It is easy to say that NVIDIA Blackwell will sell like hotcakes in 2025. The company went into the platform architecture a bit more at Hot Chips 2024. Blackwell is something a lot of folks are excited about in the industry. As a quick note, for some reason NVIDIA had strange slides, so there is a lot of white space when doing this quickly. This was the one strange PDF of the over a dozen posted today. Sorry for that. On the plus side, NVIDIA showed its latest data center roadmap.
Please note that we are doing these live at Hot Chips 2024 this week, so please excuse typos.
NVIDIA Blackwell Platform at Hot Chips 2024
NVIDIA is not talking about the individual GPU as much as it is talking about the cluster level for AI. That makes a lot of sense especially if you see talks from large AI shops like the OpenAI Keynote on Building Scalable AI Infrastructure at Hot Chips 2024.
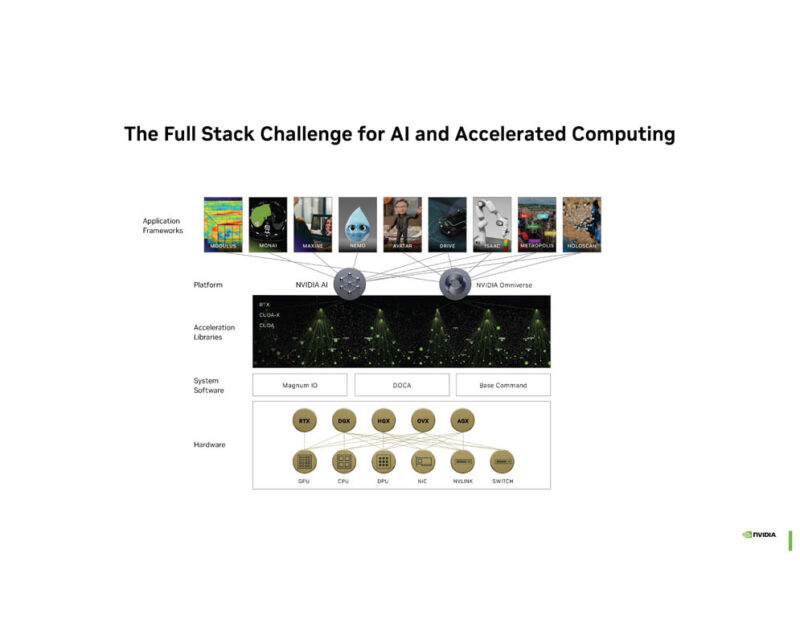
NVIDIA does not just focus on building the hardware cluster, but it also the software with optimized libraries.
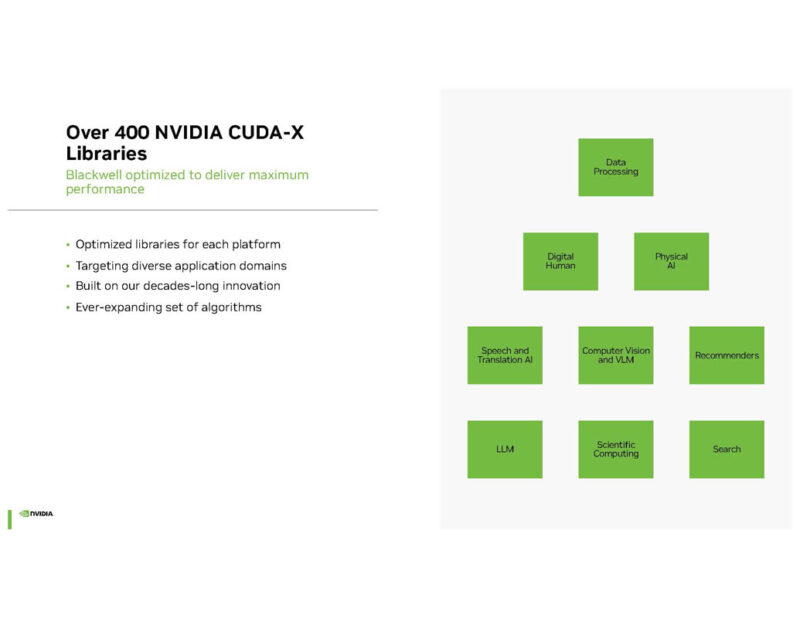
The NVIDIA Blackwell platform spans from the CPU and GPU compute, to the different types of networks used for interconnects. This is chips to racks and interconnects, not just a GPU.
