In terms of AI startups, Cerebras has been our front-runner to make it to the next stage for years. Now, it seems to have exited a gaggle of startups scaling its giant wafer scale engine to AI supercomputer scale (for revenue.) At Hot Chips 2023, the company is detailing the new cluster that it plans to use to dwarf what NVIDIA is building.
We are doing this live, so please excuse typos.
Detail of the NVIDIA Dwarfing Cerebras Wafer-Scale Cluster
Cerebras started the presentation with a company update and that AI/ ML models are getting bigger (~40,000x in 5 years.) They also discussed some of the history of ML acceleration.
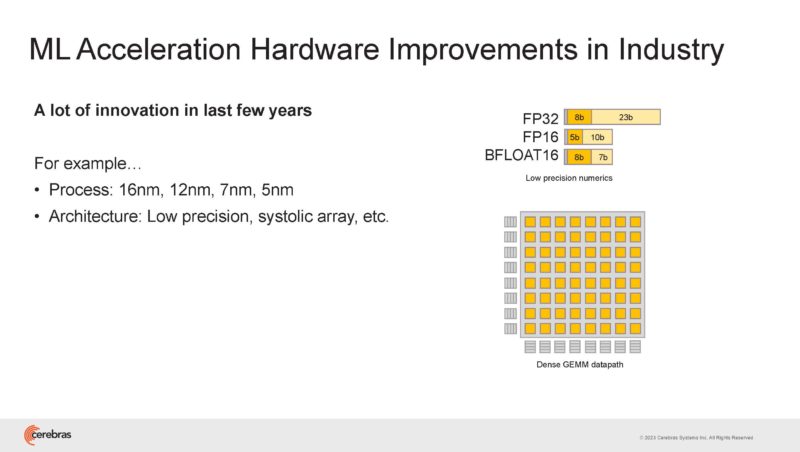
Process technology has given gains over time.
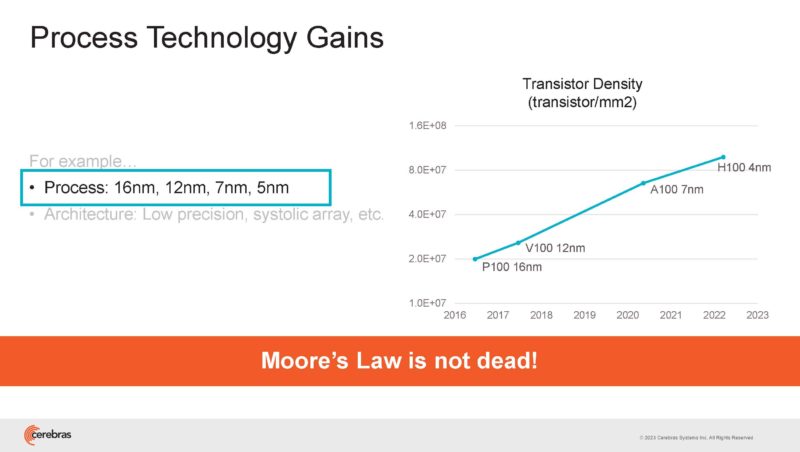
Architecture gains such as changing calculations from FP32 to bfloat16, INT8, or other formats and techniques have also given huge gains.
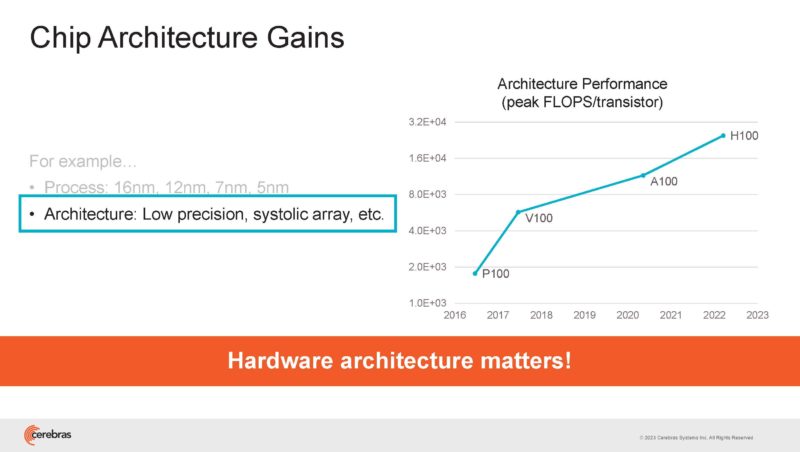
Still, what models are practical to use depends on the ability to not just get gains at a chip level, but also at the cluster level.
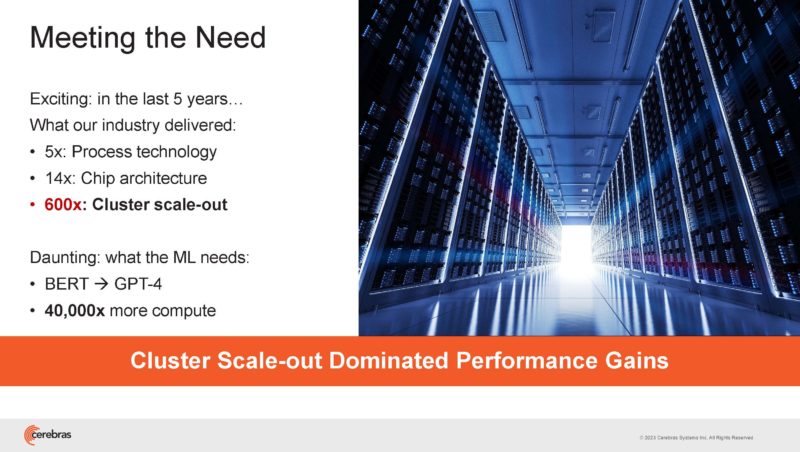
Some of the challenges of current scale-out is just the communication needs to keep data moving to smaller compute and memory nodes.
