Graphcore v. NVIDIA HGX A100 Power Consumption
At this point, one may assume that perhaps the Graphcore IPU-POD16 is significantly better in terms of TCO because of lower power consumption. We looked up the specs, and that seems not to be the case.
The Graphcore IPU-POD16 is effectively a server plus four of the company’s IPU-M2000 units.
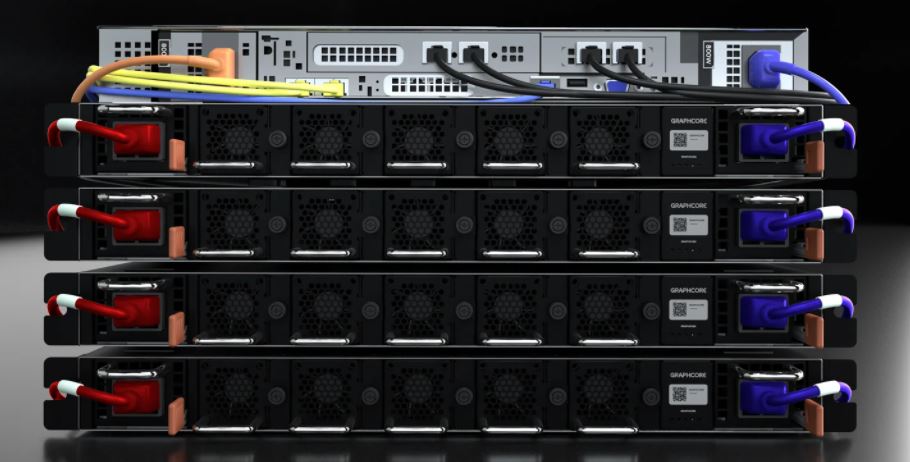
Here is an IPU-M2000 unit that makes up the four 1U chassis above:
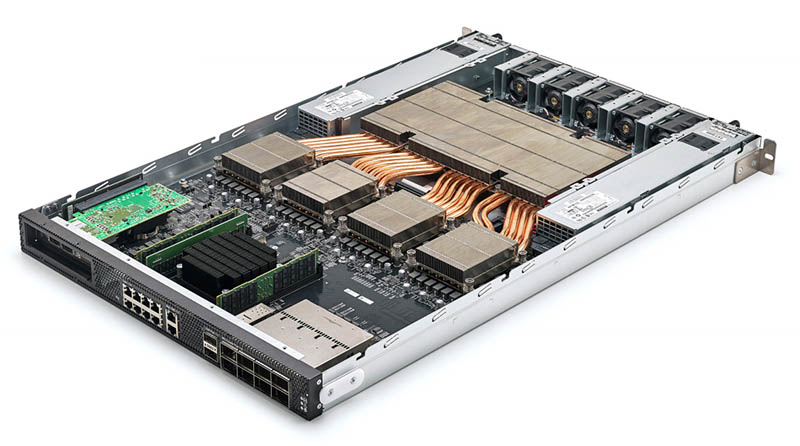
Each of the four IPU-M2000 systems has four GC200 Mk2 IPUs (not to be confused with Intel’s confusingly similar IPU usage as its rebellion to DPUs.) While we could not find power consumption for the IPU-POD16, we could for the IPU-M2000.
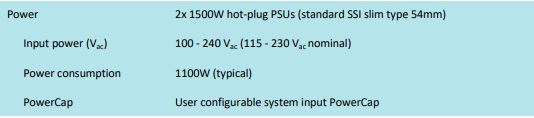
Typical power is rated at 1.1kW. There are four of the IPU-M2000 units in an IPU-POD16 that Graphcore is using for MLPerf Training v1.0. That gives us 4.4kW typical power usage.
Above the four IPU-M2000’s is a 1U server. One of Graphcore’s big VC backers is Dell (those VCs may not be feeling too good about their investment after this piece, sorry.) The idea is that a reseller/ channel partner can use their own server here. One can also scale IPU-M2000 and compute servers asymmetrically, but the IPU-POD16 is what was submitted to MLPerf Training v1.0. Whether using higher-end Intel Xeon Ice Lake or AMD EPYC 7003 Milan servers, a current-generation 1U dual-socket server will use in the 800W – 1kW range these days. There are options for other lower-power configurations, but that range holds regardless of vendor given the CPU power, system component power, and cooling requirements. We test these servers all the time, and that is a very reasonable range with top-end CPUs, 16x DDR4-3200 DIMMs, and so forth.