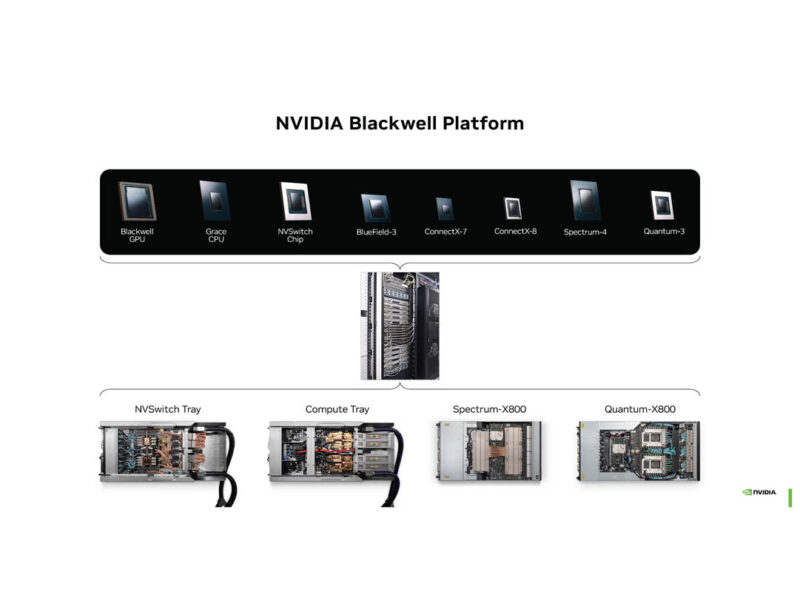
We did a fairly in-depth look at Blackwell during the NVIDIA GTC 2024 Keynote earlier this year.
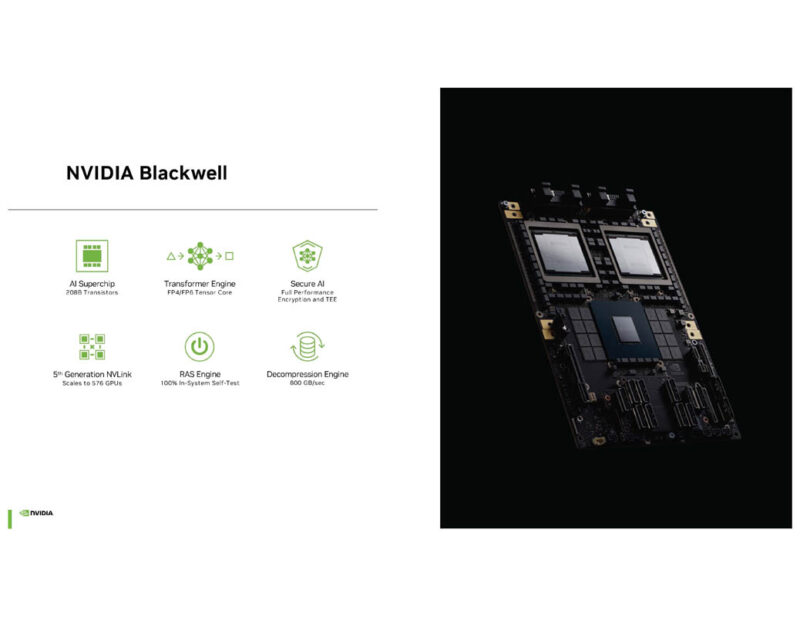
The GPU is huge. One of the big features is the NVLink-C2C to the Grace CPU.
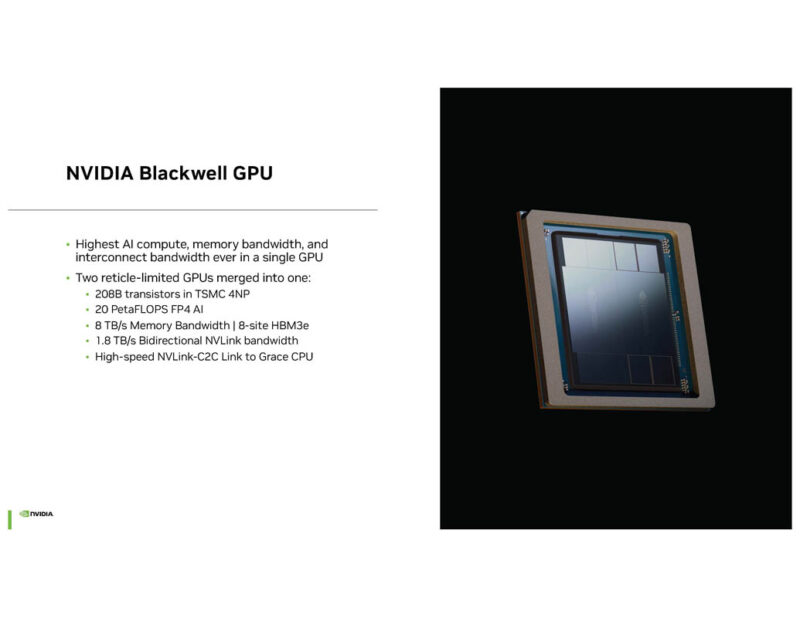
As NVIDIA’s newest, GPU is also its highest performance one.
