The Cerebras Wafer Scale Engine is by far one of the coolest chips, and one of the only commercially successful AI chip offerings from a startup. We now have a new third version of the chip called the Cerebras WSE-3. The new chip leverages another process shrink to achieve even more density than previous generations.
Cerebras WSE-3 AI Chip Launched 56x Larger than NVIDIA H100
This is the world’s largest AI chip with over 4 trillion transistors and 46225mm2 of silicon in TSMC 5nm. For those who have not seen our previous WSE-2 coverage, this chip is the largest square that TSMC can make.
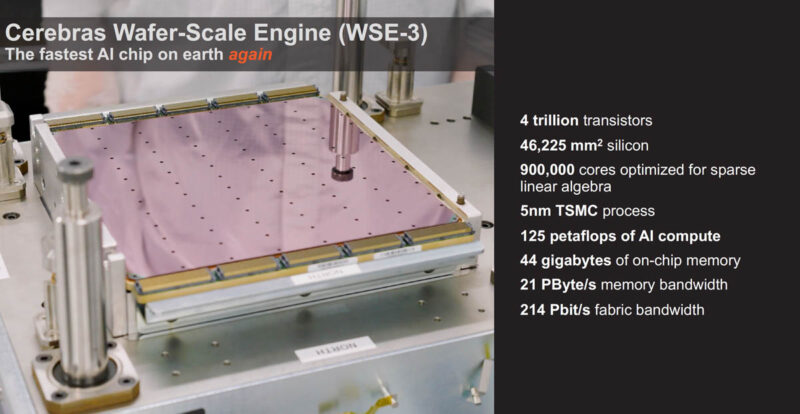
Onboard are 900,000 cores and 44GB of memory. When Cerebras says memory, this is more of SRAM rather than off-die HBM3E or DDR5. The memory is distributed alongside the cores with the goal of keeping data and compute as close as possible.
For a sense of scale, this is the WSE-3 next to he NVIDIA H100.
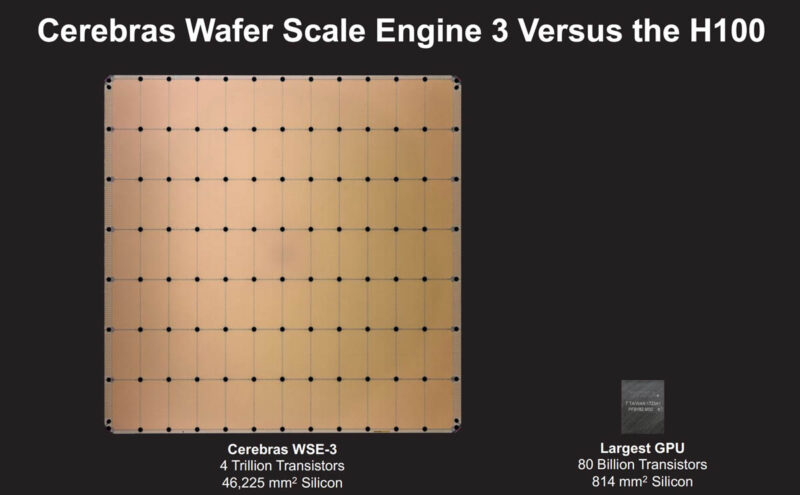
One of the reasons Cerebras has been successful, aside from bringing this giant chip to market, is that it is doing something different than NVIDIA. While companies like NVIDIA, AMD, Intel, and others take a big TSMC wafer and slice it into smaller segments to make their chips, Cerebras keeps the wafer together. In today’s clusters, where there can be tens of thousands of GPUs or AI accelerators working on a problem, reducing the number of chips by a factor of 50+ makes the interconnect and networking costs and power consumption lower. In a NVIDIA GPU cluster with Infiniband, Ethernet, PCIe, and NVLink switches a huge amount of power and cost is spent on re-linking chips. Cerebras cuts that out by just keeping the entire chip together.
Here I am with the original WSE and Andrew Feldman, Cerebras’ CEO and a NVIDIA Tesla V100 in my other hand just before the pandemic.
