The world of training big AI models is no longer the realm of a desktop or a few servers. Big AI research organizations are using thousands of accelerators to train huge models. Google, a major force in AI training, designs its own accelerators and systems to use both for internal purposes, as well as to rent via the Google Cloud platform. At Hot Chips 32 (2020) we had the chance to learn a bit more about the Google TPUv3 and the improvements over TPUv2 pods.
Google TPUv3 at Hot Chips 32
Starting with the basic building block, the chips under the cold plates in the cover image above, this is the basic block diagram for the TPUv3. One can see the two cores, their vector, scalar, matrix multiply, and transpose/ permute units. There is HBM memory as well. One can see PCIe connectivity to a host as well as a high-speed interconnect.
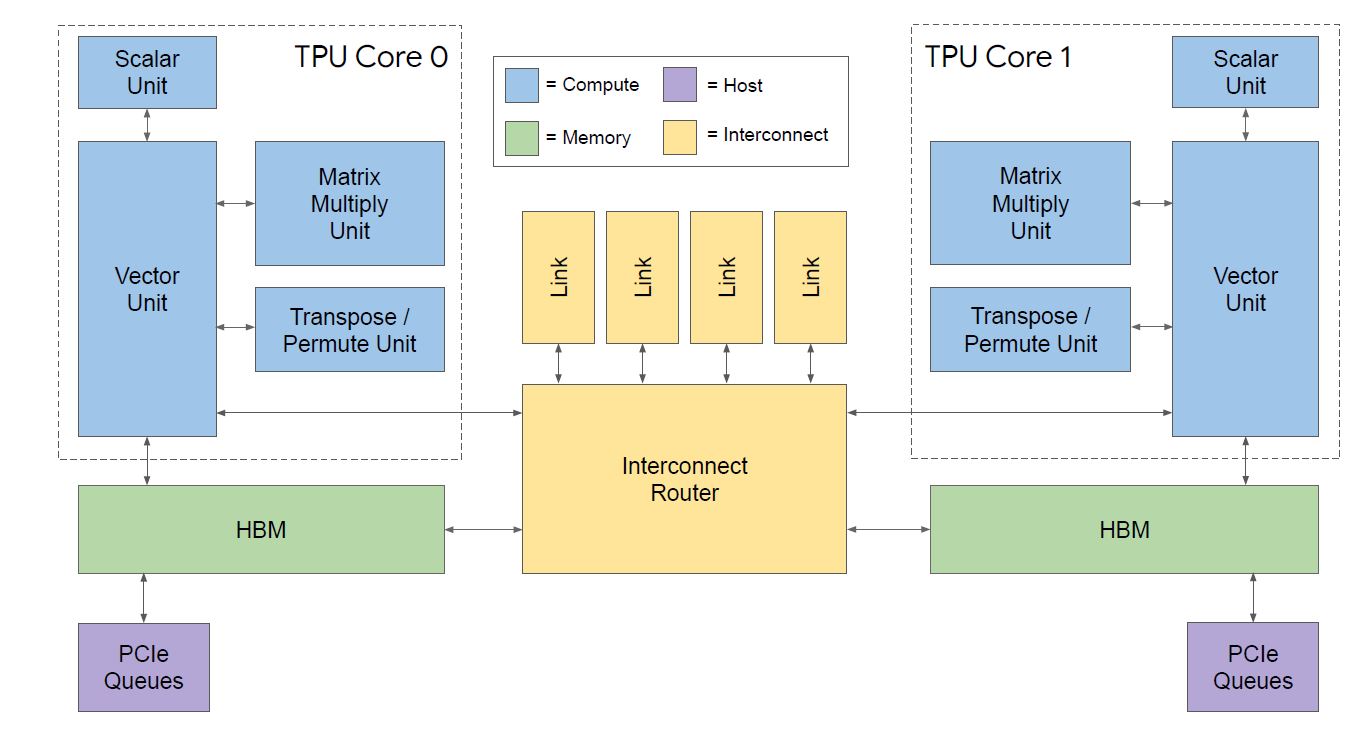
A big change with TPUv3 is that the matrix multiply units are doubled, just like the HBM capacity. This is an ugly image as we took it from the PDF version of Google’s talk. The presented version did not have the diagram over the text. It is somewhat amazing that Google can make massive AI training systems and index the world, but Google Slides gives this kind of export to PDF experience in 2020.