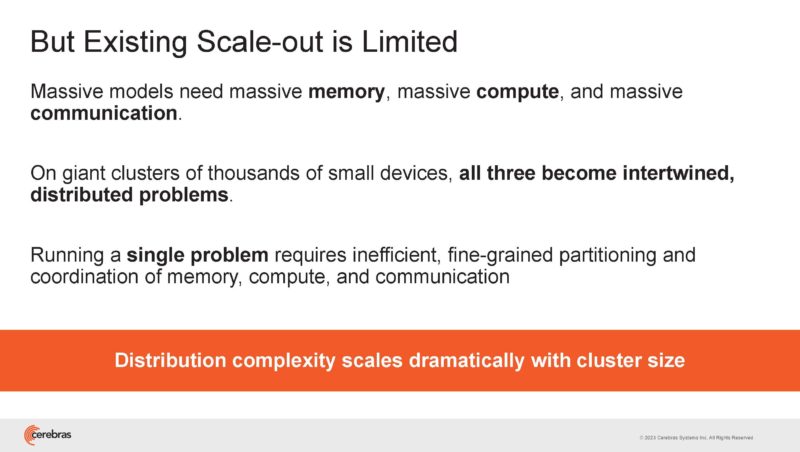
Cerebras built a giant chip to get an order-of-magnitude improvement, but it also needs to scale out to clusters since one chip is not enough.
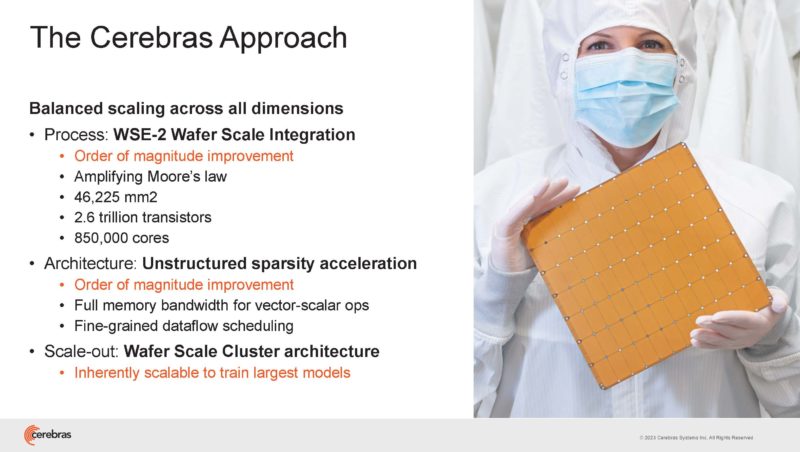
Traditional scale-out has challenges because it is trying to split a problem, data, and compute across so many devices.
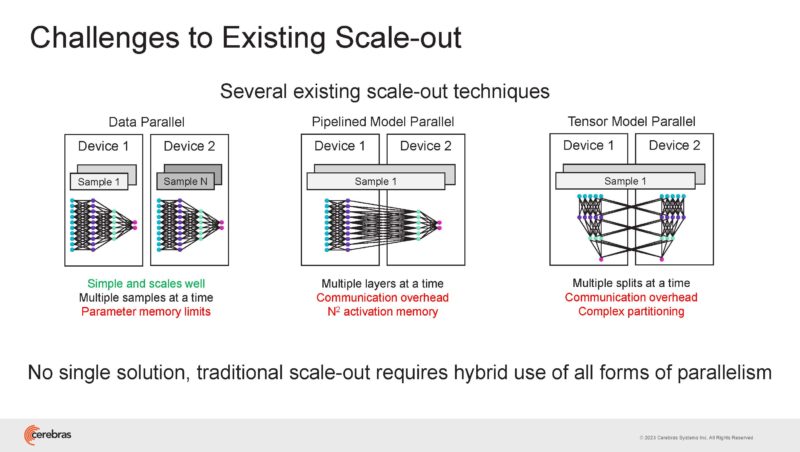
On GPUs, that means using different types of parallelism to scale out to more compute and memory devices.
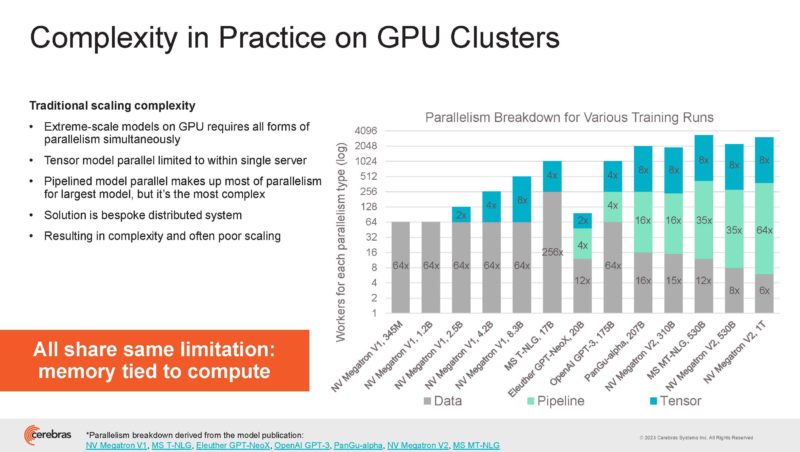
Cerebras is looking to scale cluster level memory and cluster level compute to decouple compute and memory scaling as is seen on GPUs.
