Gigabyte W291-Z00 GPU Performance
Frameworks change rapidly in the deep learning space, as do software optimizations. As a result, we are looking for two main factors in our GPU performance figures. First, can the system perform well with the NVIDIA Tesla V100 GPUs. Second, can the system keep the GPUs properly cooled to maintain performance over time.
Inspur NF5468M5 P2P Performance
With our system, we have the ability to do peer-to-peer GPU-to-GPU transfers due to the fact that we are using 4x NVIDIA Tesla V100 32GB PCIe GPUs. This is not the same as we may see using other GPUs without P2P enabled in these scenarios. Instead of parsing these out too finely, here is the output of the p2pBandwidthLatencyTest tool:
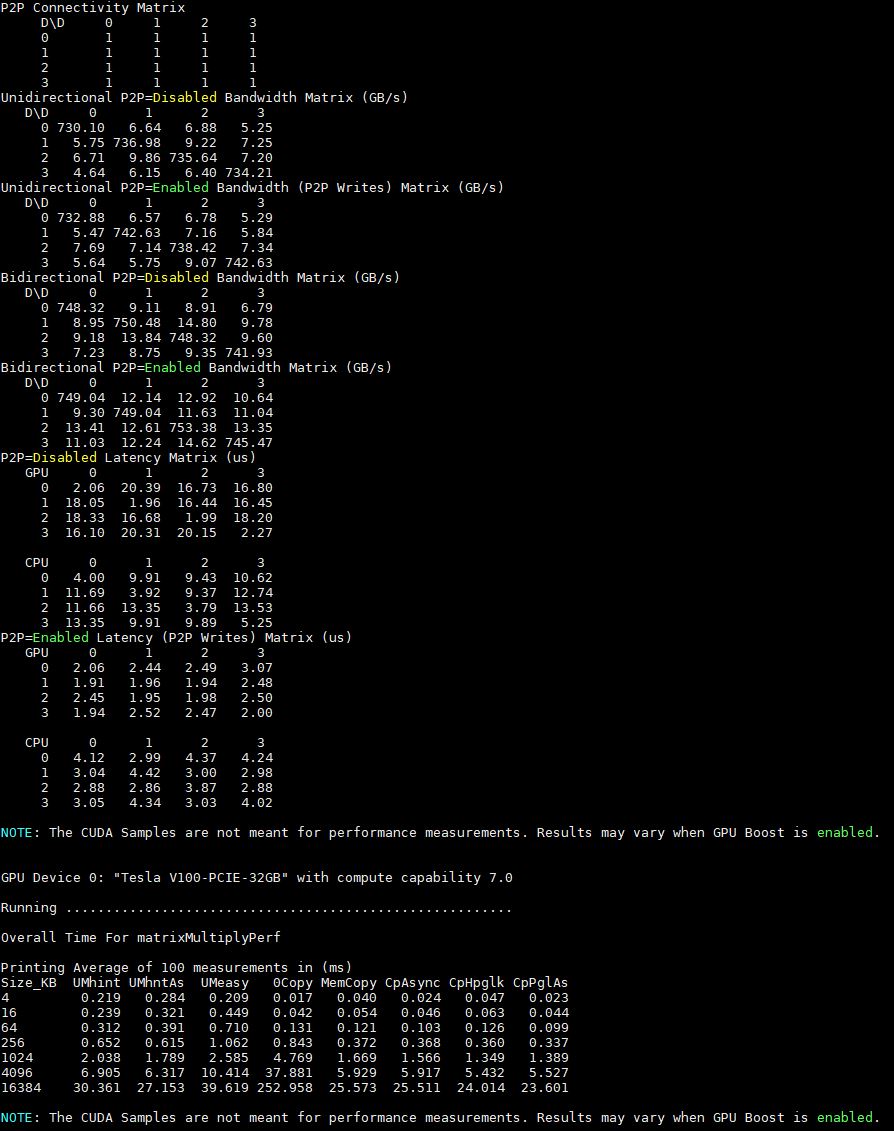
One can see that we get generally good performance, with a caveat. The GPU-to-GPU latencies are higher going across AMD EPYC Infinity Fabric than going across a PCIe switch architecture. For HPC applications, there is some benefit having all GPUs directly attached to each die in a 1:1 ratio since those tend to see more RAM to GPU transfers and this solution removes an extra hop.
We are going to take a look at the performance using some comparison points of 8x Tesla P100/ V100 GPU servers we have tested as well.
Gigabyte W291-Z00 TensorFlow Resnet-50 GPU Scaling
We wanted to give some sense of performance using one of the TensorFlow workloads that we utilized. Here, we are increasing the number of GPUs used while training Resnet50 on Imagenet data.