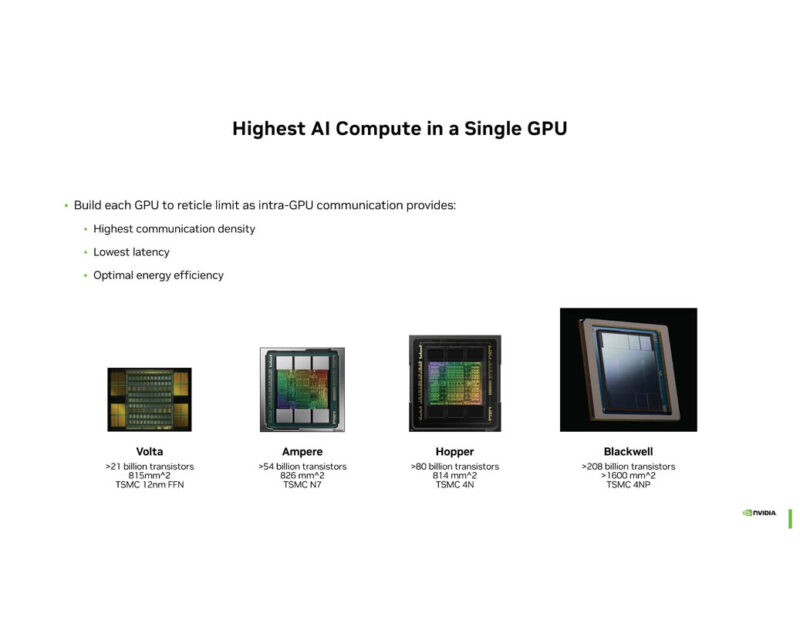
NVIDIA uses the NVIDIA High-Bandwidth Interface (NV-HBI) to provide 10TB/s of bandwidth between the two GPU dies.
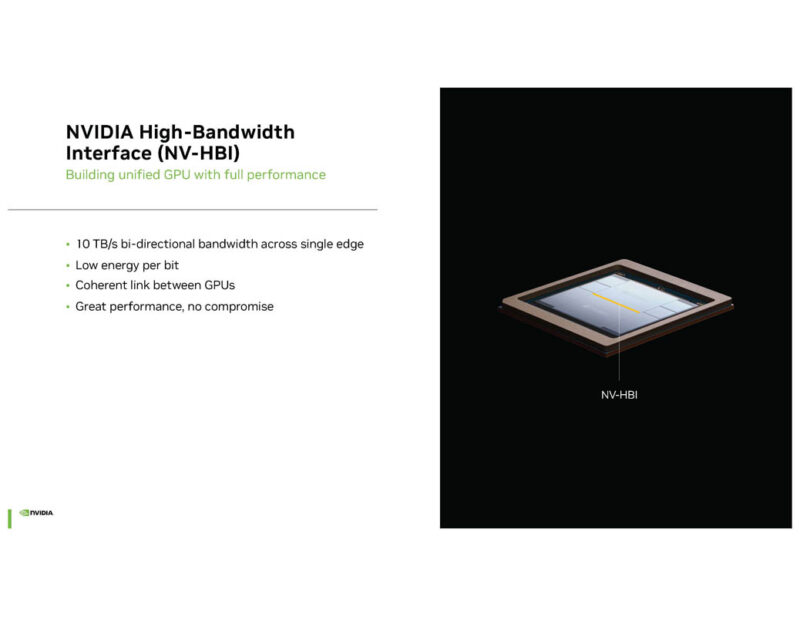
The NVIDIA GB200 Superchip is the NVIDIA Grace CPU and two NVIDIA Blackwell GPUs in a half-width platform. Two of these side-by-side means that each compute tray has four GPUs and two Arm CPUs.
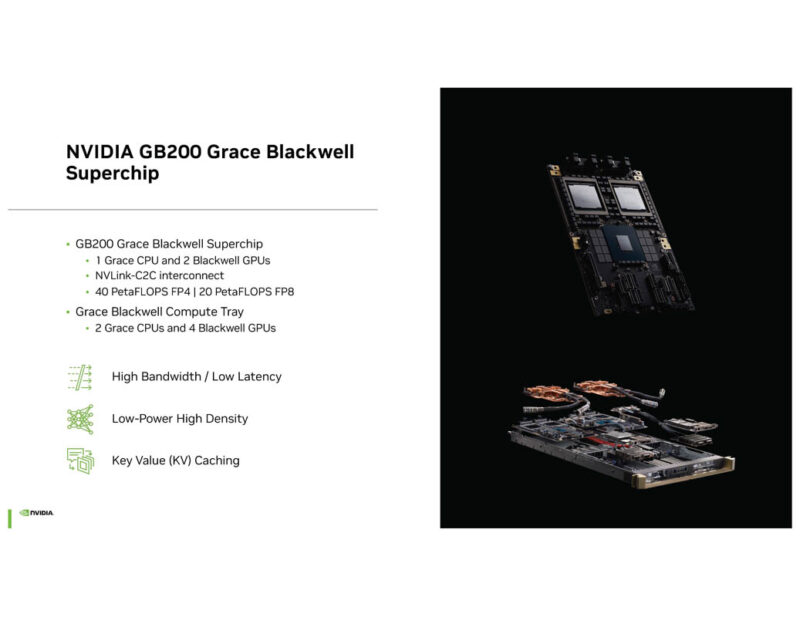
NVIDIA has new FP4 and FP6 precision. Lowering the precision of compute is a well-known way to increase performance.
