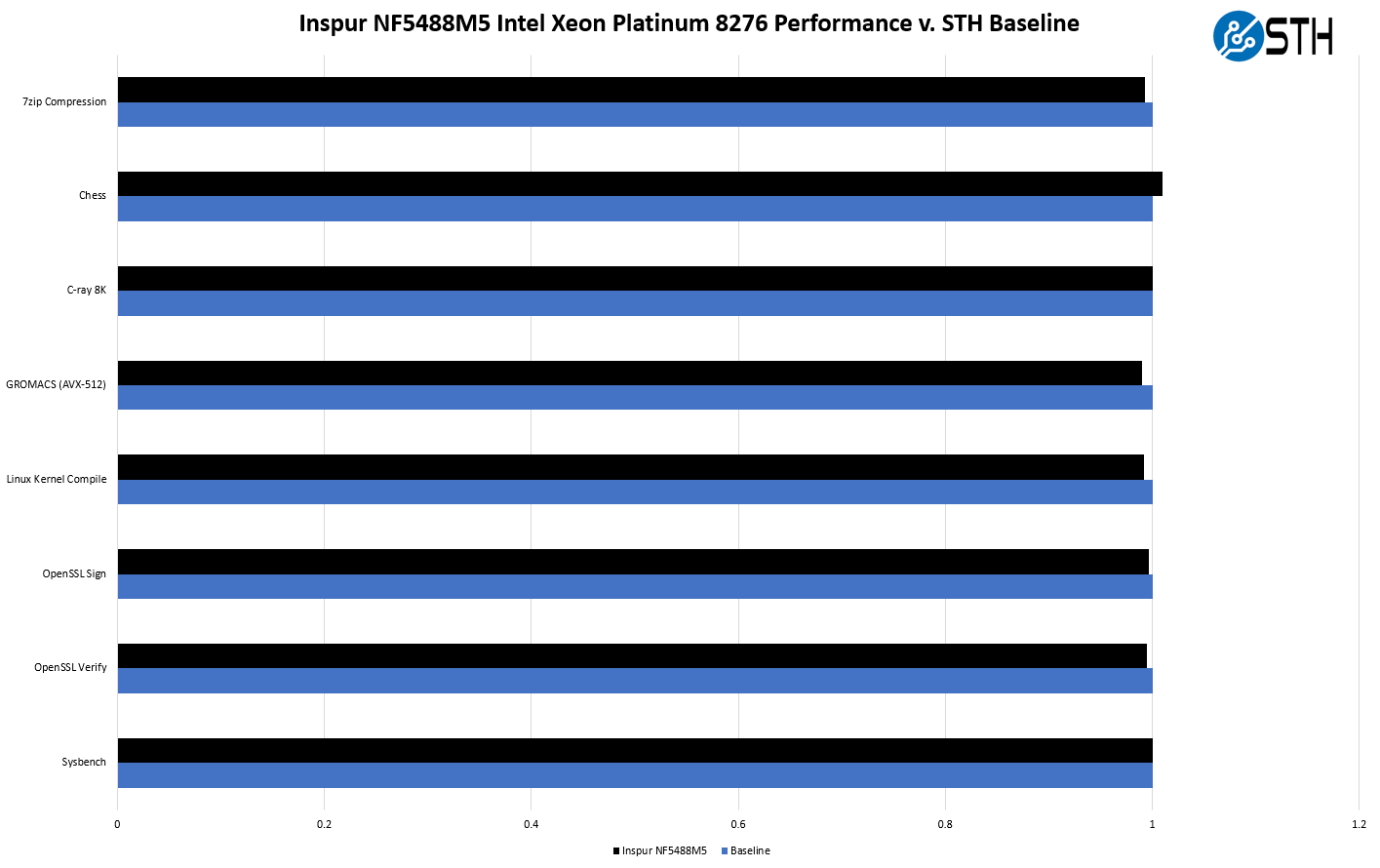
As you can see, we generally stayed very close to our primary testbed which shows we are getting adequate cooling to the CPUs.
Inspur NF5488M5 P2P Testing
We wanted to take a look at what the peer-to-peer bandwidth looks like. For comparison, we have DeepLearning10, a dual root Xeon E5 server, and DeepLearning11 a single root Xeon E5 server, and DeepLearning12 a Tesla P100 SXM2 server. If you want to compare some of these numbers to an 8x Tesla V100 32GB PCIe server, you can check out our Inspur Systems NF5468M5 review.