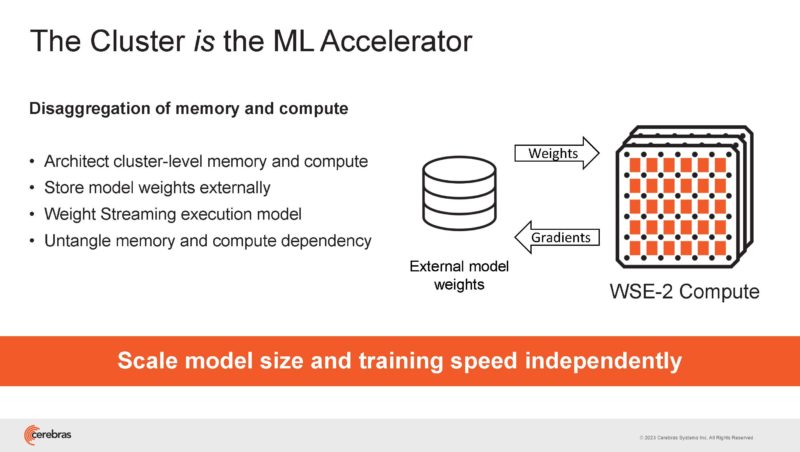
Cerebras has 850,000 cores on the WSE-2 for its base. When will we get a 5nm WSE-3? Sounds like not today.
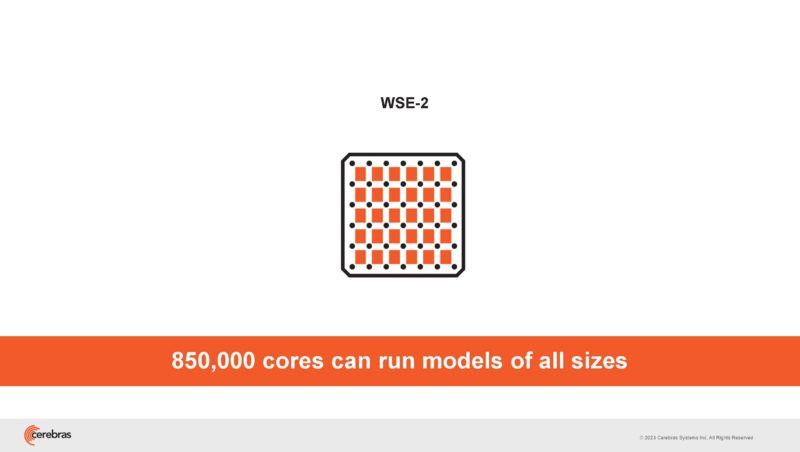
Cerebras houses the WSE-2 in a CS2 and then connects it to MemoryX. It then can stream data to the big chip.
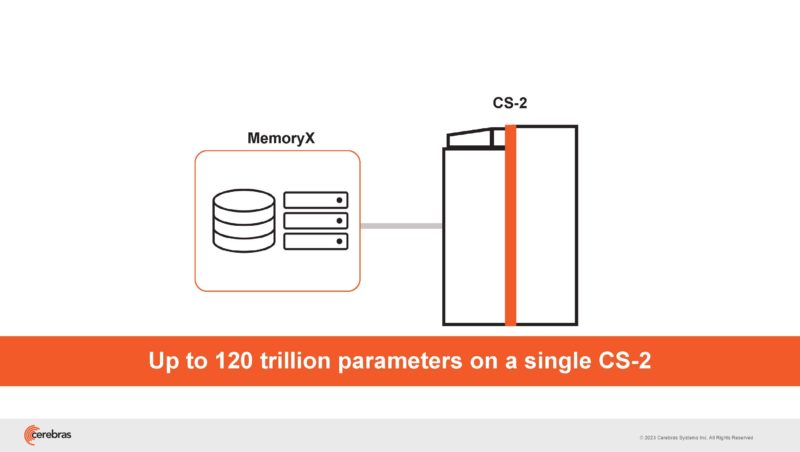
It then has the SwarmX interconnect that does the data parallel scaling.
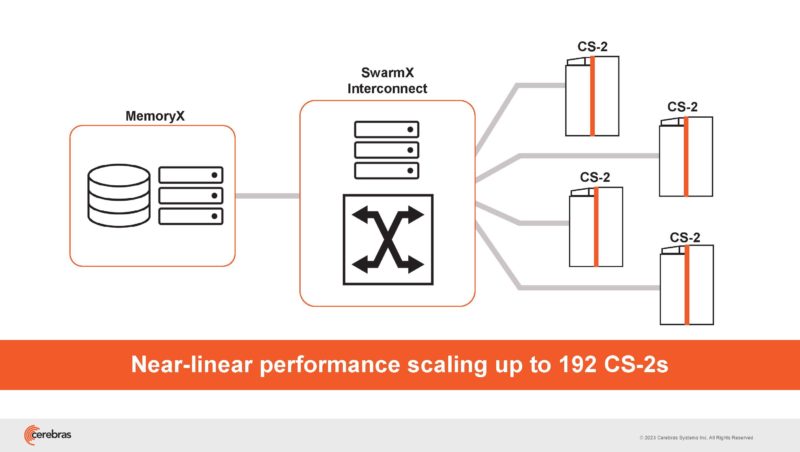
Weights are never stored on the wafer. They are just streamed in.
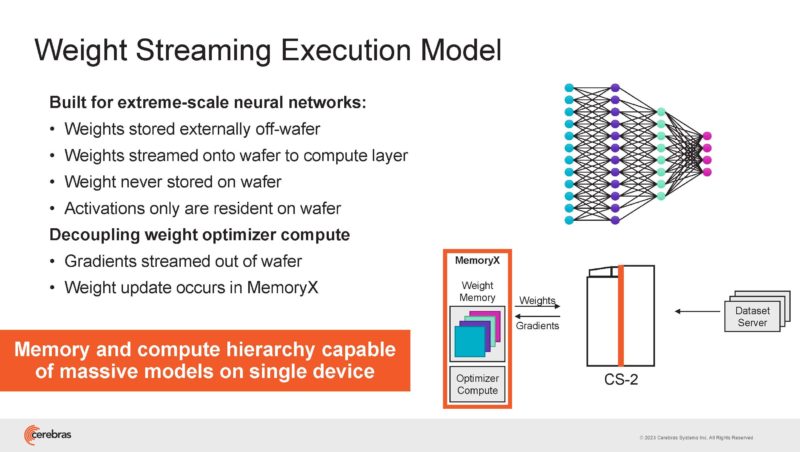
The SwarmX fabric scales weights and reduces gradients on the return.