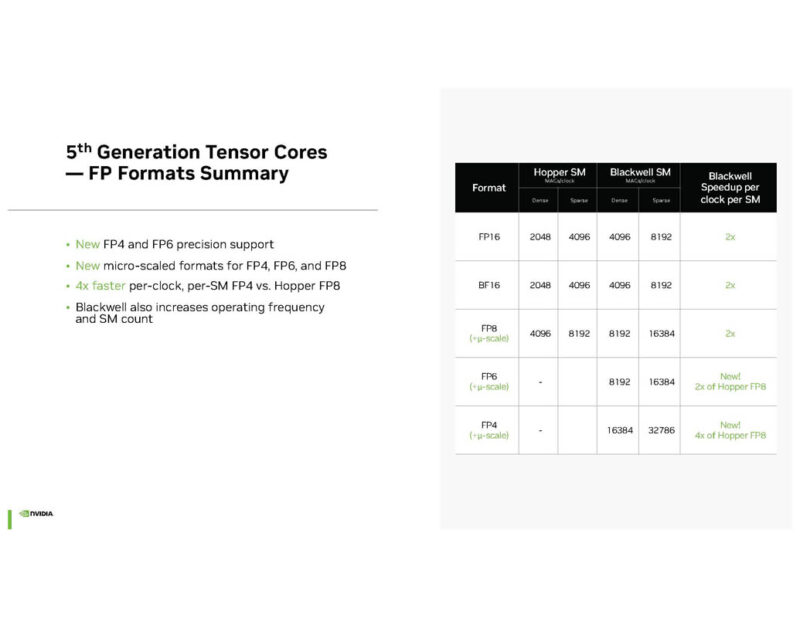
NVIDIA Quasar Quantization is used to figure out what can use lower precision, and therefore less compute and storage.
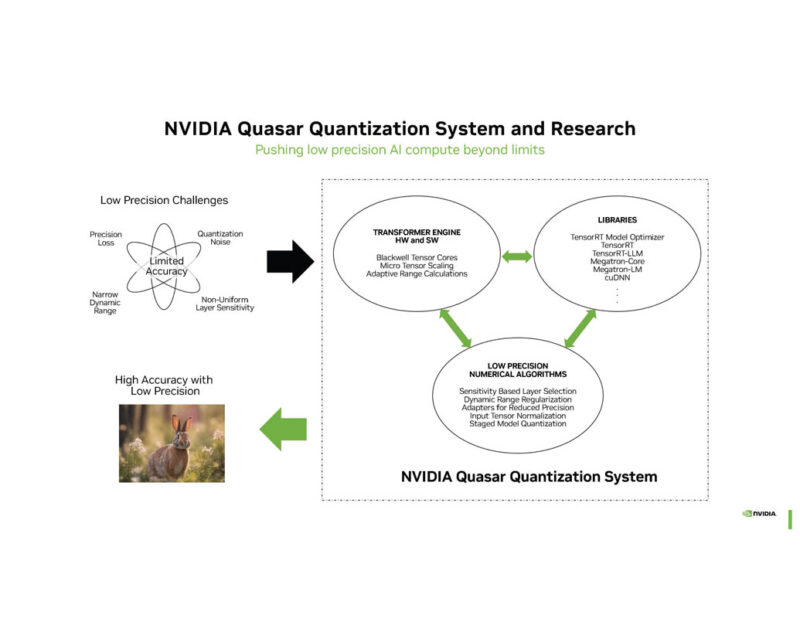
FP4 for Inference NVIDIA says can get close to BF16 performance in some cases.