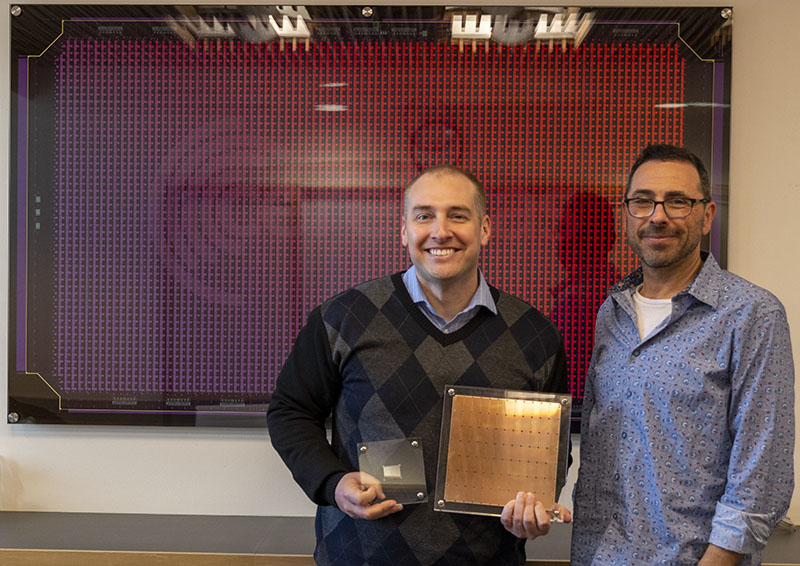
Here is the side-by-side. One item to note is, again, Cerebras uses on-die memory, not on-package memory for NVIDIA, so we do not get the 80GB of HBM3 on the H100 as an example.
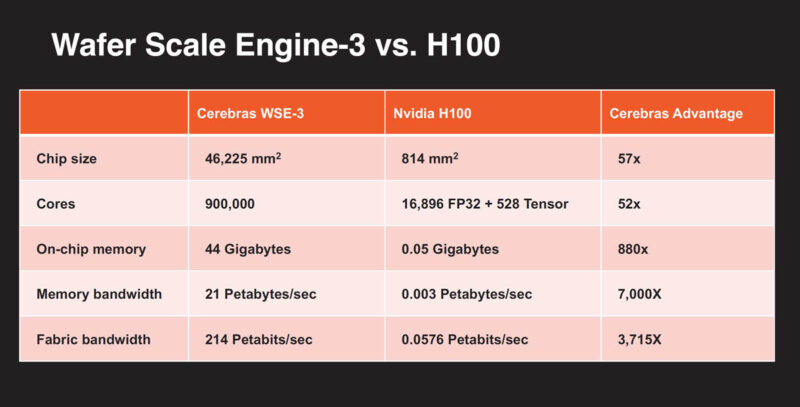
Of course, having a chip is nice, but it needs to fit into a system. That system is the CS-3.
Cerebras CS-3 Wafer Scale System
The Cerebras CS-3 is the third-generation Wafer Scale system. This has the MTP/MPO fiber connectivity at the top, along with power supplies, fans, and redundant pumps for cooling.
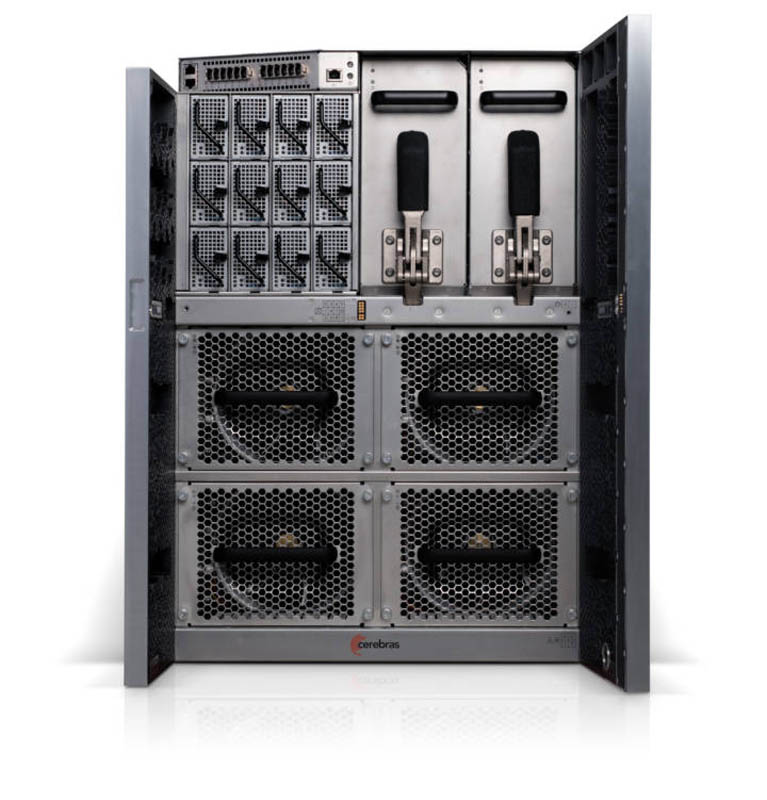
Cerebras need to get power, data, and cooling to the giant chip while also managing things like thermal expansion across a relatively huge area. This is another big engineering win for the company. Inside, the chip is liquid-cooled, and the heat can either be removed via fans or via facility water. We showed how this worked in our Cerebras CS-2 Engine Block Bare on the SC22 Show Floor piece. Here is a video featuring this.