That gives us 4.4kW for the Graphcore units and 0.8-1kW for the server which is 5.2kW to 5.4kW combined.
For comparison, we did a quick Linpack run on an HGX A100 320GB (8x 40GB) 400W GPU system with AMD EPYC CPUs and this is what we saw for power:
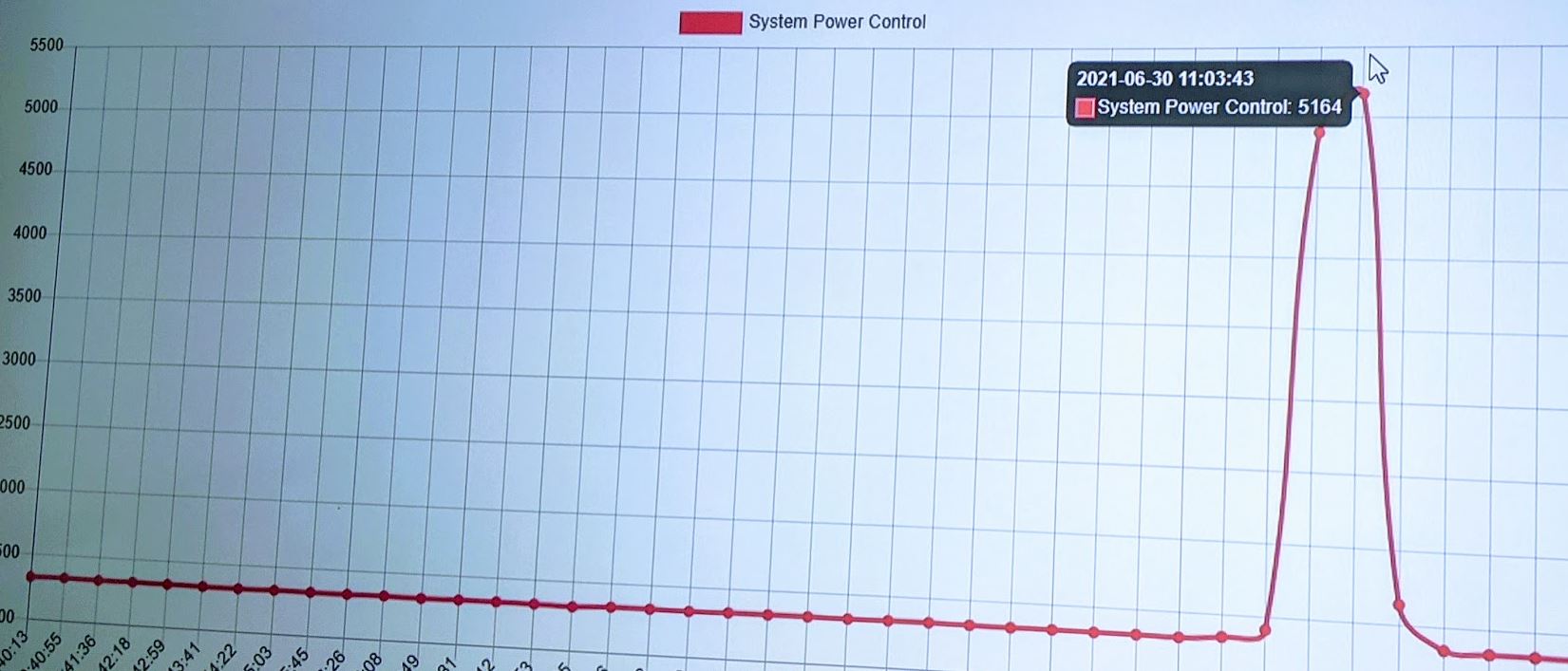
More on that system soon, but you can see that we hit 5.164kW when we ran this about an hour after the MLPerf results were announced. We did the 500W A100 system at the same time, and the power consumption is higher, but we are not considering that solution a direct competitor. This is not the Inspur NF5488A5 that was in MLPerf Training v1.0 and we will review next week, but the Inspur system is also very similar in terms of this 5.1kW to 5.3kW range. Since we have now tested both, this range seems directionally accurate for HGX A100 8 GPU 400W systems.
Comparing actual measured NVIDIA HGX A100 400W system power consumption with Linpack to the Graphcore “Typical” power consumption figures plus what we measure for 1U/2U dual-socket high-end servers is not perfect since we are not independently measuring the Graphcore figures. Power consumption also varies based on workload so this is far from a perfect representation. Still, it feels roughly similar.