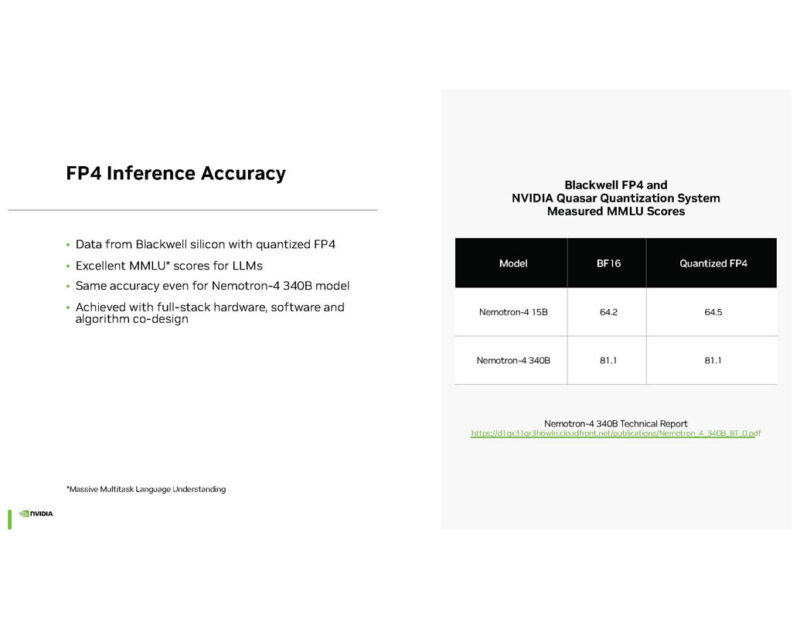
Here is an image generation task using FP16 inference and FP4. These rabbits are not the same, but they are fairly close at a quick glance.
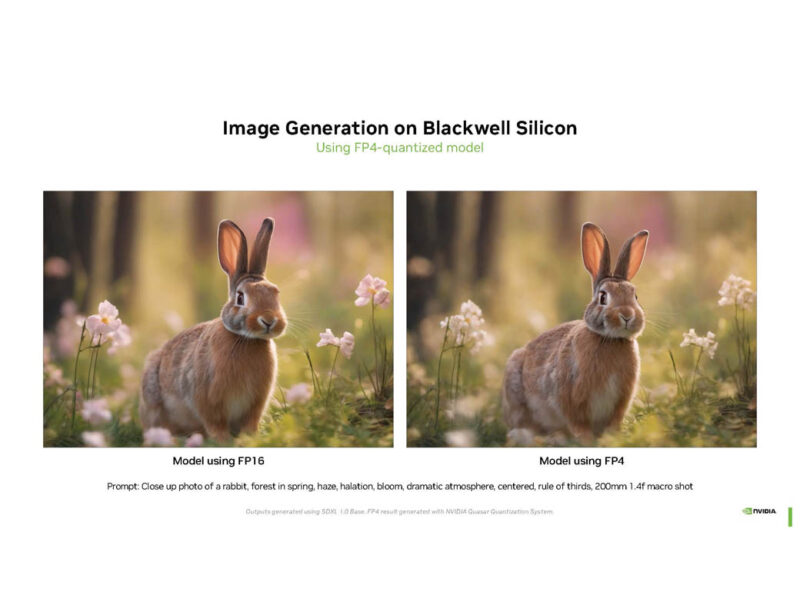
NVIDIA says AI models are growing.
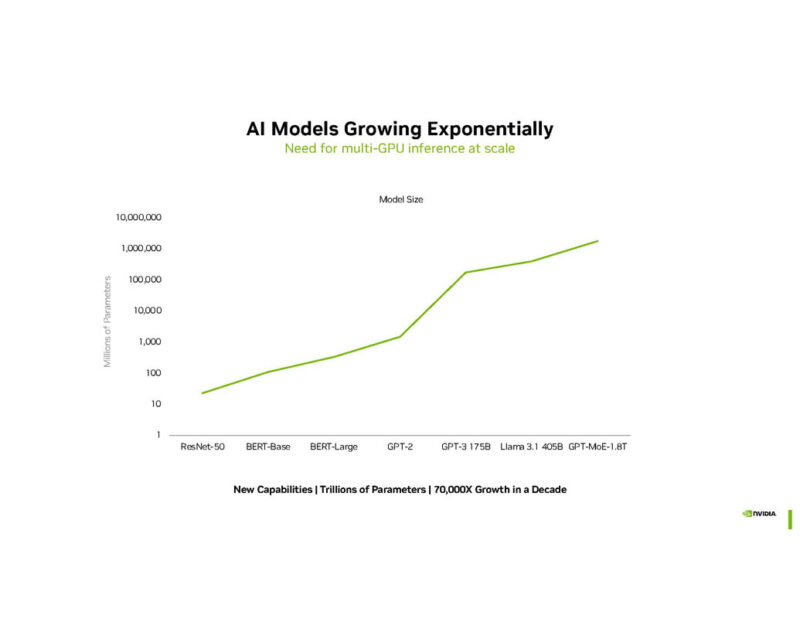
The PHY has become a big deal because part of NVIDIA’s secret sauce is being able to ship data around different parts of systems over NVLink more efficiently than with other technologies.