Inspur NF5488M5 P2P Bandwidth
Here is the Unidirectional P2P bandwidth on the dual root PCIe server:
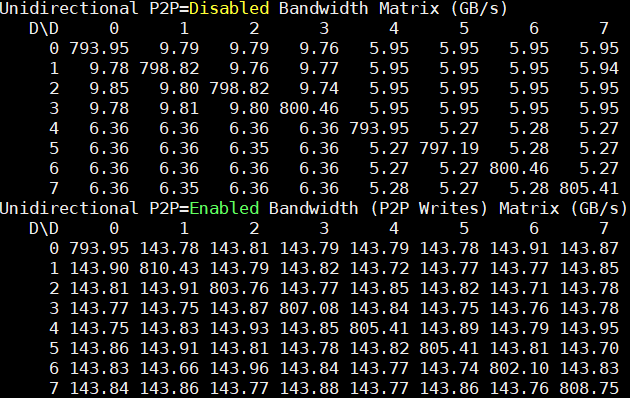
Here we can see the unidirectional P2P bandwidth is 143GB/s that was about 9-18GB/s on the PCIe dual root server with the Tesla V100’s. Also, that is more consistent across the GPUs whereas the PCIe server had a lot of variation depending on the placement of the GPUs.
Looking at bidirectional bandwidth:
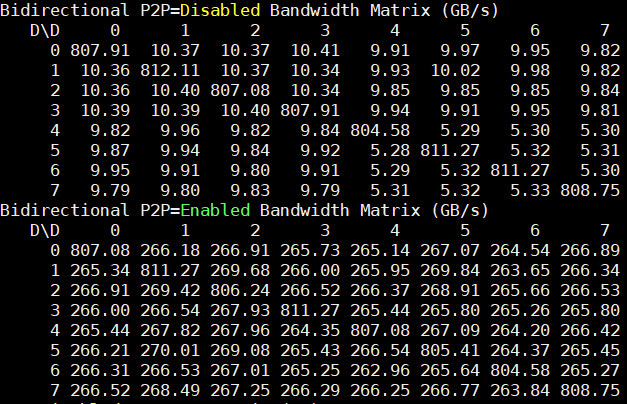
We again see 266GB/s bandwidth between GPUs and very consistent results. We compare this from about 18-37GB/s on a PCIe Gen3 switched server. You can also see the 800GB/s figures here for the same GPU (e.g. 0,0) which was closer to 400GB/s on the Tesla P100 SXM2 generation.
Just for good measure, we also had the CUDA bandwidth test:
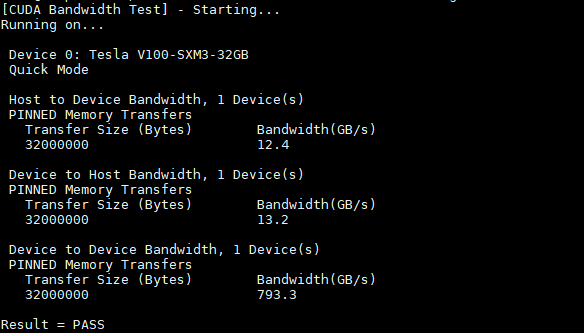
We wanted to show here that the on-device bandwidth is phenomenal around 800GB/s as you can see in these P2P numbers.
Inspur NF5488M5 P2P Latency
Beyond raw bandwidth, we wanted to show Inspur Systems NF5488M5 GPU-to-GPU latency. Again, see links above for comparison points:
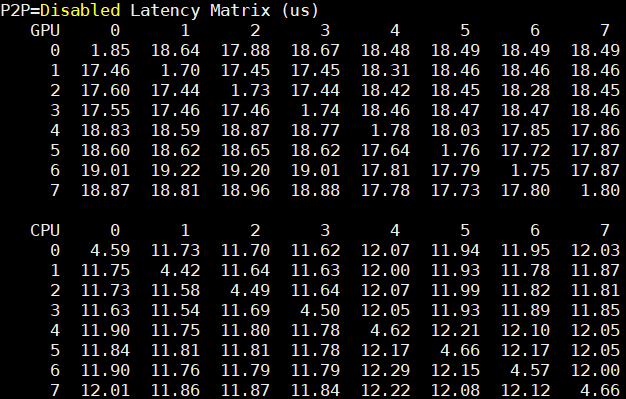
Here are the P2P enabled latency figures:
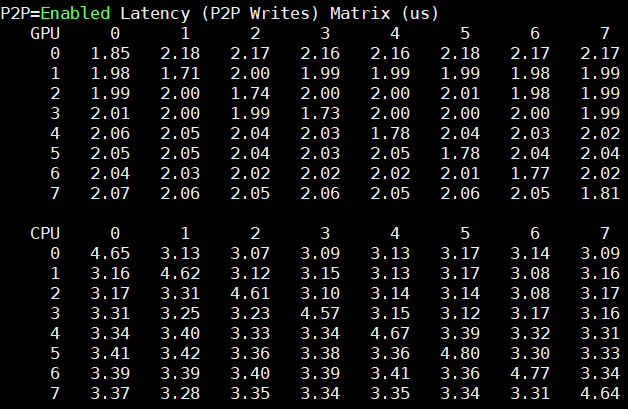
These figures are again very low and consistent.
While this is not intended to be an exact performance measurement, it is a tool you can quickly use on your deep learning servers to see how they compare.