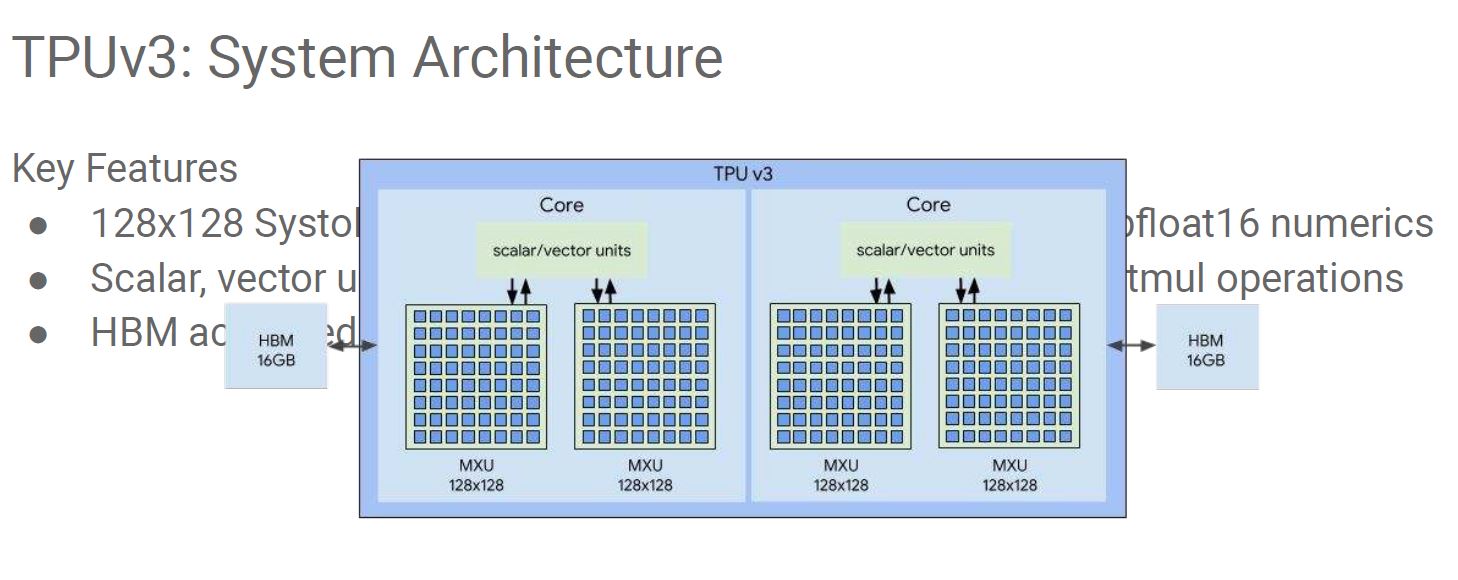
There are a number of other improvements beyond the MMU and HBM capacity doubling. The core frequencies, memory bandwidth, and interconnect link bandwidth all went up by 30% as well. When you see “4x nodes” in this diagram below, that is referring to the number of nodes per pod is scaling to 4x the previous generation (1024 up from 256.)