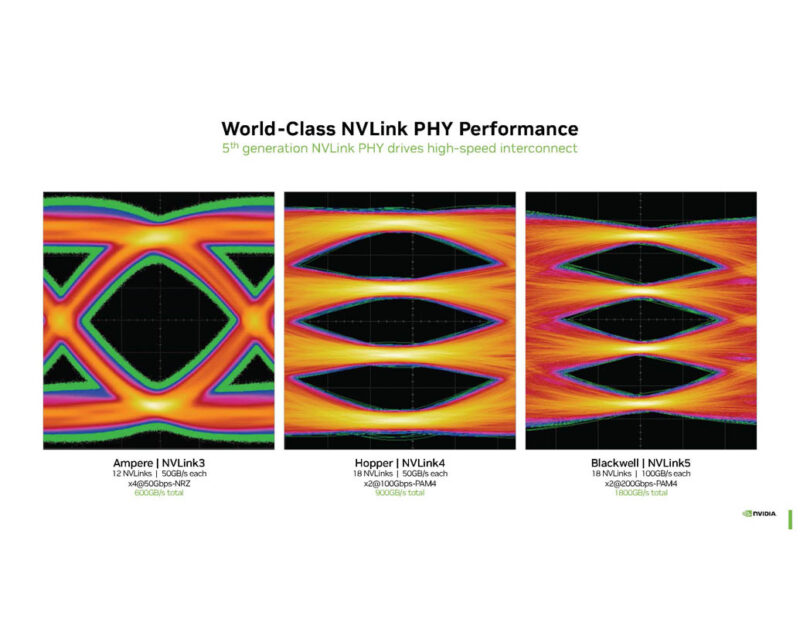
The NVLink Switch Chip and NVLink switch tray are designed to push a ton of data at lower power than simply using an off-the-shelf solution like Ethernet.
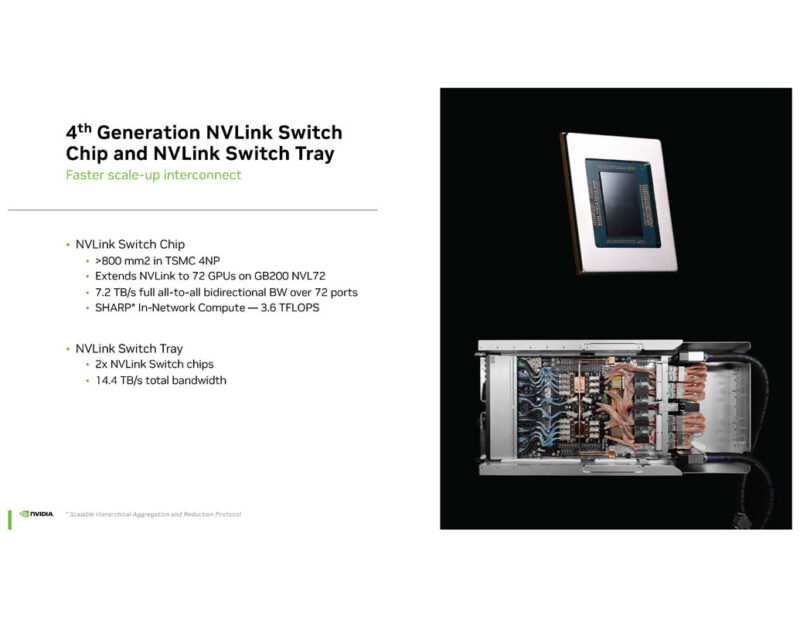
NVLink has done this from 2016 with eight GPUs to 72 GPUs in the current generation. Conveniently, the NVIDIA NVSwitch Details at Hot Chips 30 talk that went into the 16-GPU NVSwitch DGX-2 topology was left out.
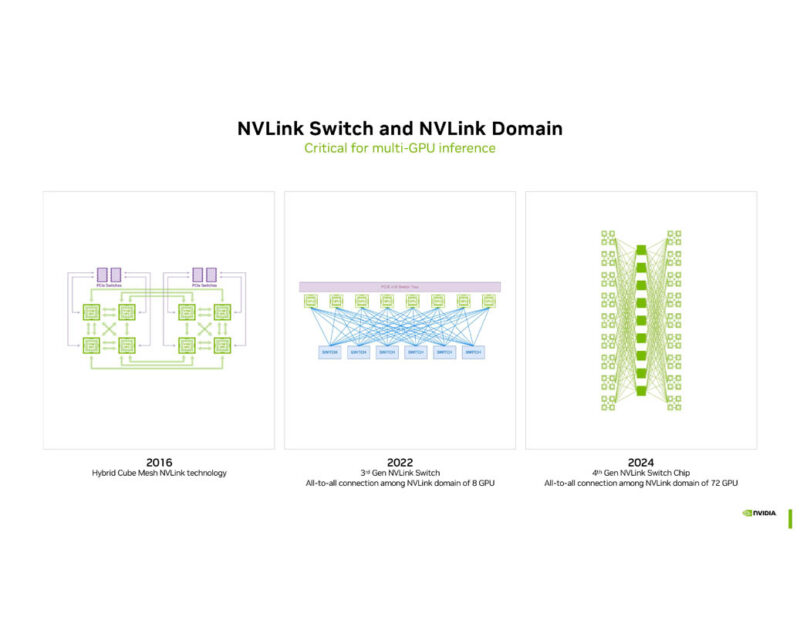
NVIDIA is showing the GB200 NVL72 and NVL36. The NVL36 is the 36 GPU version for those data centers that cannot handle 120kW racks.