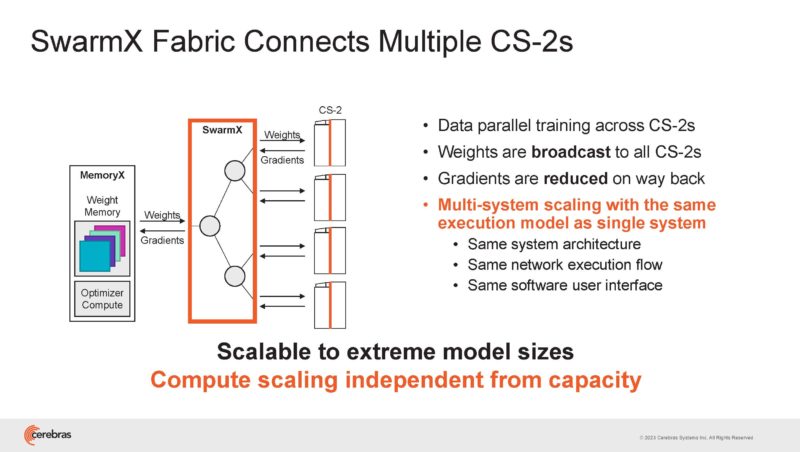
Each MemoryX unit has 12x MemoryX nodes. States are stored in DRAM and in flash. Up to 1TB of DRAM and 500TB of flash. The CPUs are interestingly only 32-core CPUs.
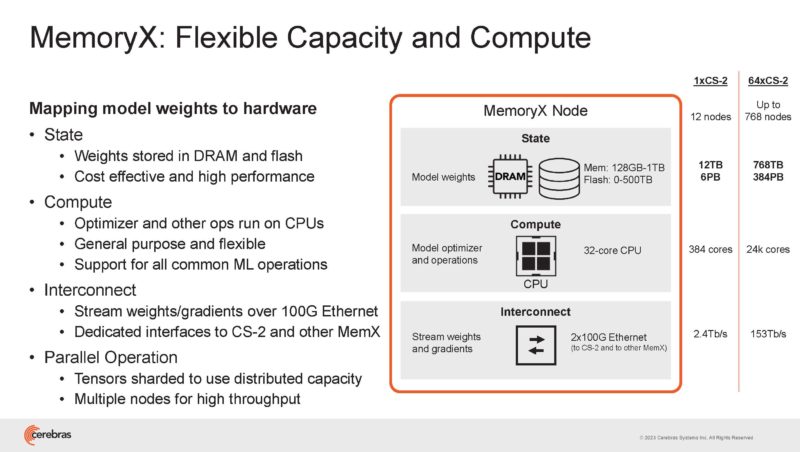
Finally, it is connected to the cluster using 100GbE. One port goes to the CS-2 and one to other MemoryX modules.
MemoryX has to handle the sharding of the weights in a thoughtful way to make this work. Ordering the streaming helps perform an almost free transpose.
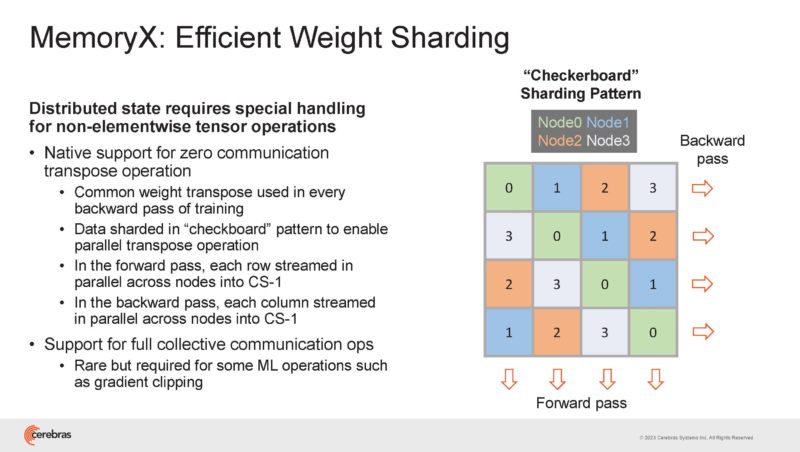
In MemoryX, there is a high-performance runtime in order to transfer data and perform computations.
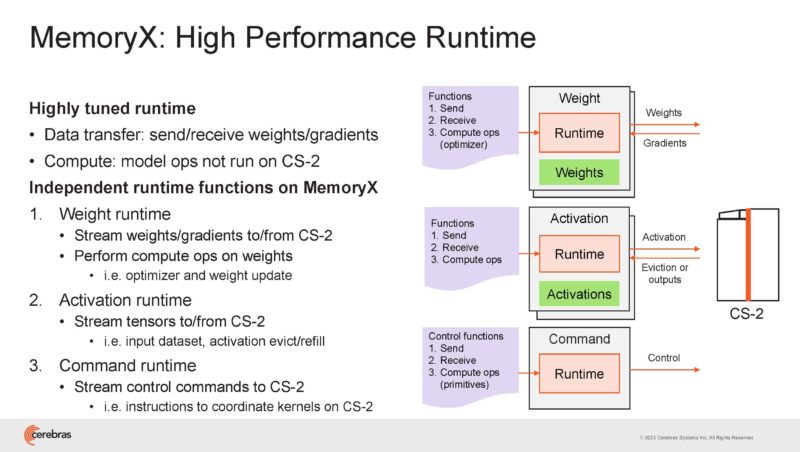
SwarmX fabric uses 100GbE and RoCE RDMA to provide connectivity and Broadcast Reduce that happens on CPUs.