This system and its new chip is a roughly 2x performance leap at the same power and price. Cerebras gets a big advantage from each process step starting with 16nm in the first generation to 5nm today.
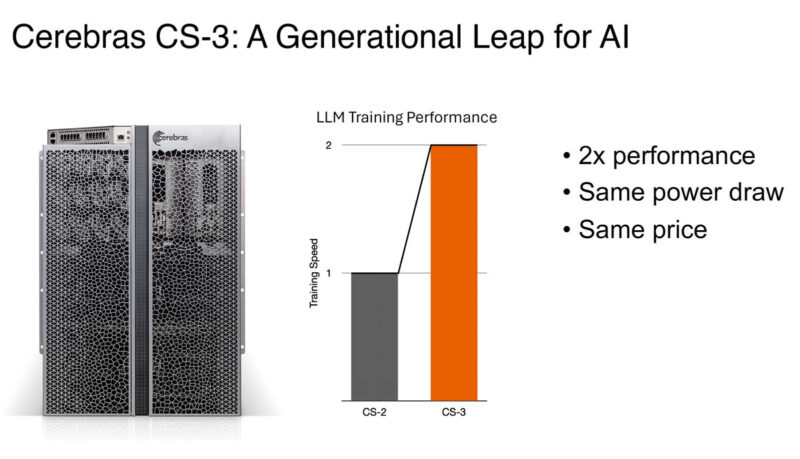
Compared to the NVIDIA DGX H100 system with eight NVIDIA H100 GPUs and internal NVSwitch and PCIe switches it is just a bigger building block.
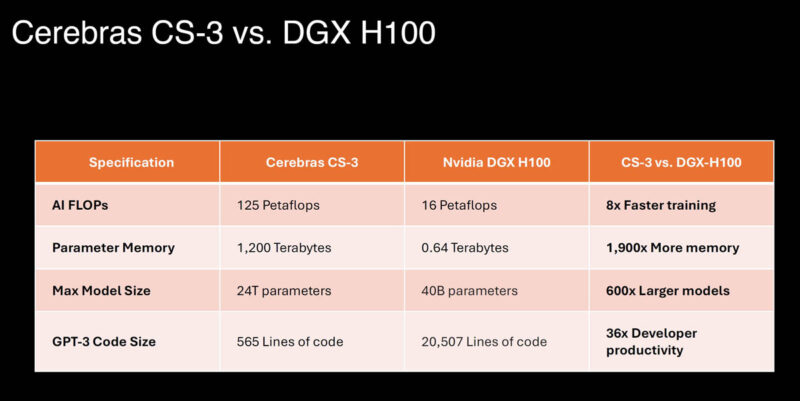
Here is a CS-3 with Supermicro 1U servers.
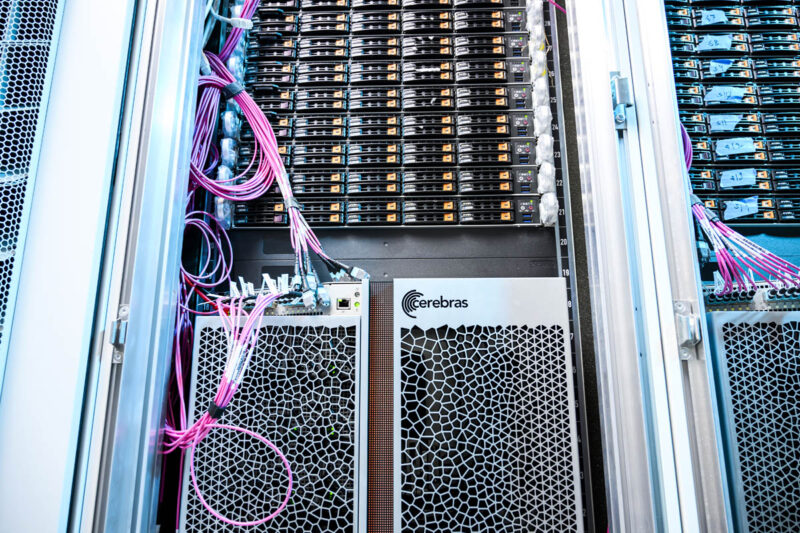
Here is another Cerebras cluster shot with Supermciro 1U servers. Cerebras generally uses AMD EPYC for higher core counts, but perhaps also because a lot of the Cerebras team came from SeaMicro, which was acquired by AMD.