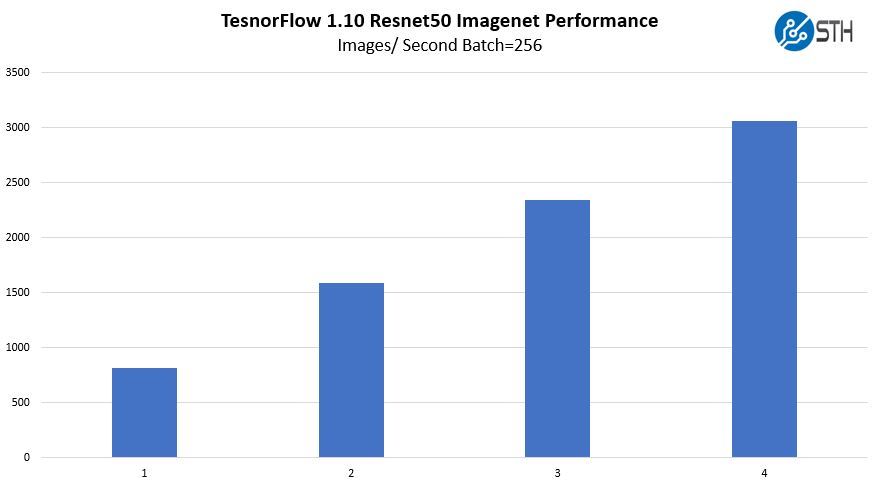
Overall, this is fairly similar to what we would see on an Intel system. These are solid results
Gigabyte W291-Z00 with 4x NVIDIA Tesla V100 32GB GPU Linpack Performance
One of the other advantages of a solution like this is the double-precision compute performance. While many in the deep learning community are focusing on lower precision, there are HPC applications, and indeed many deep learning applications that still want the extra precision that dual precision offers. Linpack is still what people use for talking HPC application performance. NVIDIA’s desktop GPUs like the GTX and RTX series have atrocious double precision performance as part of market de-featuring. We are instead using some HPC CPUs for comparison from Intel, AMD, and Cavium.
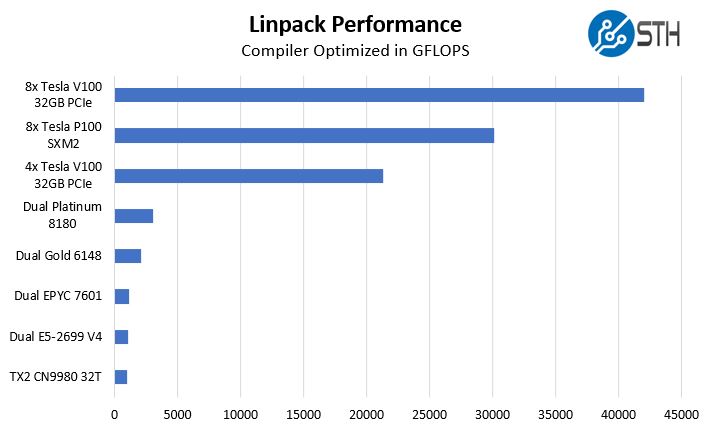
This is generally a good result, in-line with expectations in terms of performance. Most HPC applications will need to scale out, and this particular system would be limited to 1GbE for scaling out to additional nodes.
OTOY OctaneBench 4.00
We often get asked for rendering benchmarks in our GPU reviews, so we added OctaneBench to the test suite recently.
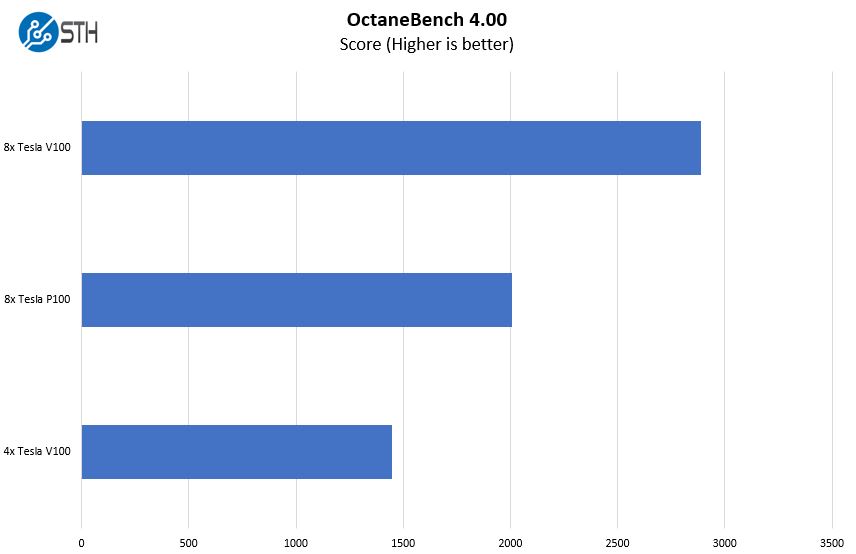
The performance here is solid, and we are not seeing the impact of each GPU hanging off of different NUMA nodes connected via AMD EPYC Infinity Fabric.
Next, we are going to look at AMD EPYC CPU performance across a range of options for the Gigabyte W291-Z00 server.
Why not the MZ31? what, slightly larger dimensions?
https://www.gigabyte.com/us/Server-Motherboard/AMD-EPYC-7000
Gigabyte MZ31-AR0, 180w tdp, two SFP+, 16 RDIMM’s, PCIe 3.0 four x16 & three x8
Gigabyte MZ01-CE1, 180w tdp, two 1GbE, 8 RDIMM’s, PCIe 3.0 four x16 & one x8
Excellent and thorough review as usual. Love the tag
“the Gigabyte W291-Z00 … the only option … is the best option.” Lol 8)
Hi ekv – The MZ31-AR0 does not have enough room behind the PCIe slots to fit full-length GPUs. You can read more about it in our Gigabyte MZ31-AR0 Review.
Thanks for this review, Patrick! Great info. I don’t suppose you have four mi60’s kicking about? An all AMD test would be very cool.
Hi hoohoo – unfortunately not. I agree it would be interesting, but it is a bit hard for us to fund that type of project.