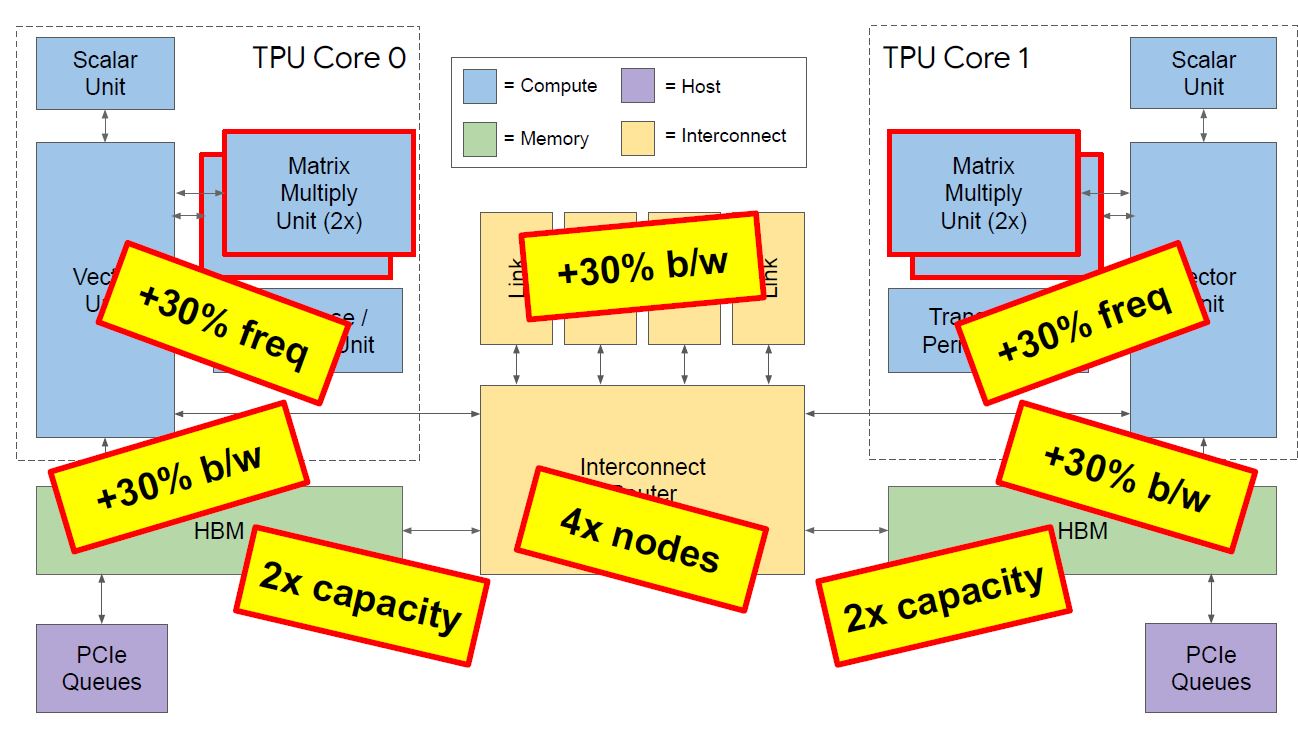
The TPUs are connected via a 2D Torus network for high-speed accelerator communication. There is also a PCIe link to host machines that provide the link to storage.
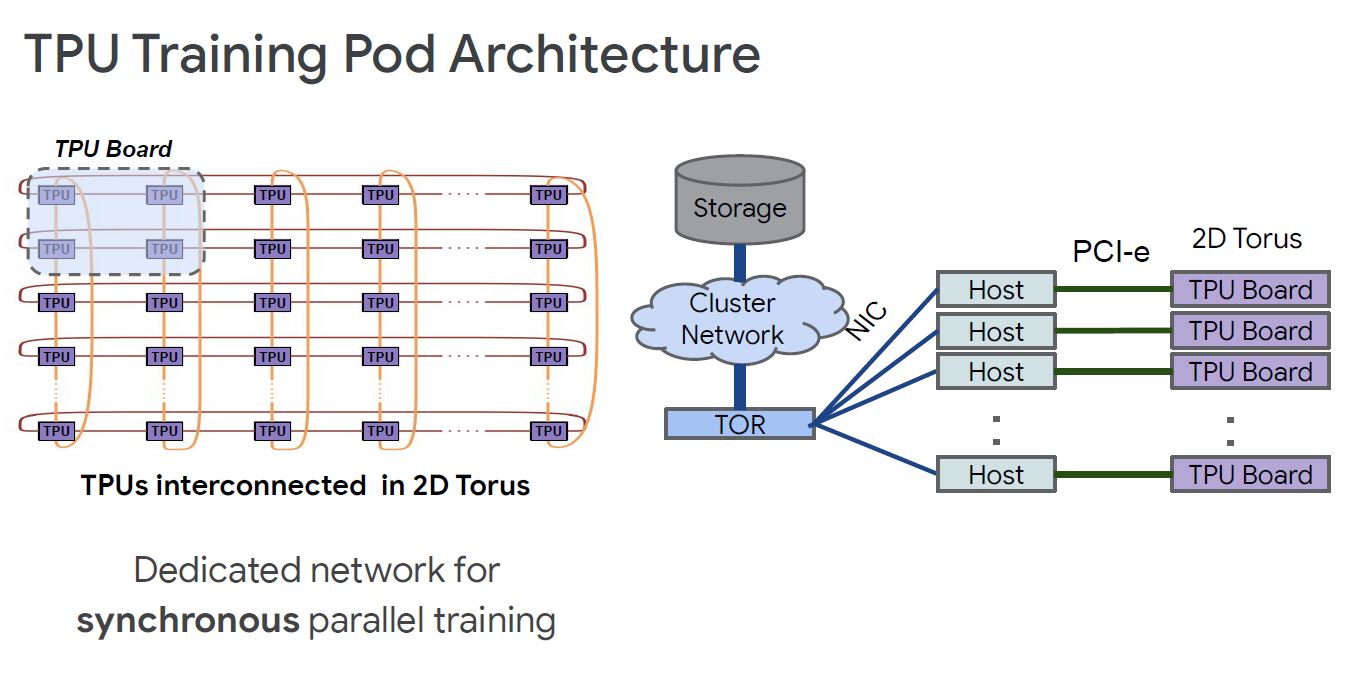
Taking a step back here, there are quite a few people who think that this is completely different from what NVIDIA is doing. As we showed in our Inspur NF5488M5 review as well as our NVIDIA DGX A100 and NVIDIA DGX A100 SuperPod look, NVIDIA is doing something similar. We can even look back to our DeepLearning12 Tesla P100 build and see a similar theme. The NVIDIA A100 (and V100’s) have an interconnect on a HGX-2/ HGX A100 board. That scales from 4x A100 GPUs HGX Redstone Platform up to 8 or 16 GPUs per platform with NVSwitch. Interconnect is NVIDIA-Mellanox Infiniband that with GPUDirect RDMA and effectively bypass going to the host to get on the training fabric. For storage, one goes back to the host system and storage is via another NIC.
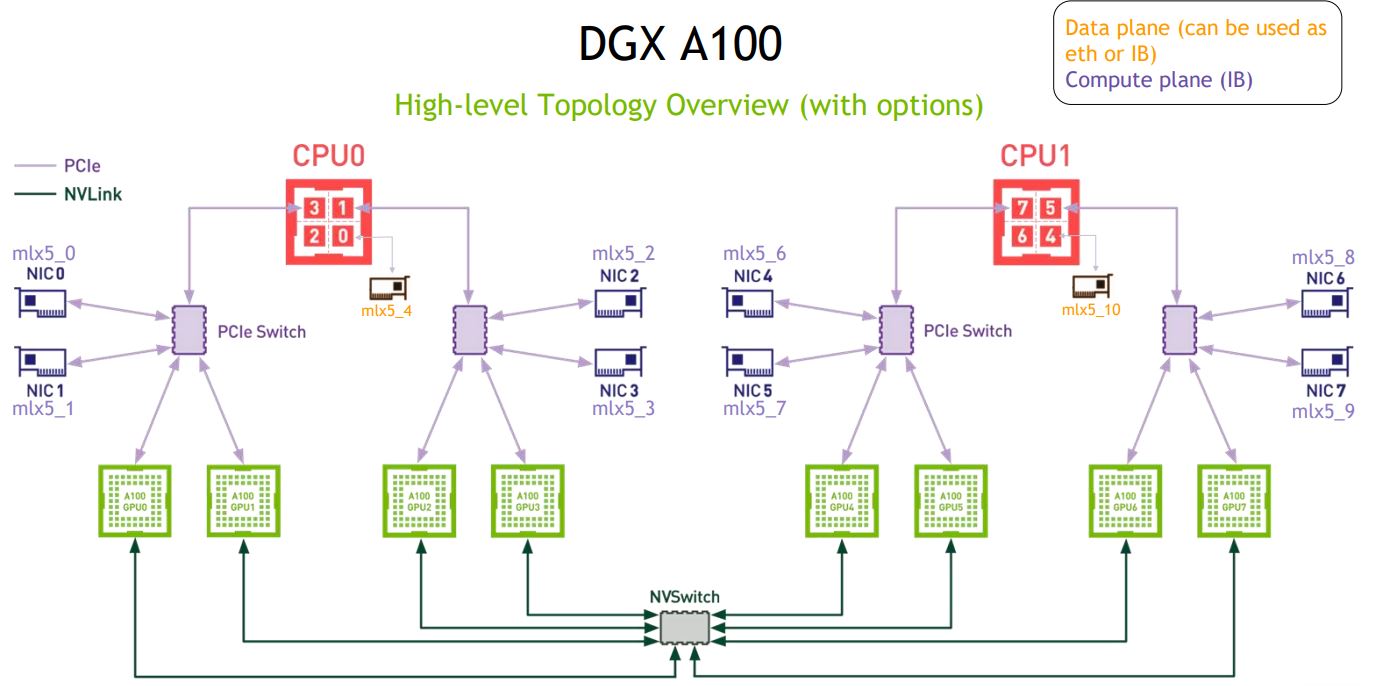
There are a few differences and some significant ones, but we just wanted to point out that there are, indeed, a lot of similarities in how the solutions are built at a high-level. From this high-level, we are comparing a BMW to a Tesla (car) rather than a BMW automobile to a bullet train.