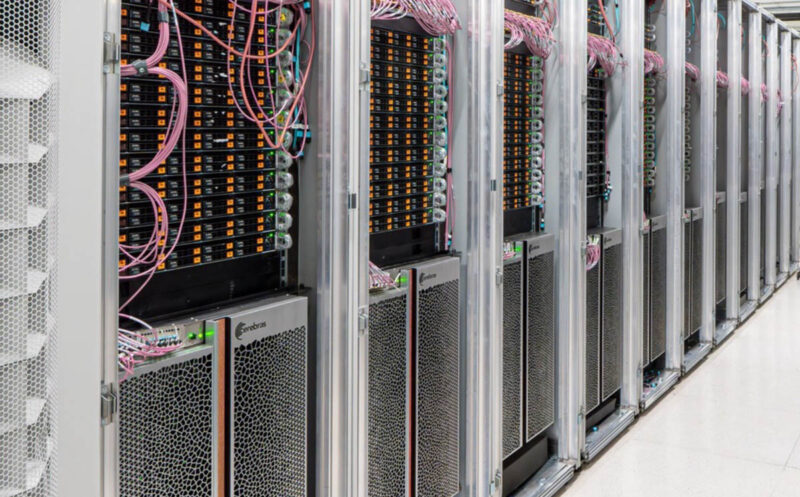
Something we noticed in this iteration is that Cerebras also has a solution with HPE servers. It is a bit strange since, generally, the Supermicro BigTwin is a step ahead of HPE’s 2U 4-node offerings.
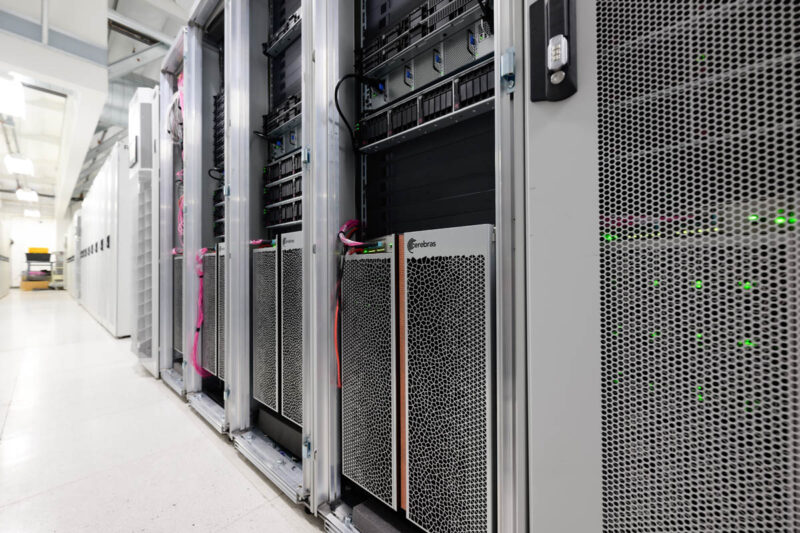
One way to think of the Cerebras CS-2/ CS-3 is that they are giant compute machines, but a lot of the data pre-processing, cluster-level tasks, and so forth happens on traditional x86 compute to feed the optimized AI chip.
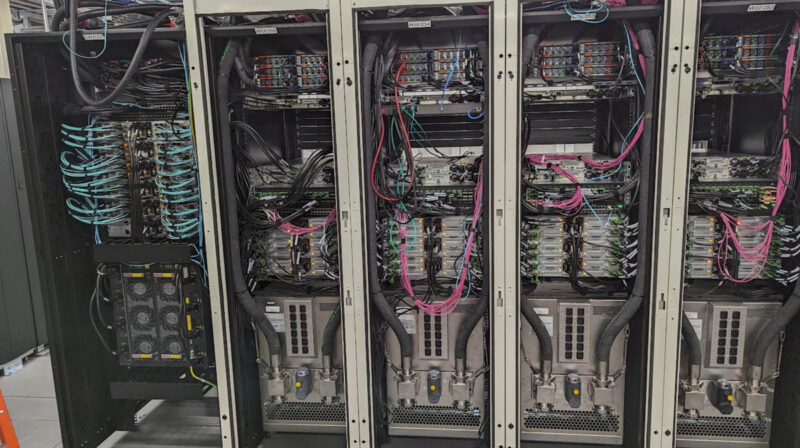
Since this is in a liquid-cooled data center, the air-cooled HPE servers have a rear door heat exchanger setup from ColdLogik, a subbrand of Legrand. If you want to learn more about rear door heat exchangers compared to other liquid cooling options, you can see our Liquid Cooling Next-Gen Servers Getting Hands-on with 3 Options from 2021.