We are going to quickly note that OEM systems with the HGX A100 8 GPU are typically 4U so Graphcore’s 5U against the DGX A100 6U is not a great comparison either. For example, we can see the Tesla Supercomputer with NVIDIA A100 80GB and Supermicro 4U servers shown clearly. Luckily, most racks run out of power and cooling before space with these systems so density is not as much of a factor in TCO for large portions of the market.
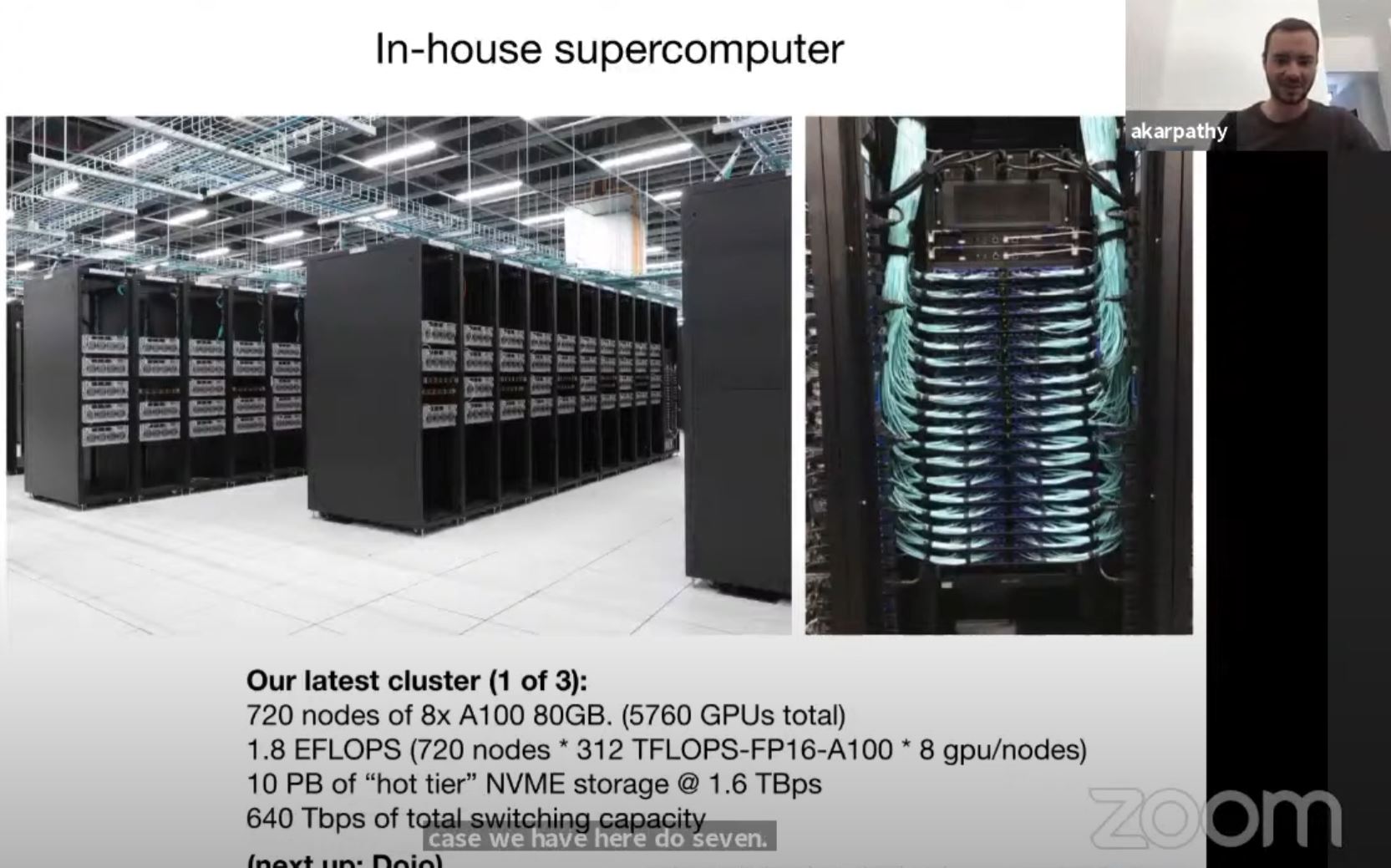
Still, at the end of the day, given the data we have, it seems like the Graphcore IPU-POD16 solution is close enough in power consumption to a NVIDIA HGX A100 system that they are roughly equivalent to the point where power is not the deciding factor. If we saw one at 10kW and the other at 5kW, we would be tempted to claim a major TCO impact. With this class of systems a few hundred watts most in the market will gladly burn to get more performance, better software support, or other benefits since it is small on a percentage basis.