The TPUv2 Google showed using large air coolers. With the TPUv3 we get liquid cooling which means a lower server height. As a result, the standard rack seems to have gone from the TPUv2’s 4×8 four across and eight high of TPUv2 boards to 2×16 of TPUv3 boards.
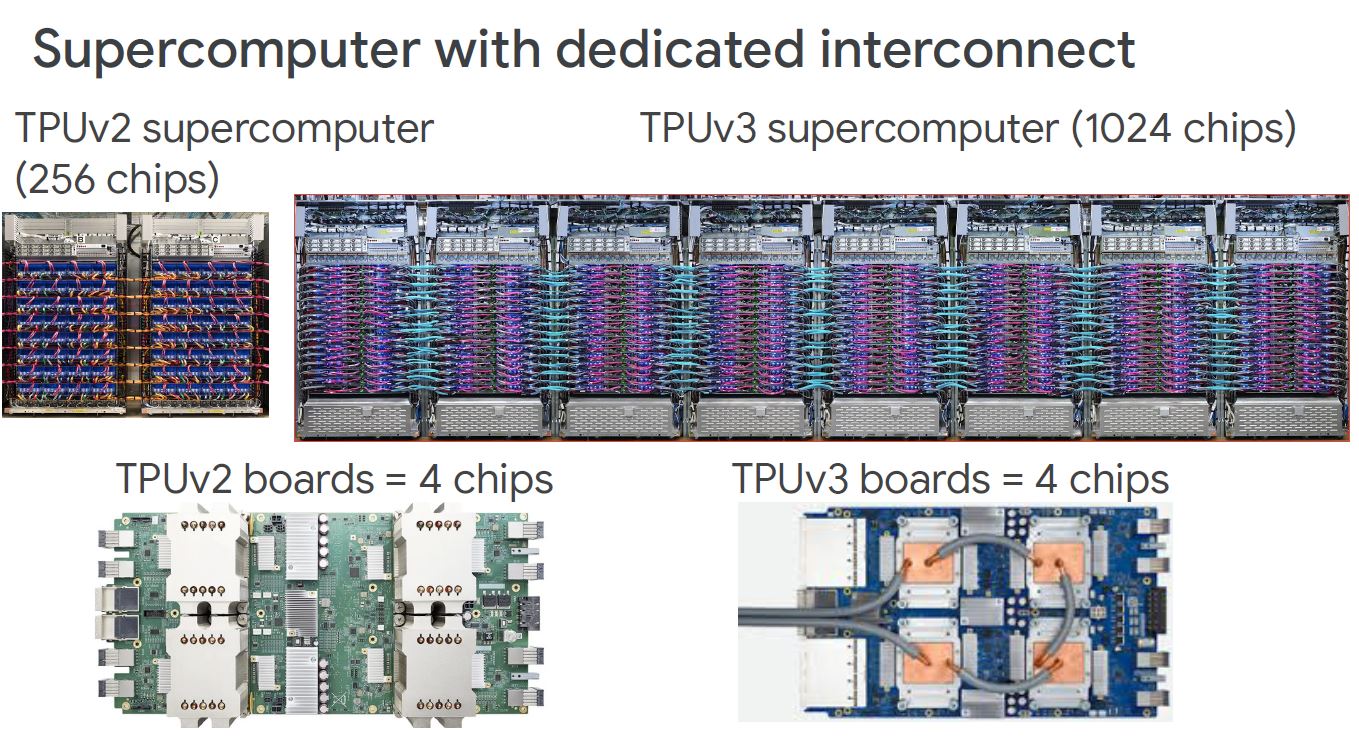
There are other changes, however, at the system level, we have 4x the number of accelerator chips but 9-10x the petaflops and 8x the memory. We can also see a big change to the cabling connectors on the left side of these nodes.