This is a great example of how Cerebras could utilize a liquid-cooled facility, but it did not have to bring cold plates to each server node.
One of the big features of this generation is even larger clusters, up to 2048 CS-3’s for up to 256 exaFLOPs of AI compute.
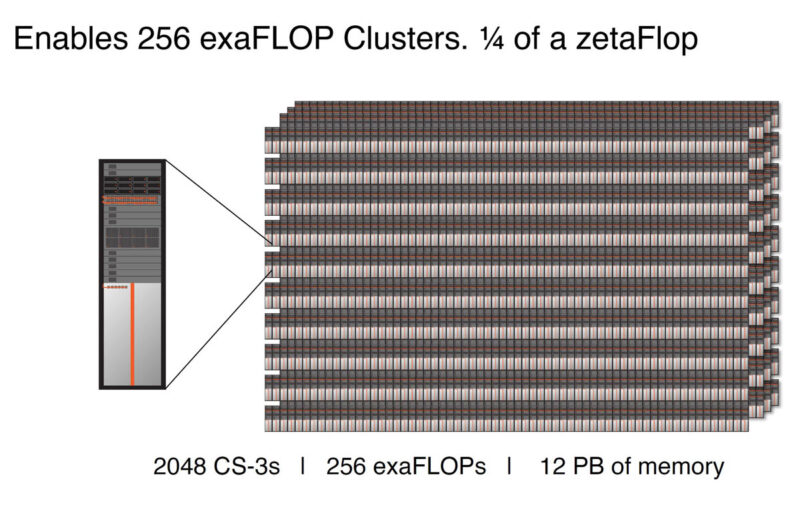
That 12PB memory figure is a top-end hyper-scale SKU designed for training GPT-5 size models quickly. Cerebras can also scale down to something like a single CS-2 with supporting servers and networking.
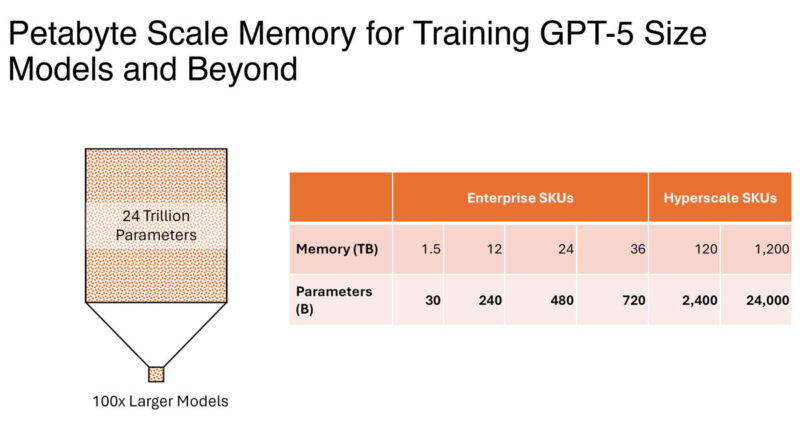
Part of the memory is not just the on-chip memory (44GB) but also the memory in the supporting servers.
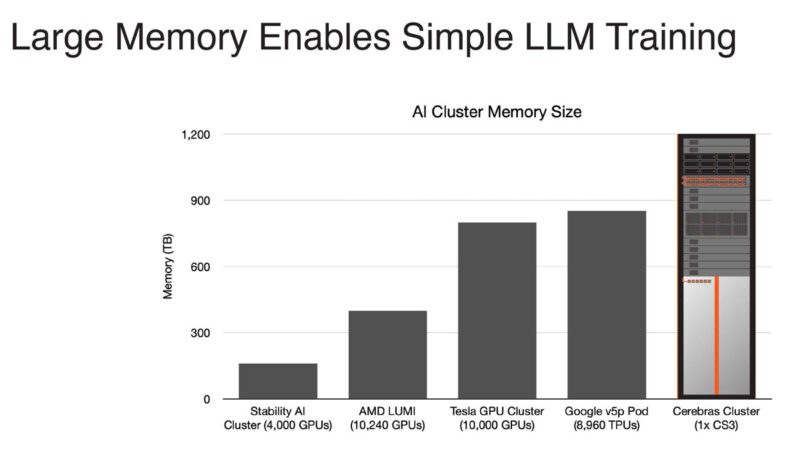
As a result, the Cerebras cluster can train much larger models than before.