Final Words
Personally, I found the Graphcore MLPerf Training v1.0 submission to be borderline heartbreaking. I have wanted to see a legitimate challenger to NVIDIA to increase competition in the industry and frankly hoped that Graphcore would be it.
Instead, Graphcore showed that it has an IPU-POD16 solution that is roughly equivalent to the NVIDIA HGX A100-based servers in terms of list price and power consumption. Graphcore’s performance, given the price/ power context, was significantly worse than NVIDIA’s. Even allowing for customization by comparing an “Open” result to the standard “Closed” result, it was far behind in terms of performance.
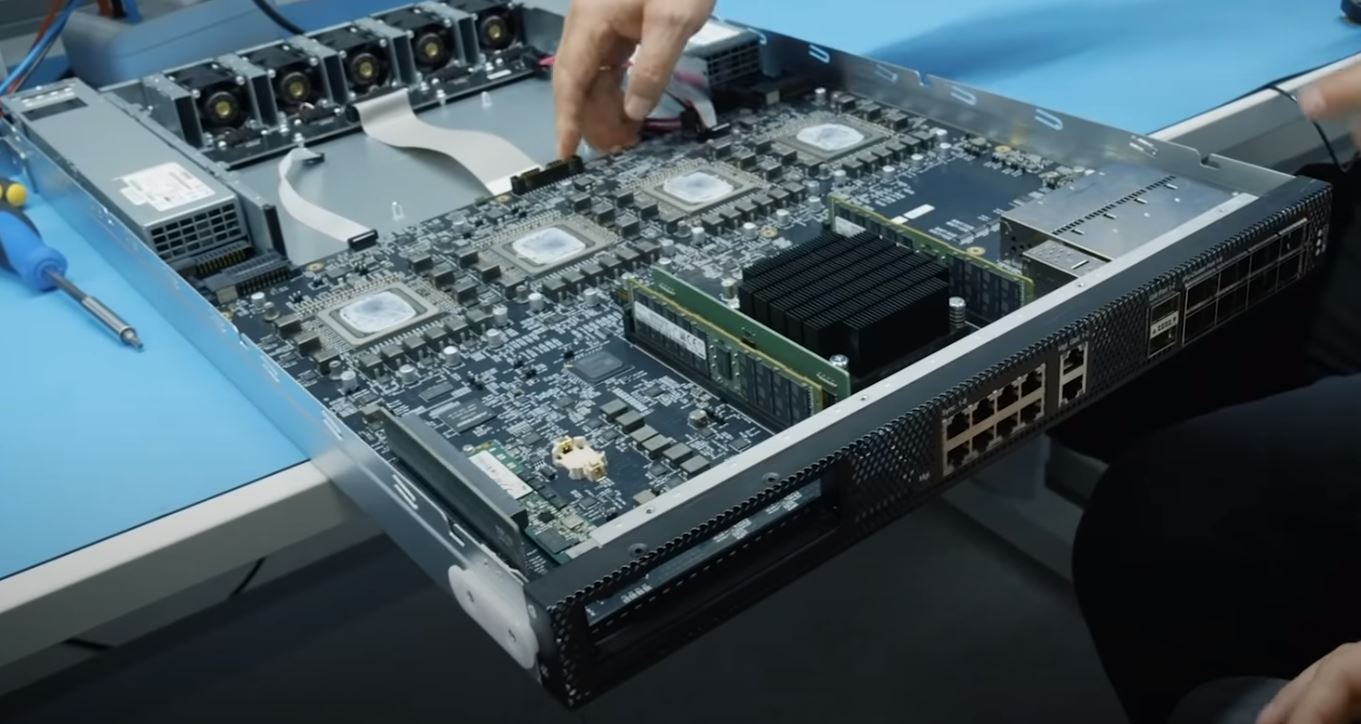
Let us get frank for a moment. NVIDIA has been leading the training market for generations and has an expansive software stack. A startup does not win by equalling NVIDIA in price/ performance, or even being 10-30% ahead. Certainly not by being behind. NVIDIA has a huge value proposition on the software and ecosystem side that is very tough for any startup to match. Intel is an example of a company that can spend and catch up, but the market is not hospitable to those entering without a great software story. Graphcore has been putting in the work, but NVIDIA is effectively the default for most of the industry at this point.