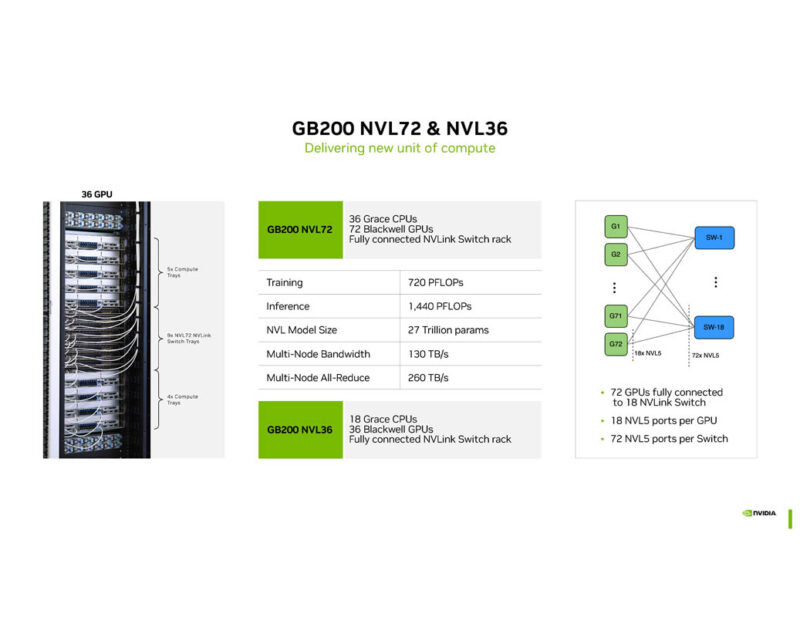
With Spectrum-X, Spectrum-4 (similar to the Marvell Teralynx 10 a 51.2T Ethernet switch) plus BlueField-3 yield a combined solution for RDMA networking over Ethernet. In a way, NVIDIA is already doing some of the things that the UltraEthernet Consortium will introduce in future generations.
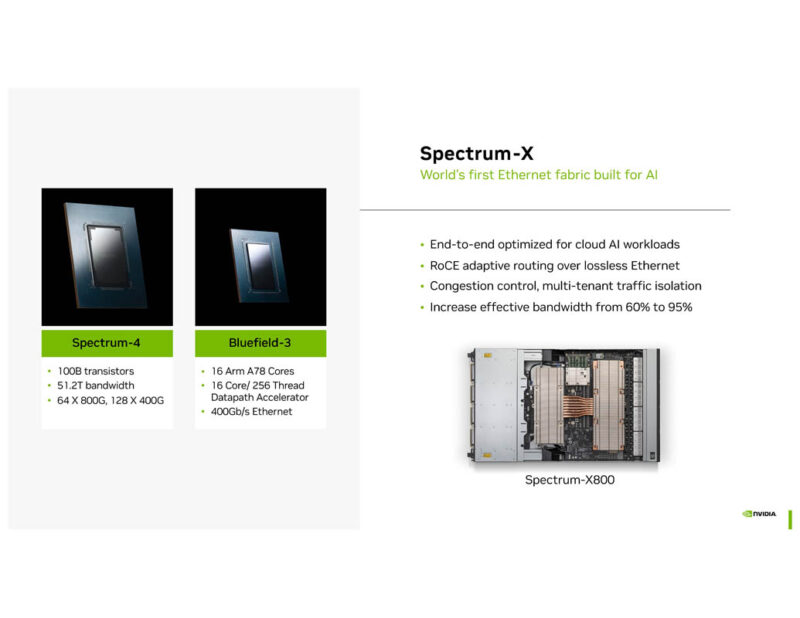
The GB200 NVL72 is designed for the trillion parameter AI.