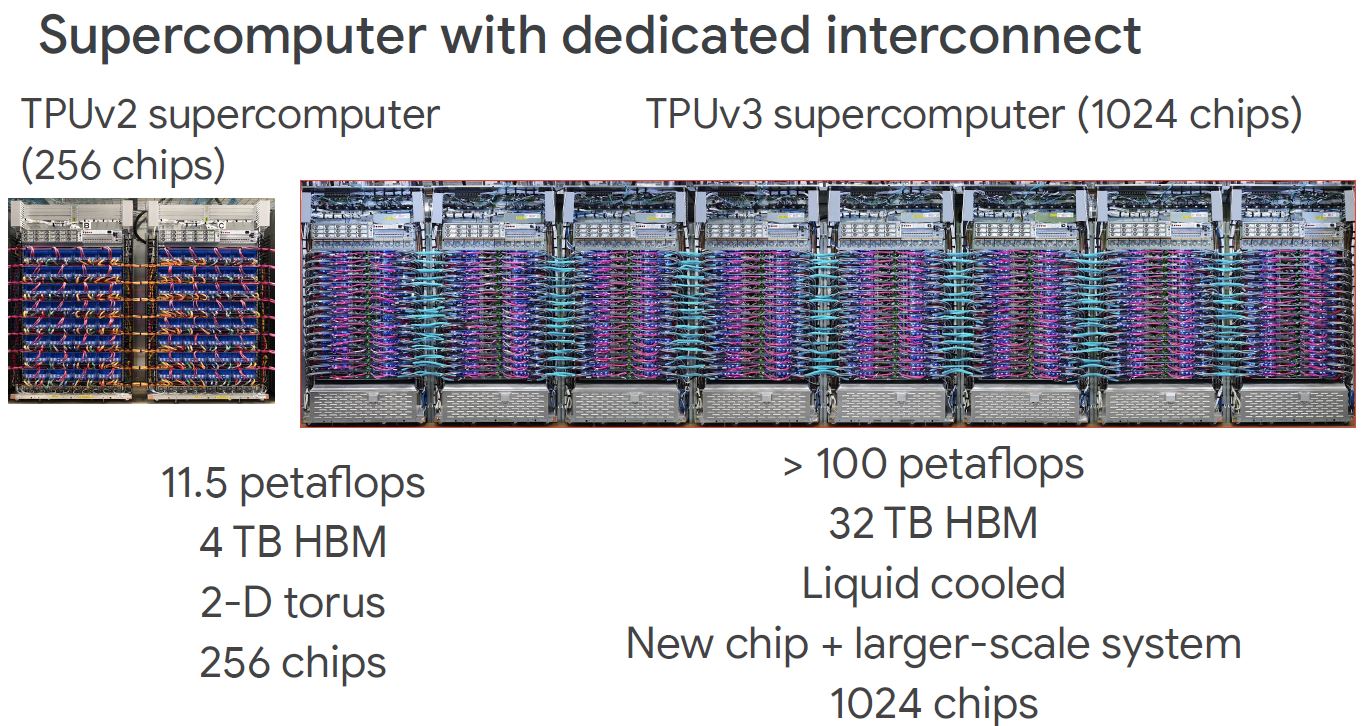
At Hot Chips 32, the company talked more about the software scaling they were seeing which lead to their recent MLPerf 0.7 Training Results. Google uses this hardware (or perhaps a newer generation that they are not talking about) internally.