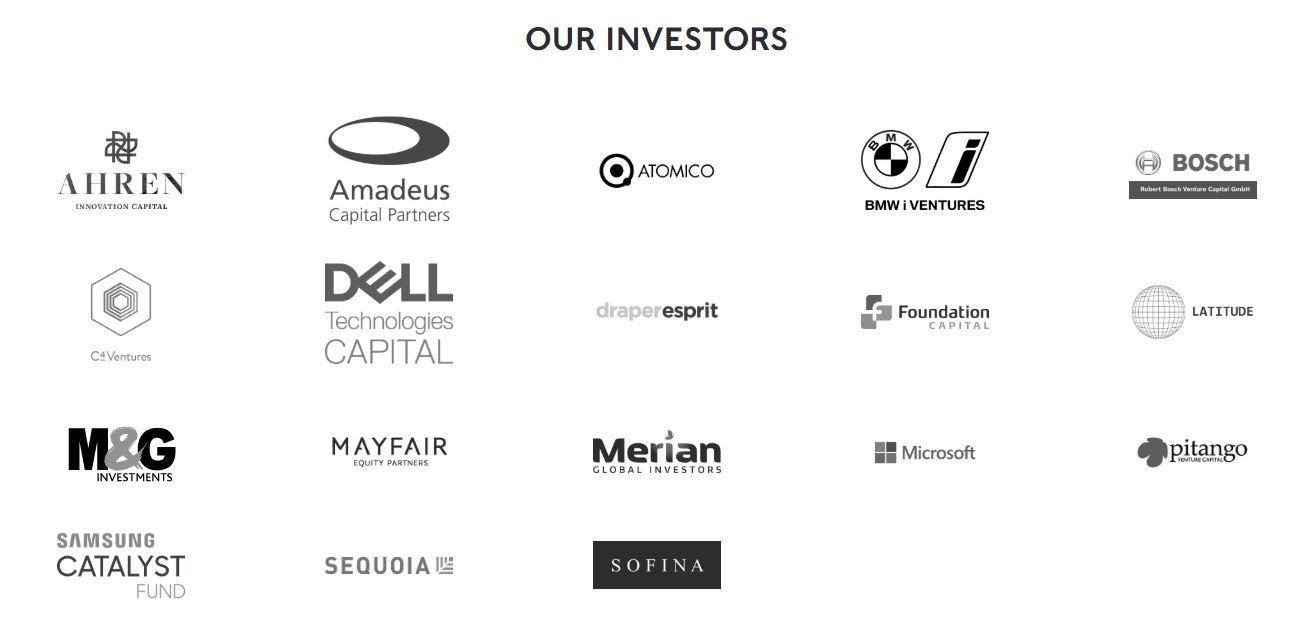
This was a spectacular failure, and it will damage the industry. Graphcore’s poor showing in MLPerf Training v1.0 (along with the Intel-Habana Labs submission) will and should dissuade other companies from submitting to MLPerf in the future. I asked in our interview with Andrew Feldman CEO of Cerebras Systems and his response was to focus on customers, not MLPerf. That is something Graphcore probably should have followed as well since even burning through huge sums of VC money has not gotten Graphcore close to NVIDIA in MLPerf.
Real-world customer results may differ, but if that is the case submitting MLPerf results was a poor choice. The outcome of MLPerf Training v1.0 results for Graphcore should have triggered a decision not to submit those results. Using fantasy pricing numbers instead of publicly available HGX A100 numbers online to take its slower performance and try spinning it into a price/ performance story was illogical. Many online may not understand NVIDIA’s go-to-market model since it has rarely been detailed as seen in this piece. Those who are in the AI hardware industry and are building competitive products should know how the largest player sells systems. Customers certainly know. Using what is effectively fantasy pricing to make its systems look better is strange since this is an industry of highly-educated folks that know what real pricing is.
If anything, I fear such a poor showing in MLPerf will dissuade customers from considering Graphcore in the future which will decrease competition in the industry. It should also prove to be a cautionary tale to other AI training chip startups.
This is why STH is great.
– Vendor “Yo we’ve got some BS to peddle.”
– STH “OK but here’s how it works and some actual data.”
You’ve been critical of NVIDIA before so it is good to see you’re being consistent.
We buy hundreds or thousands of GPU servers a year. You’re correct that we’re paying half of the $300K that Graphcore is using. Calling that “fantasy” is correct if you’re comparing real world price performance. We get a discount over what you’re using for the HGX A100’s but it isn’t that far off.