Raw Deep Learning/ AI Performance Increase over PCIe
We had data from the Inspur Systems NF5468M5 that we reviewed and so we ran some of the same containers on this system to see if we indeed saw a direct speedup in performance.
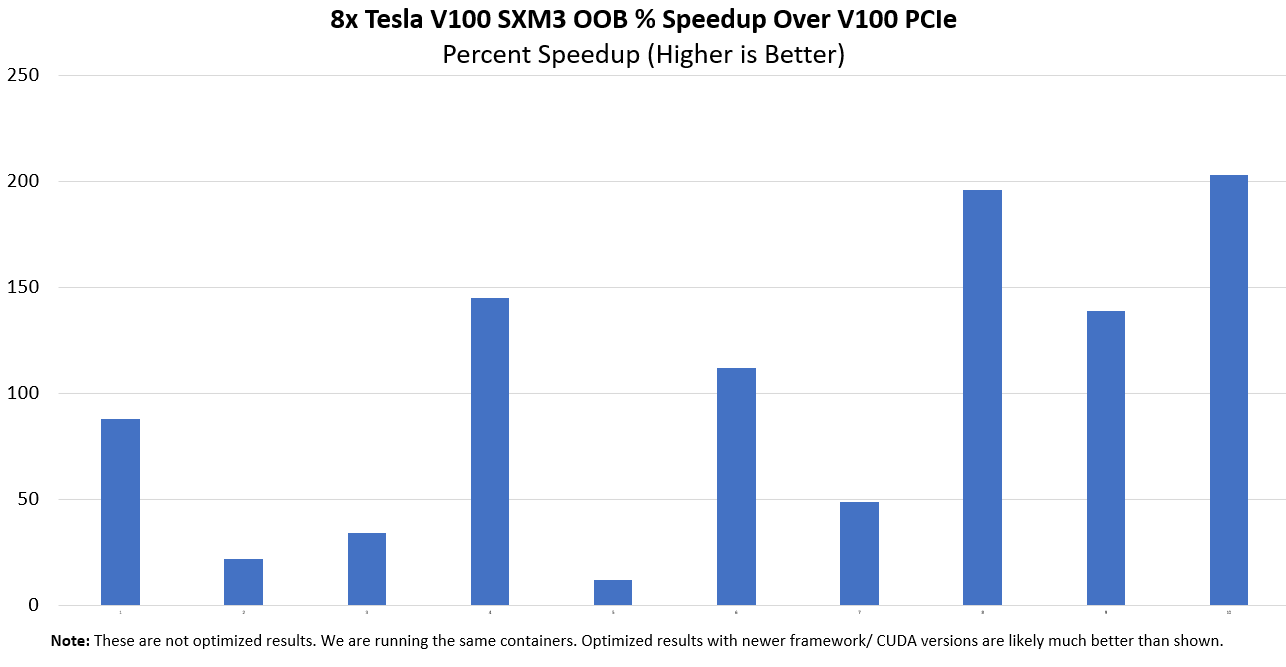
Of course, the usual disclaimers here are that these are not highly optimized results, so you are seeing more of a real-world out-of-box speedup across a few companies that we help test their workloads on different machines as part of DemoEval. Realistically, if one uses newer frameworks, and optimizes for the system, better results are obtainable. The above chart took almost two weeks to generate, so we did not get to iterate on optimizations since we had a single system and a limited time running it.
This is one where we are just going to say, our testing confirmed what one would expect, faster GPUs and faster interconnects yield better performance, the degree of which depends on the application.
That’s a nice (and I bet really expensive) server for AI workloads!
The idle consumption, as shown in the nvidia-smi terminal, of the V100s is a bit higher than what I’d have expected. It seems weird that the cards stay at the p0 power state (max freq.). In my experience (which is not with v100s, to be fair), just about half a minute after setting the persistence mode to on, the power state reaches p8 and the consumption is way lower (~10W). It may very well be the default power management for these cards, IDK. I don’t think that’s a concern for any purchaser of that server, though, since I don’t think they will keep it idling for just a second…
Thank you for the great review Patrick! Is there any chance that you’d at some point be able to test some non-standard AI accelerators such as Groq’s tensor streaming processor, Habana’s Gaudi etc. in the same fashion?
What’re the advantages (if any) of this Inspur server vs Supermicro 9029GP-TNVRT (which is expandable to 16GPU and even then cost under 250K$ fully configured – and price is <150K$ with 8 V100 32GB SXM3 GPUs, RAM, NVMe etc)?
While usually 4U is much better than 10U I don't think it's really important in this case.
Igor – different companies supporting so you would next look to the software and services portfolio beyond the box itself. You are right that this would be 8 GPU in 4U while you are discussing 8 GPU in 10U for the Supermicro half-configured box. Inspur’s alternative to the Supermicro 9029GP-TNVRT is the 16x GPU AGX-5 which fits in 8U if you wanted 16x GPUs in a HGX-2 platform in a denser configuration.
L.P. – hopefully, that will start late this year.