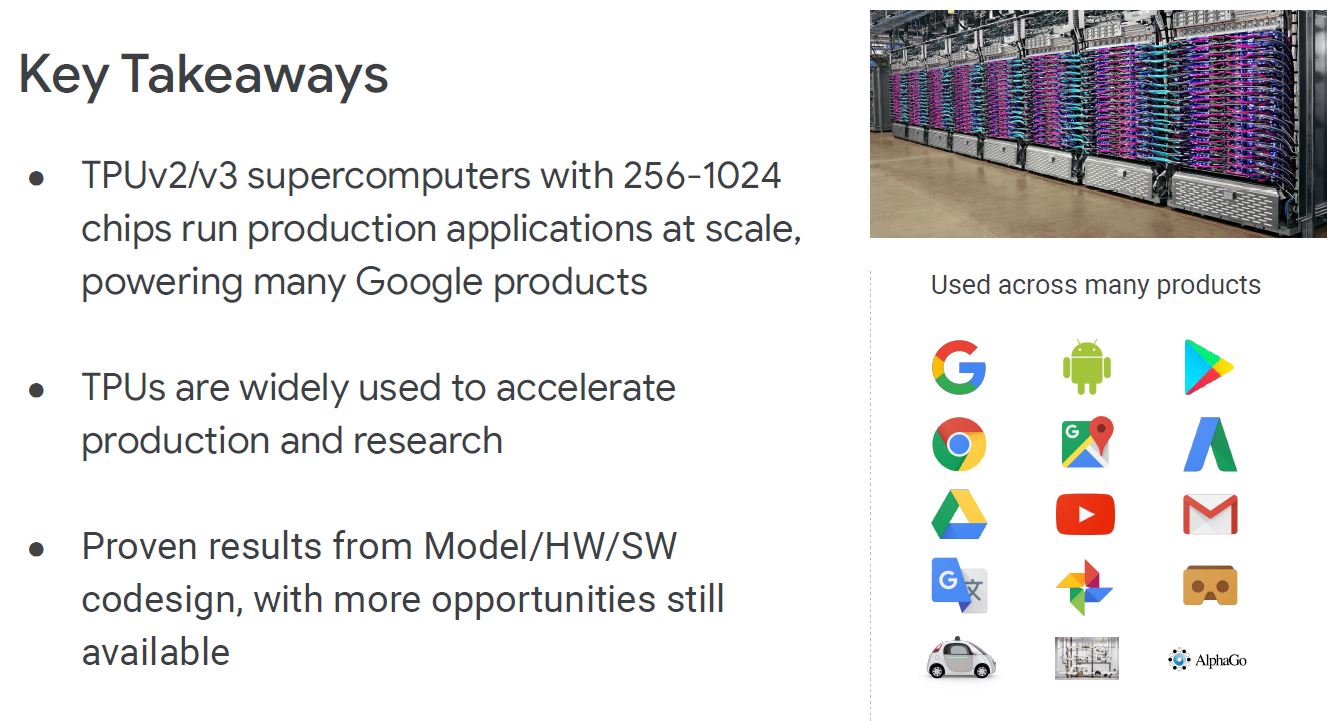
NVIDIA also saw the need to build a large internal-use cluster to aid in software development as we saw in 0 to Supercomputer NVIDIA Selene Deployment.
Final Words
The TPUv3 was announced in 2018 so hearing talk on it with a lot of obfuscated details in 2020 seems strange. This is especially so given the relatively open manner NVIDIA offerings are discussed. That may be due to NVIDIA having partners and a stack that is scaling from low-power to high-power chips and software across multiple domains. Still, we wish Google had used its multiple talks at Hot Chips 32 to go into more detail about the systems themselves especially since we are around two years after TPUv3 announcement. Google tends to be relatively opaque on its hardware (e.g. there is very little public information on the company’s Arm CPU usage.) Facebook and Microsoft tend to be much more open, AWS is somewhere in the middle. At this point, given Google’s scale, they are hard to imitate except for the other hyper-scale outfits. While it is great to see more, we wish Google would let its engineers show off their engineering prowess a bit more.
In the motorcycle versus electric car comparison between the A100 and the TPUv3 another significant difference is that Nvidia is selling hardware that fosters competition while Google is the single and only service provider where the TPUv3 will be available. This also has consequences for data security, especially at the international level.
Forgive me if I come off as somewhat Luddite, but why do we need all this AI nonsense? Is there really any good usage case for artificial intelligence of any kind? Or is this “Big Tech’s” way of obsoleting human beings and human jobs? I don’t personally have a need for AI and will likely never need or own any kind of robot device that uses AI as it will be much too expensive for my unemployed broke self. Plus, this stuff is too dangerous, too unpredictable to be even a little safe or “good” and that ain’t good.