To say that the Cerebras Wafer Scale Engine caused a stir when it was announced is likely an understatement. While others in the industry were packing as many chips onto wafters as they could, Cerebras went in the other direction: making the wafer a big chip. The first generation product was a 16nm part but now Cerebras has a TSMC 7nm Wafer Scale Engine-2 (WSE-2) and system (CS-2) that pack more than twice the compute and memory per wafer.
WSE-2 and CS-2 Comparisons
In terms of the move from the WSE-1 to WSE-2, here is what some of the key specs are:
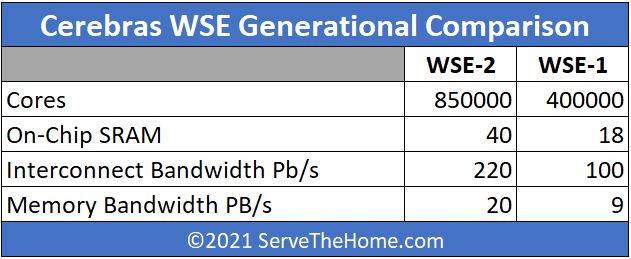
In rough terms, these numbers are in the 2.1-2.2x generation/ generation increase. Using the wafer as effectively a cluster-on-chip means that Cerebras is scaling core counts, memory, and bandwidth in relative unison.
Cerebras has this comparison of its new WSE-2 chip versus the NVIDIA A100:
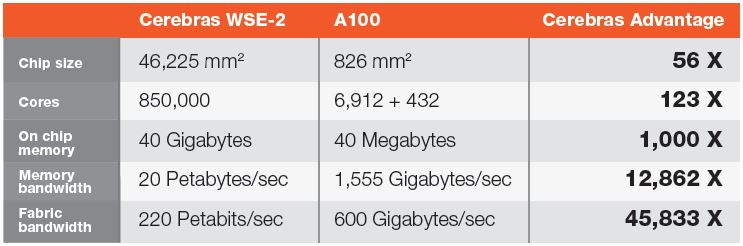
This is only part of the story though. The CS-2 uses up to 23kW. That is around the same as 56 NVIDIA A100 SXM4 40GB 400W modules or 46 NVIDIA A100 SXM4 500W modules, but without the supporting infrastructure on the NVIDIA side. Using a HGX A100/ DGX A100 for every eight GPUs one also needs two CPUs, RAM, PCIe switches, often NVSwitches. Then, there are the Infiniband interconnects as well. A big part of the Cerebras value proposition is the ability to increase the ratio of compute to external infrastructure.
What is somewhat interesting is that Cerebras is scaling its design basically taking advantage of process improvements. That is a different model than NVIDIA constantly adding new features to its GPUs and taking advantage of process improvements.
The Cerebras CS-2 System Wrapper
In terms of the CS-2 system, it looks largely the same as the CS-1 system we saw previously. We still have 12x 100GbE external links. It is interesting that Cerebras did not scale that aspect of the system. However, we still have a photo of the old Los Altos, California lab where the company was assembling a three CS-1 rack for a facility liquid-cooled supercomputing customer. One of the key challenges of putting a cluster in a rack is that power density increases significantly.
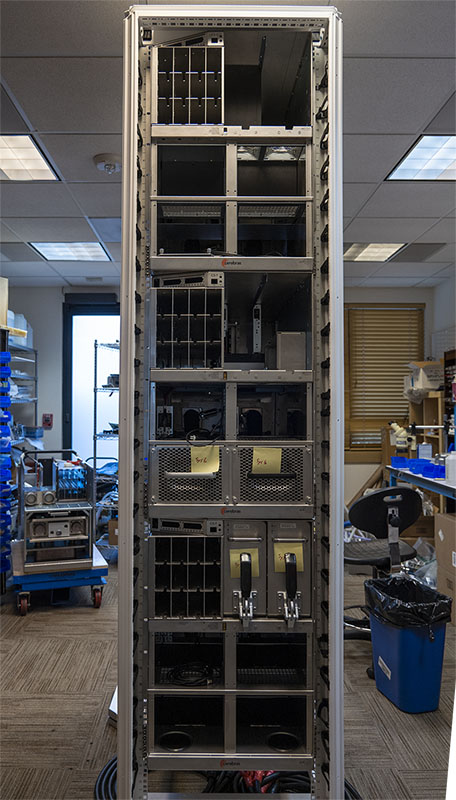
We have a discussion around the CS-1 and how it is internally liquid-cooled in Cerebras CS-1 Wafer-Scale AI System at SC19.
Final Words
If you want to learn more about the system and software that makes this work, Cerebras has a great whitepaper on the new CS-2, WSE-2, and how it looks to integrate the system into existing deep learning workflows. You can also see Our Interview with Andrew Feldman CEO of Cerebras Systems to learn more about the company’s approach.
While WSE-2/CS-2 looks like an outstanding piece of hardware for AI/ML, table above is highly misleading as it’s (likely intentionally) mixing up on-chip and off-chip memory size (which I think is 0 for WSE-2) and on-chip and off-chip memory bandwidth (as on-chip memory bandwidth is much higher than 1.55 or 2TB/sec for A100).
It’s not even clear whom they’re trying to mislead as people who can afford CS-2 almost certainly understand such differences.
Something isn’t right with this statement. “… 56 NVIDIA A100 SXM4 40GB 400W modules or 46 NVIDIA A100 SXM4 500W modules …” as the GPU appear identical… except for the 40GB reference…
Dan, the difference is the power envelope. They’re comparing how much you can get for the same power the WSE-2 consumes.