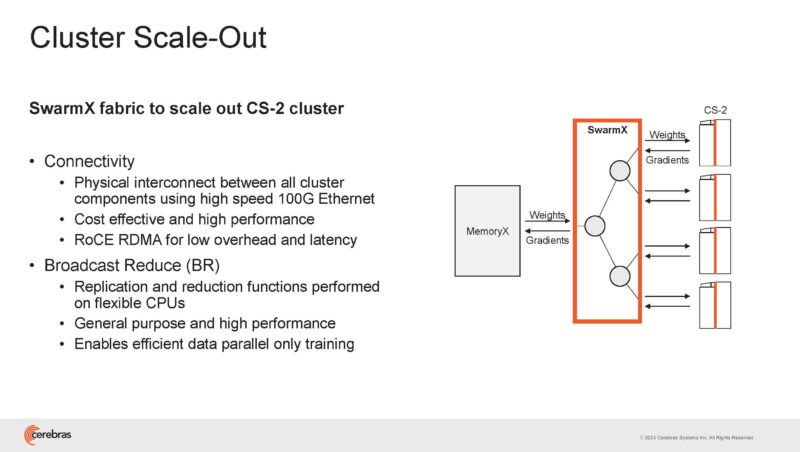
Every broadcast reduce node has 12 nodes with 6x 100GbE links. Five of them are used for a 1:4 broadcast plus a redundant link. That means 150Tbps of broadcast reduce bandwidth.
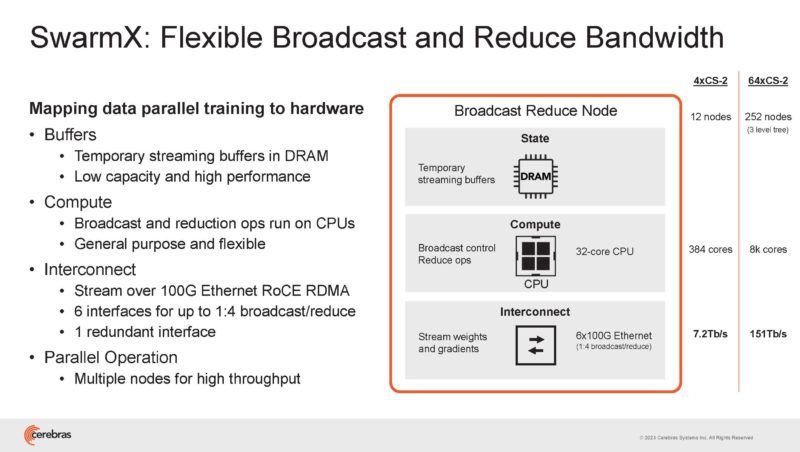
100GbE is interesting since it is now a very commoditized interconnect as compared to NVLink/ NVSwitch and InfiniBand.
Cerebras is doing these operations off of the CS-2/ WSE and that is helping this scale.
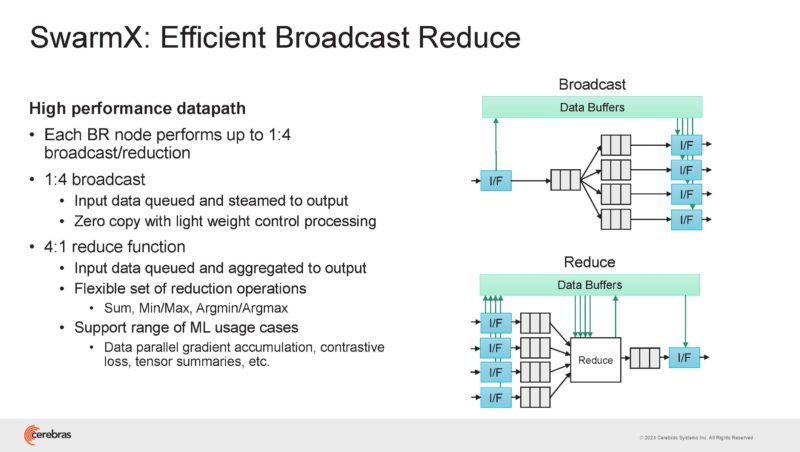
This is the SwarmX topology.
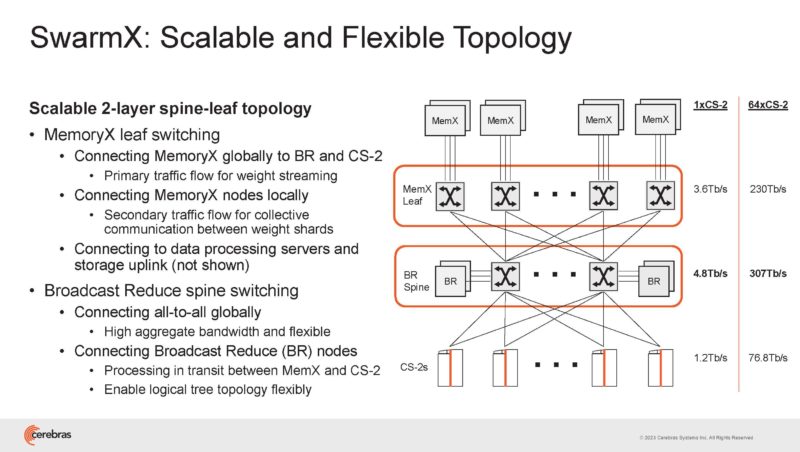
The flexibility in the fabric can be used to effectively provision work across the cluster while supporting sub-cluster partitioning.