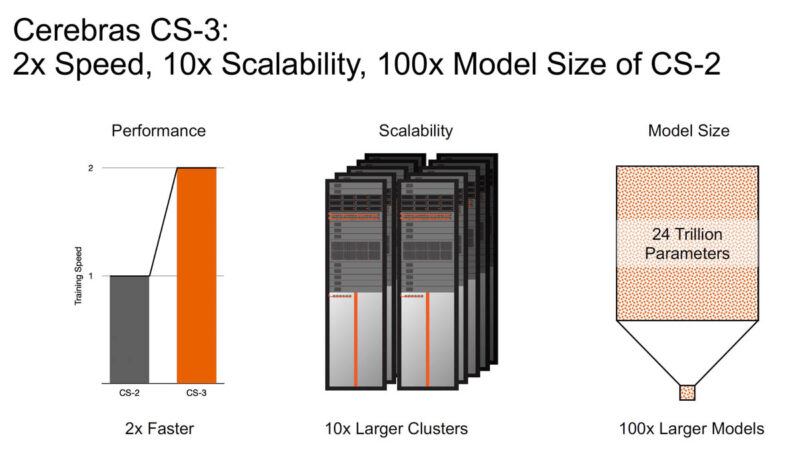
Earlier, we said that Cerebras was a commercial success, and there was an update on that as well.
Cerebras Condor Galaxy Update
A few months ago, we covered how the first $100M+ Cerebras AI Cluster makes it the Post-Legacy Silicon AI Winner. We also discussed the Giant Cerebras Wafer-Scale Cluster in more detail. Not only did the Condor Galaxy 1 get an announcement, but it is completed and is training for customers.
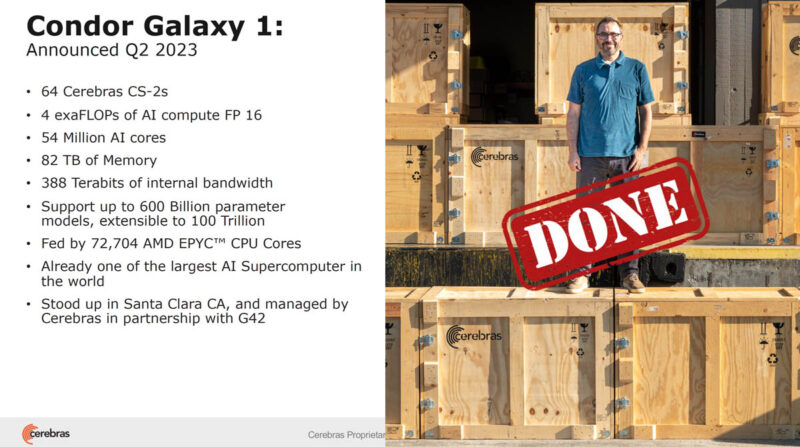
Cerebras did not just announce one, there is another cluster, the Condor Galaxy 2 that is now up and running for G42.
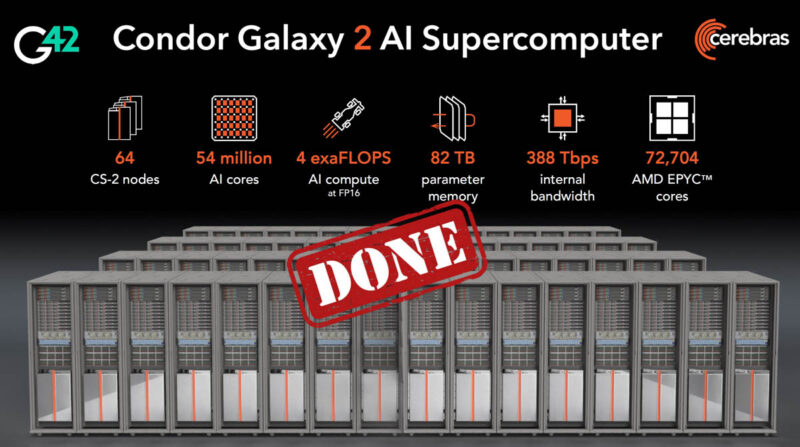
The new Condor Galaxy 3 is the Dallas (good luck with Texas property tax on these!) cluster that will use the new 5nm WSE-3 and CS-3 for compute.