You could’ve stopped with just people don’t pay 300K for a system with 8 11-15k GPUs because that’d be stupid. Great analysis tho. Graphcore sells to AI shops that have smart engineers. I don’t see why they’ve used 300K since that’s not real. We also have A100 systems and they weren’t 300K.
You’re missing that the point of the article is not just about Graphcore.
It’s really a cautionary article for other AI startups looking to submit to MLPerf.
I’d only add that Google competes well in MLPerf but others shouldn’t even try.
I agree that comparing list price of high-end equipment is of little use because the procurement policy at most organizations for such expensive stuff involves a special contract negotiated by a purchasing department.
Thanks for including the energy analysis at the end of the article. From a technology point of view, performance per watt is much more interesting. It may also be more relevant in terms of total cost of ownership.
In the future it would be nice to measure total energy and time needed for each system to complete the same task for a number of different machine learning related activities.
You are right that Graphcore shouldn’t have participated in MLPerf.
My comment is you should make the PCIe-RedStone-Delta its own article so it is easy to reference. That and the DELTA label pic are golden.
Patrick, do you have any similar real-world pricing for the Graphcore solutions? NVIDIA is sold at 50% of MSRP but if Graphcore is similarly discounted then their comparison still holds.
ListVLower if you read, he’s saying the LIST of the HGX A100 is in the 150-180k range before discounts. This is a very educated view of pricing because all this is priced at the cluster level and it’s not priced at the node.
It’s a little silly to make the price comparison because any organization that is buying a specialized training computer is spending a multiple of what it costs on developers, “data scientists”, labeling and such.
The devs already know something about the NVIDIA ecosystem and won’t feel like they’re wasting time learning more. (e.g. as it is widely used in ML, image synthesis, etc.) NVIDIA also has some nice tools that would be hard for GraphCore to match. Dev(s) can even develop with laptop & desktop cards w/ NVIDIA but w/ Graphcore Dev(s) will have to fight for access to the server and put up with a slower training cycle so add it all up and they will be less productive and you’ll pay back whatever you saved on the machine — if you really saved on the machine.
In inference there is more the case new tech can be transformative, but not if we are talking a factor of 1.2x but more if there is a factor of 12x. (Incredible if memory bandwidth is anywhere near being the memory bottleneck)
An alternative takeaway is that this is the first time we get real numbers out of these startups, and they’re not good. “Focusing on customers” is not going to do much if your platform is not competitive.
I think saying that all these startups chose to not participate is being generous. I guarantee that everyone who has working hardware ran the suite. The absence of good results should be taken as a signal that they aren’t competitive, not that they didn’t bother trying.
—–
As always, great in-depth reporting!
The text under the “ML Perf training: ResNet-50” image states
“Graphcore highlights its 32.12 minutes to complete the benchmark while the NVIDIA DGX A100 takes 28.77 minutes which is the result 1.0-1059.”
The time shown in that image for Graphcore is 37.12, not 32.12
Thanks for the catch Grant!
There seems to be typos in:
“One major OEM had too think of thermal paste”
and
“Instead, and OEM purchases the NVIDIA Redstone platform and integrates it”
You might like to fix. Thanks :-)
This is one where STH shows why its #1 in server analysis. Usually the next platform does good stuff but they swung and missed on this one. The HGX A100 8 GPU bits, power, and the “cluster” view where the training systems are only part of the deployed solution are relevant and this is an insane level of depth.
Just know we appreciate it.
“We will have a review of the Inspur NF5488A5 next week, but the 1.0-1035 result is using the 500W variant so we should exclude it here. They have been offered by most OEMs for some time, but are not listed on NVIDIA’s current A100 data sheet which only lists 500W models.”
only lists.. 400W models?
@Chu Lim I’d bet Graphcore sponsors TNP.
Amazing stuff! For years i was getting the impression, that those gazillions of startups would be much more competetive than they now actually seem.
That NVIDIA is holding up so good with GPU derivied silicon against supposedly hyper-optimized silicion exclusively design for AI is stunning.
Just a naive view from a AI novice.
Again, really amazing article!
The site NextPlatform’s article aligned with GraphCore’s claims: https://www.nextplatform.com/2021/06/30/graphcore-right-on-the-money-in-first-mlperf-appearance/
U̶s̶u̶a̶l̶l̶y̶ almost always their articles are better than that.
@Moderator, I noticed that my comment was deleted; was there a problem with it?