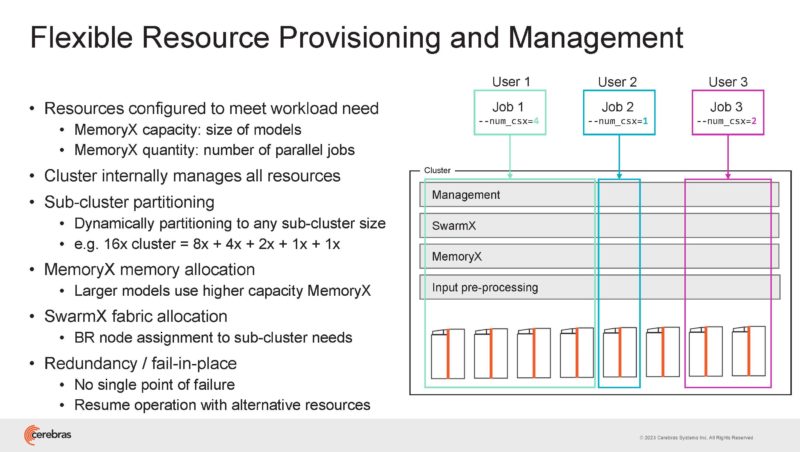
Here is the Cerebras WSE-2 with me at ISC 2022:
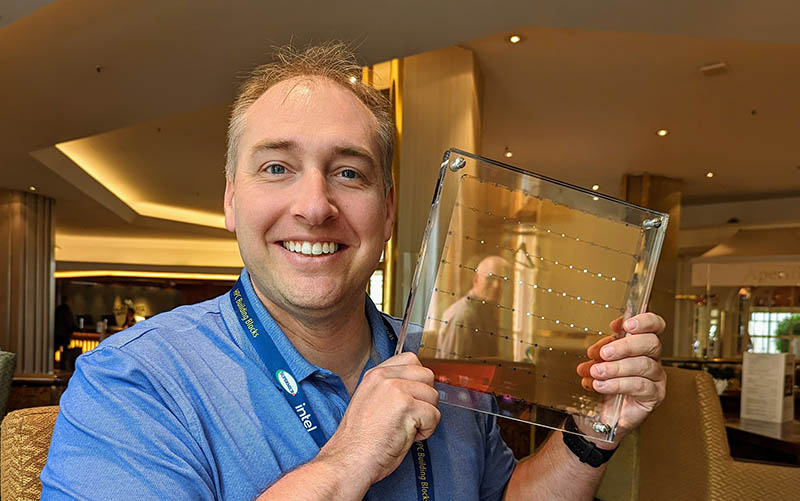
That goes into an engine block that looks like this:
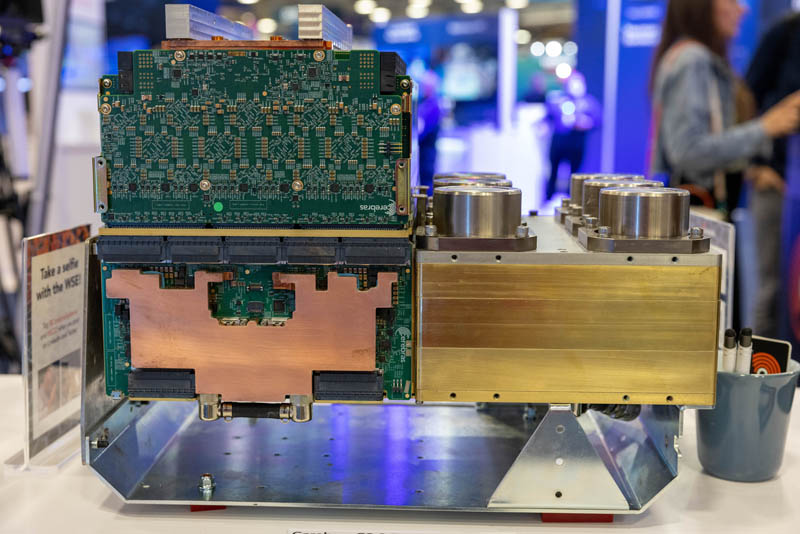
That goes into the Cerebras CS-2.
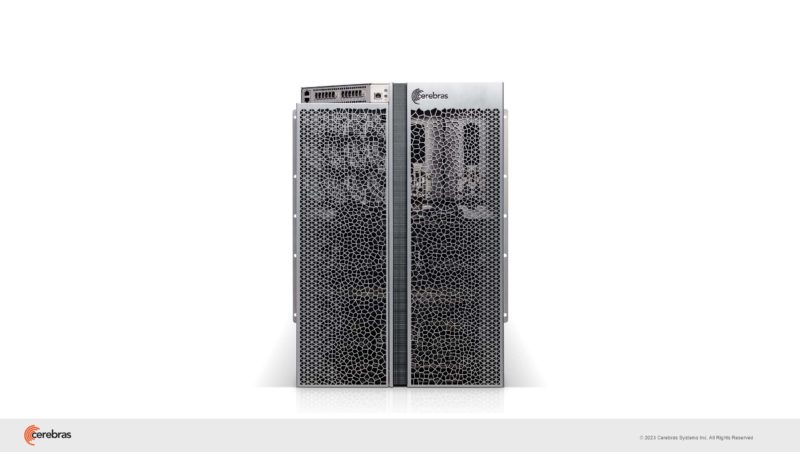
Those were built into racks.