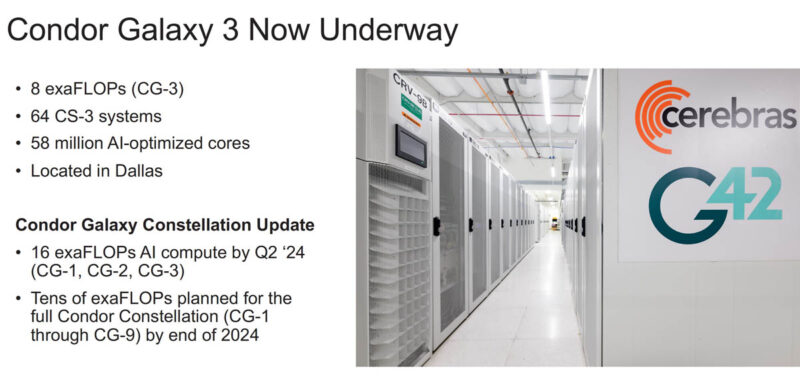
These are the current US-based clusters in Santa Clara, Stockton, and Dallas, but the plan is to build at least six more.
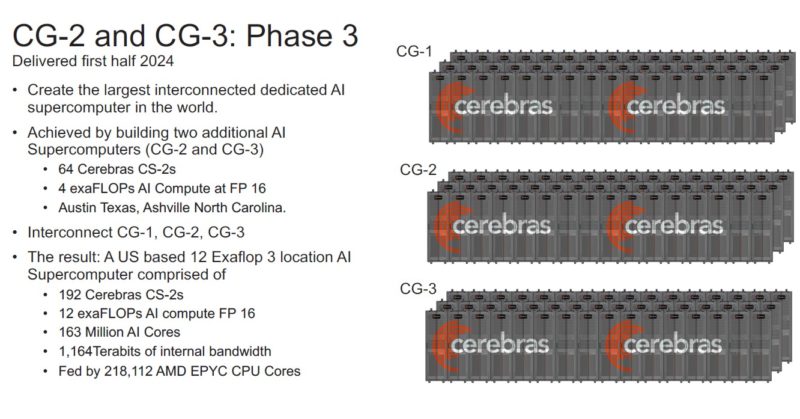
The total value of these clusters should be north of $1B and be completed in 2024. Aside from the $1B in deal value, Cerebras told us that they are currently supply-limited, so the demand is there for the WSE-3.
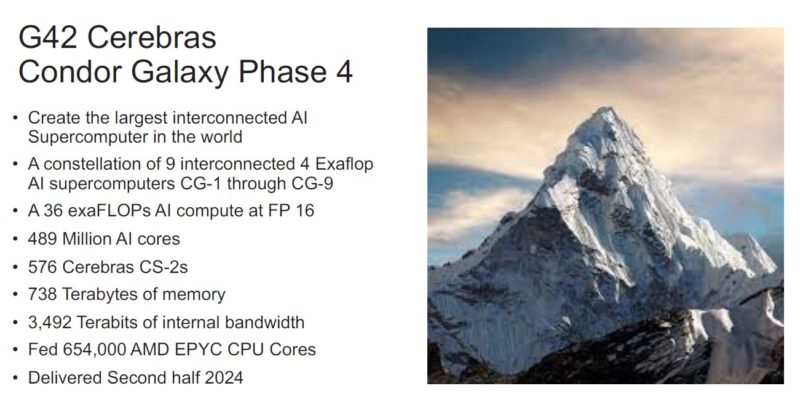
Since we know customers are using these, the question is how hard is it to use?
Cerebras Programming for AI
You may have seen that Cerebras says its platform is easier to use than NVIDIA’s. One big reason for this is how Cerebras stores weights and activations, and it does not have to scale to multiple GPUs in a system and then multiple GPU servers across a cluster.
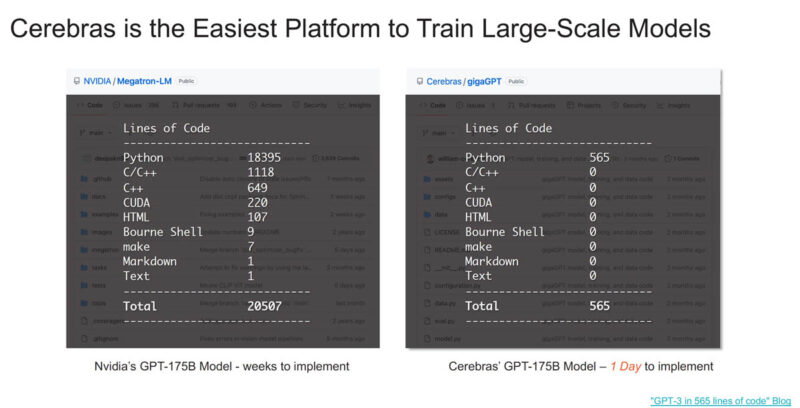
Aside from the code changes being easy, Cerebras says that it can train faster than a Meta GPU cluster. Of course, this seems to be a theoretical Cerebras CS-3 cluster at this point since we did not hear of any 2048 CS-3 clusters up and running while Meta has AI GPU clusters already.
