At Google I/O 2017 the company revealed information on what it calls the Cloud TPU. Along with the immensely popular TensorFlow machine learning framework, Google is revealing its next step for dedicated hardware. Google previously revealed that it is making its own hardware to support machine learning, specifically the TPU. While many companies rely heavily on Intel CPUs and NVIDIA GPUs, Google’s scale is leading them down a path of dedicated hardware.
Google Cloud TPU Details
Google released photos and details of the Cloud TPU. Each TPU is capable of 180 teraflops of performance for training and inferencing. The picture that was shared has what appears to be eight large ASIC heat pipe coolers.
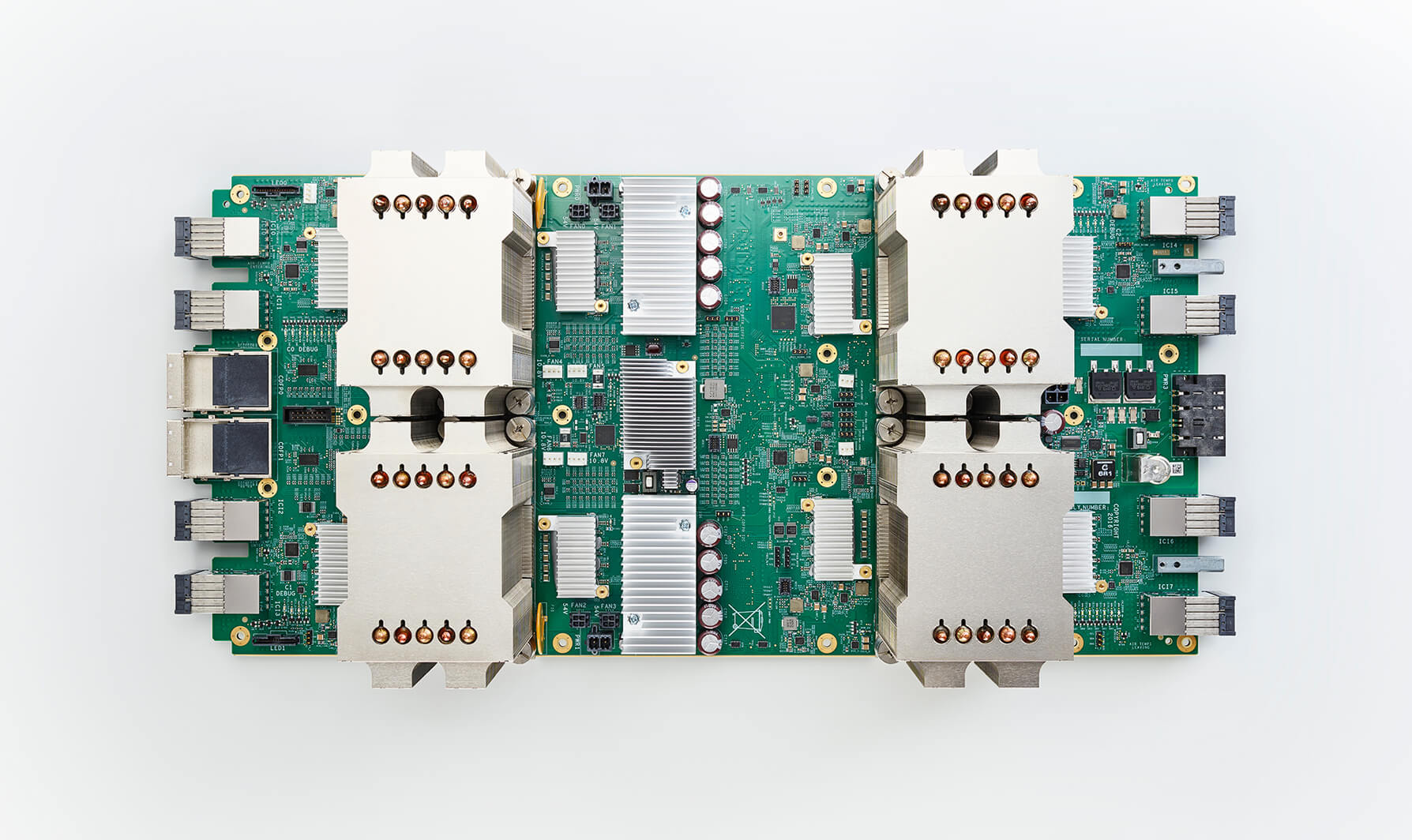
These large socketed ASICs along with large coolers are becoming a more popular form factor. Another example we saw at Super Computing 2016 was this IBM Power plus NVIDIA P100 NVLINK server:
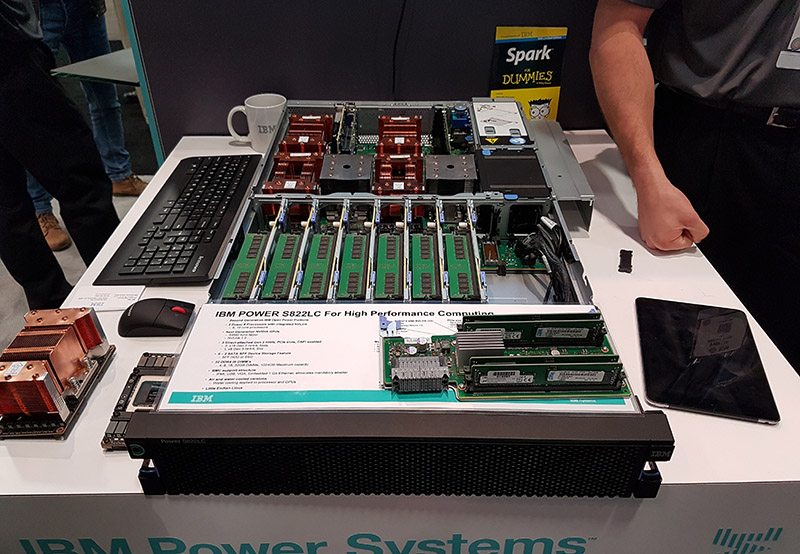
You can see that these high-power designs are eschewing traditional PCIe add-on card form factors instead of focusing on providing optimal cooling via tower coolers oriented perpendicular to the motherboard.
Where Google Sees the Machine Learning Hardware Market
One tidbit that we saw was that in signing up for more information Google had a survey. In the survey, Google asked what hardware users currently are training on. Here are the options:
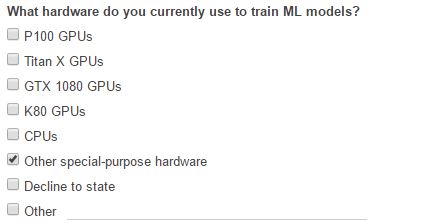
We received a lot of user feedback around a recent assertion in our NVIDIA Deep Learning / AI GPU Value Comparison Q2 2017 that AMD GPUs, given recent press, should have been included. It is very telling to see that Google only lists five main architectures. Four are NIVIDA GPUs, one is (Intel) CPUs. AMD GPUs, FPGAs and even Google’s own TPUs would fall into one of the “Other” categories.
Google TensorFlow Research Cloud
Google also stated that it would be donating 1000 TPUs to the TensorFlow Research Cloud in order to speed adoption and see what users would come up with. In addition, the Google Cloud Platform will have TPUs available with NVIDIA GPUs and Intel CPUs (including Skylake-SP already) to utilize for deep learning workloads.
By offering customized hardware alongside its cloud platform and open machine learning software platform, Google is aggressively targeting the next generation of applications that will have increasing prominence over the next few years.