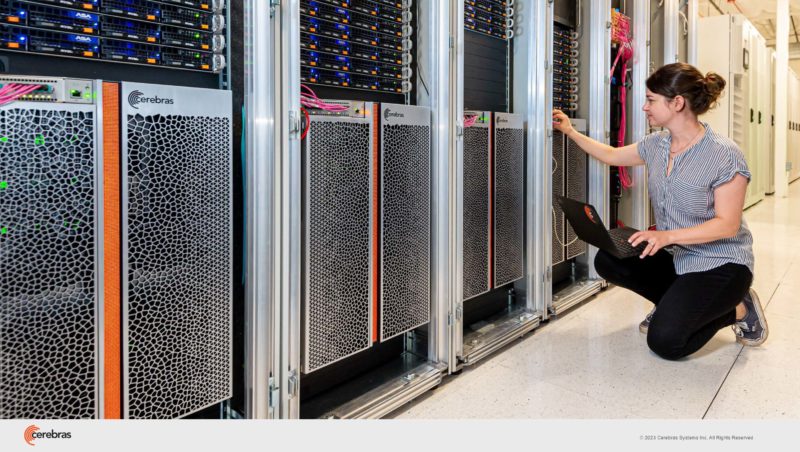
We can say hello to Supermicro 1U servers above the CS-2’s.
Then CS-2’s went into larger clusters.
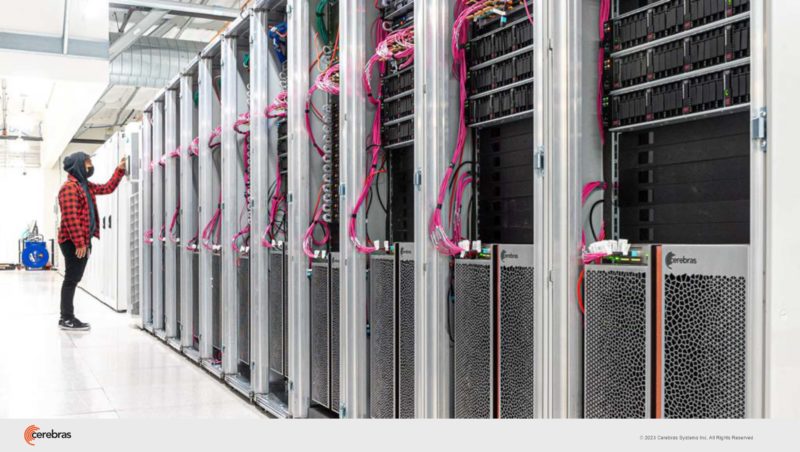
Now bigger clusters.
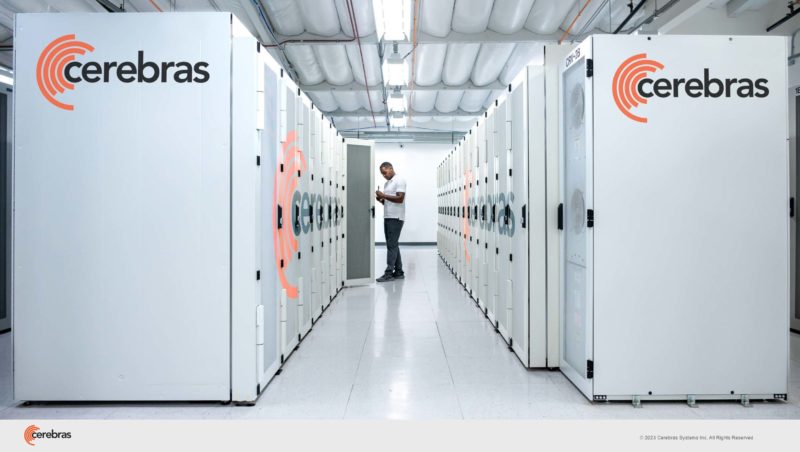
This is the older Andromeda wafer scale cluster.
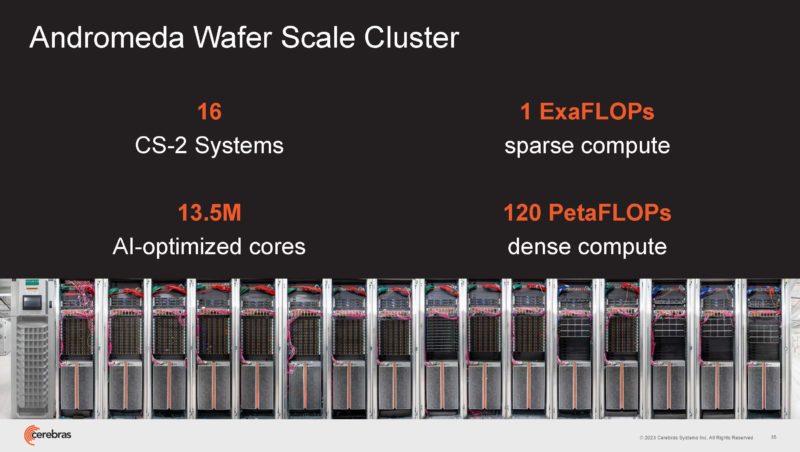
Cerebras was training large models on Andromeda quickly with 16x CS-2’s.
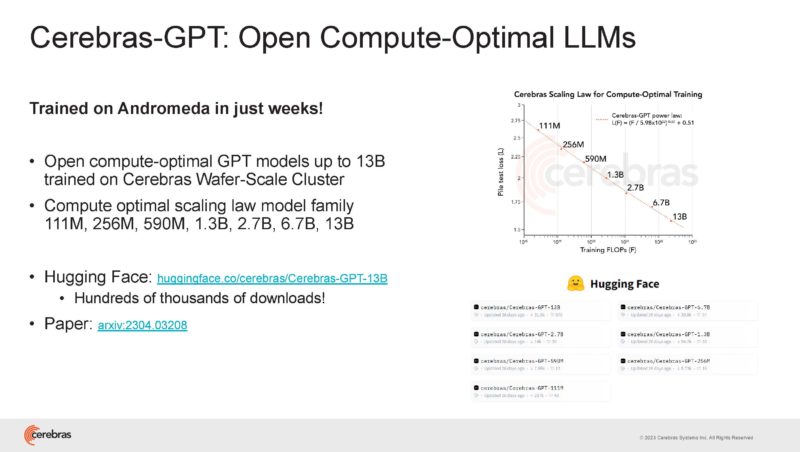
It found that programming a job for a single CS-2 scaled to 16x CS-2’s.