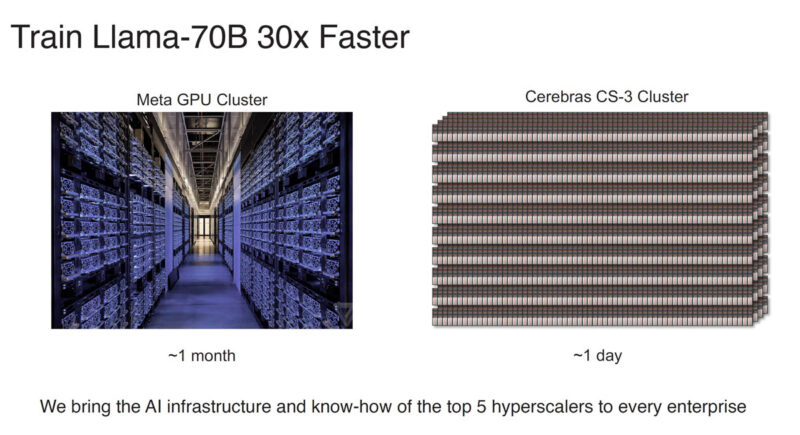
Overall, there is a lot going on here, but one thing we know is that more people are using NVIDIA-based clusters today.
Cerebras and… Qualcomm?
While Cerebras is focused on training in inference, it announced a partnership with Qualcomm to use Qualcomm’s legacy AI inference accelerators. We first covered the Qualcomm Cloud AI 100 AI Inference Card at Hot Chips 33 in 2021.
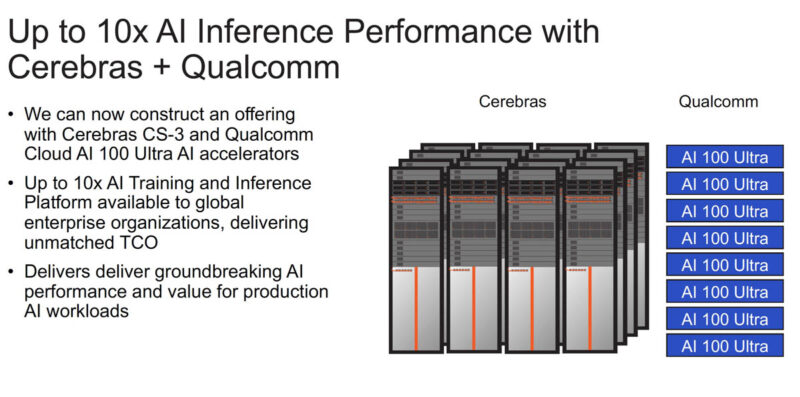
The idea is to have a GPU-free training and inference platform, but it just left us wondering if Qualcomm invested in Cerebras. It felt like that kind of add-on announcement.
Final Words
The Cerebras Wafer Scale Engine series is still a fantastic bit of engineering. A big part of the announcement is that the 5nm WSE-3 is out. One of the cool things is that Cerebras gets a huge bump from process advancements.
We know that the AMD MI300X will easily pass $1B in revenue this year. Cerebras looks on track to pass $1B of revenue, assuming it is selling the entire cluster, not just the multi-million dollar CS-3 boxes. NVIDIA will sell $1B of hardware next week at GTC when it talks more about the NVIDIA H200 and the next-generation NVIDIA B100. Intel’s Gaudi3 we will get an update on, but we have heard a few folks share nine-figure sales forecasts for 2024. Cerebras is perhaps the only company focusing on training that is playing with the larger chipmakers in terms of revenue.
Hopefully we get WSE-4 in the second half of 2025 for another big jump!
that’s big
I’d be curious to see the yeild factor of these chips
A lot about the hardware, nothing about the software.
Let’s see the tooling and real life benchmarks.
@Flippo
There is a Cerebras Developer Community Meeting videos on YouTube
where real performance and development environment of those devices is presented.