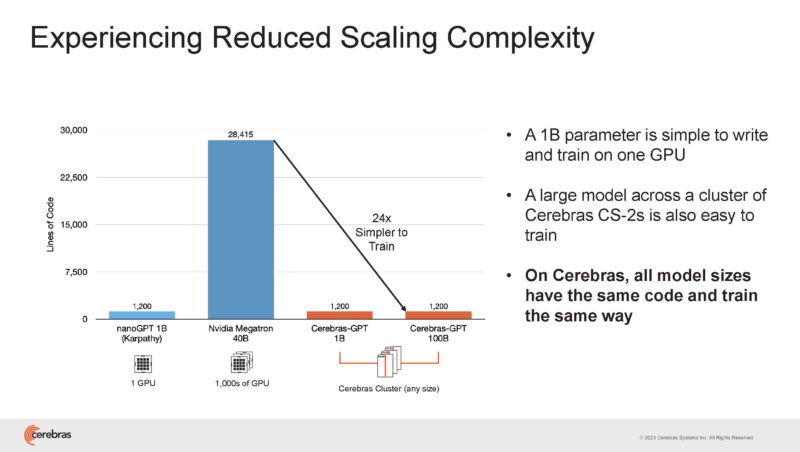
Then Cerebras got bigger with the Condor Galaxy-1 Wafer Scale Cluster that we covered in: 100M USD Cerebras AI Cluster Makes it the Post-Legacy Silicon AI Winner.
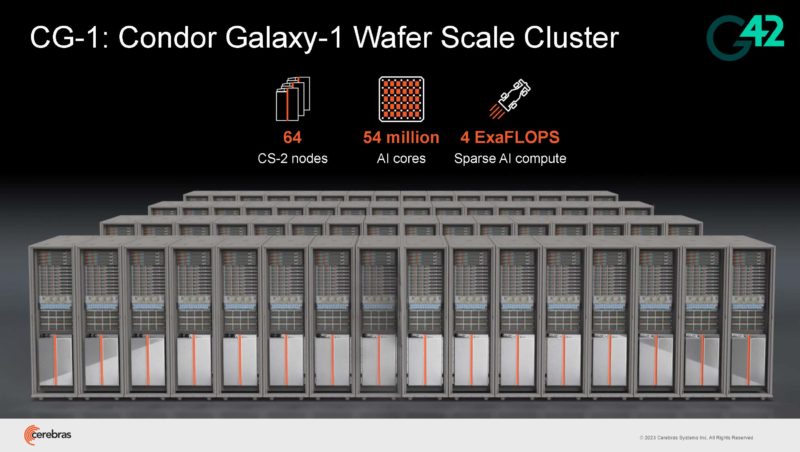
Cerebras traned BTLM on that which is the top 3B model right now.
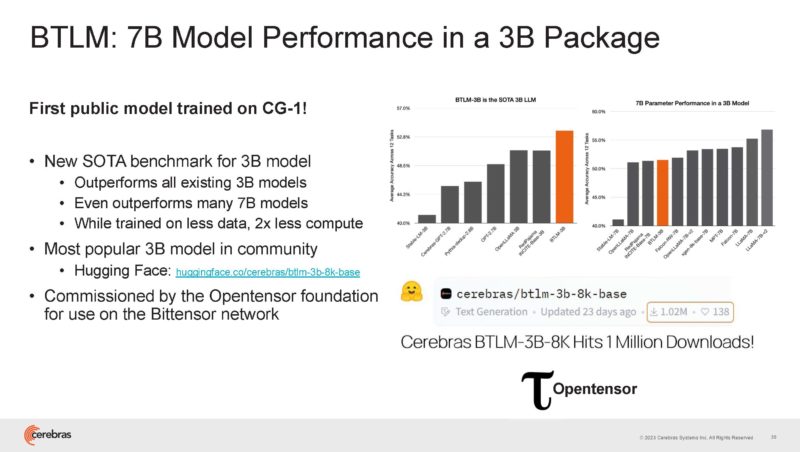
Next, Cerebras is scaling to even larger clusters.
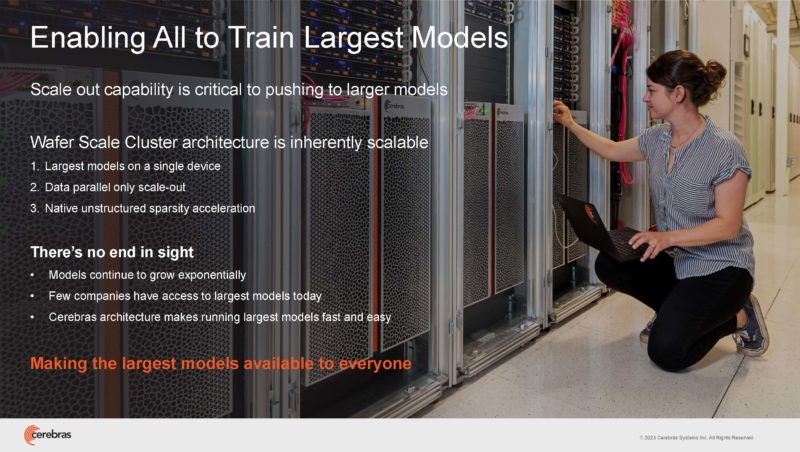
Final Words
I fell pretty far behind covering this talk. Still, Cerebras is big game hunting which is important in the era of big models. Having customers buying huge amounts of hardware to get these clusters online is a big vote of confidence for the company. It is a very different approach to scaling than NVIDIA and companies trying to duplicate NVIDIA.