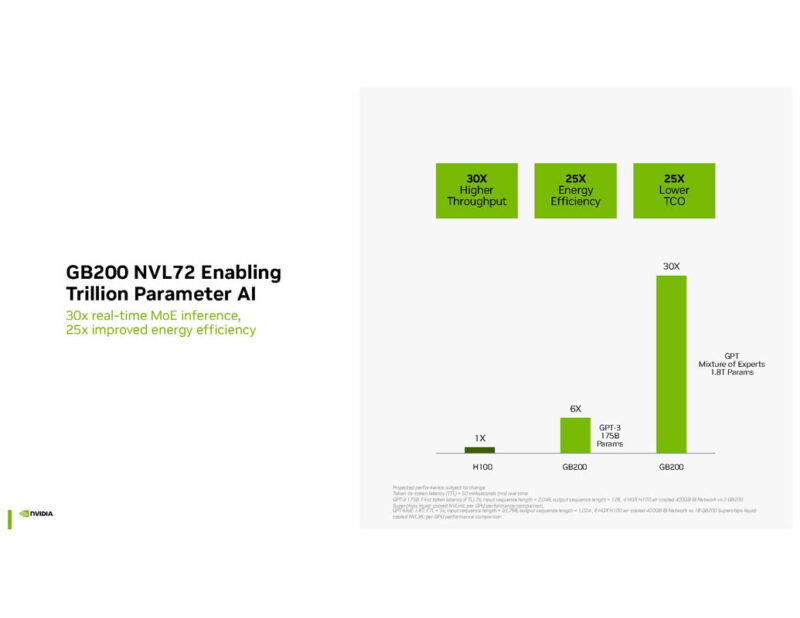
With increasing model sizes, splitting workloads across multiple GPUs is imperative.
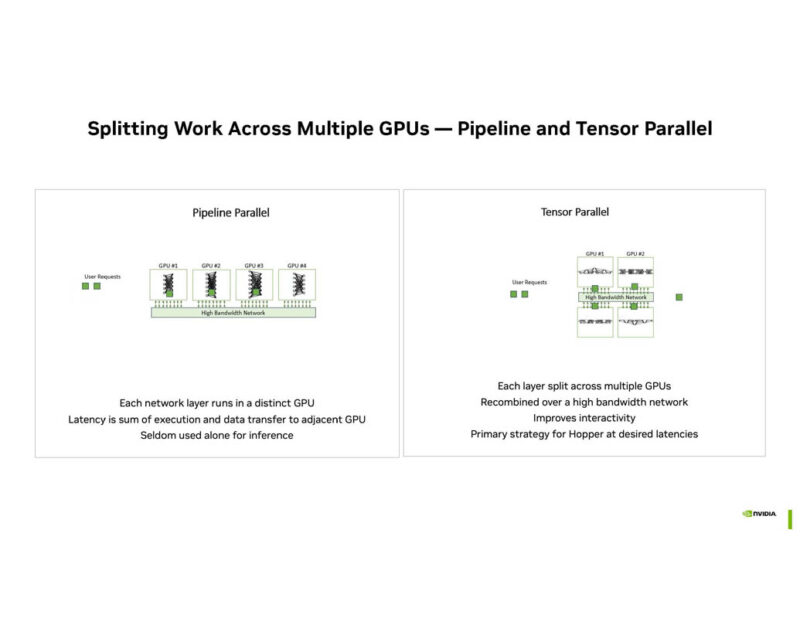
Blackwell is big enough to handle expert models in one GPU.
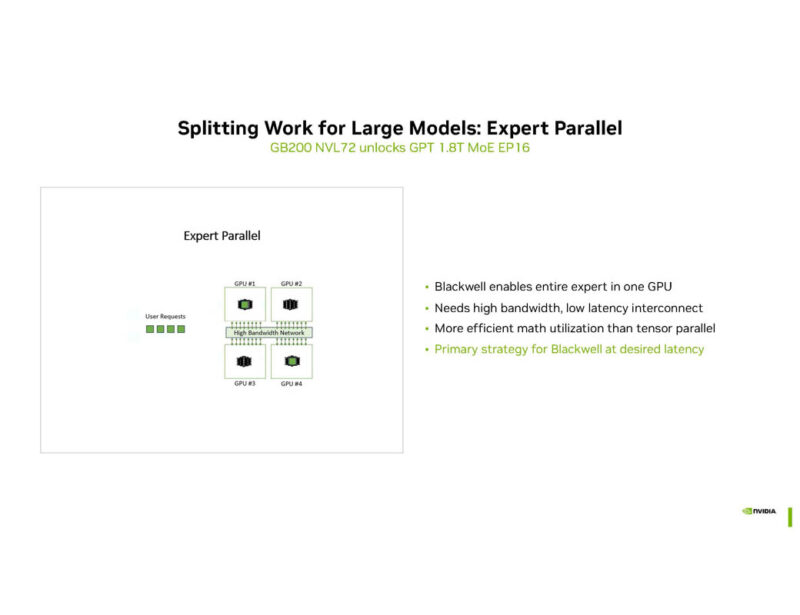
NVIDIA is showing the GPT-MoE 1.8T performance.
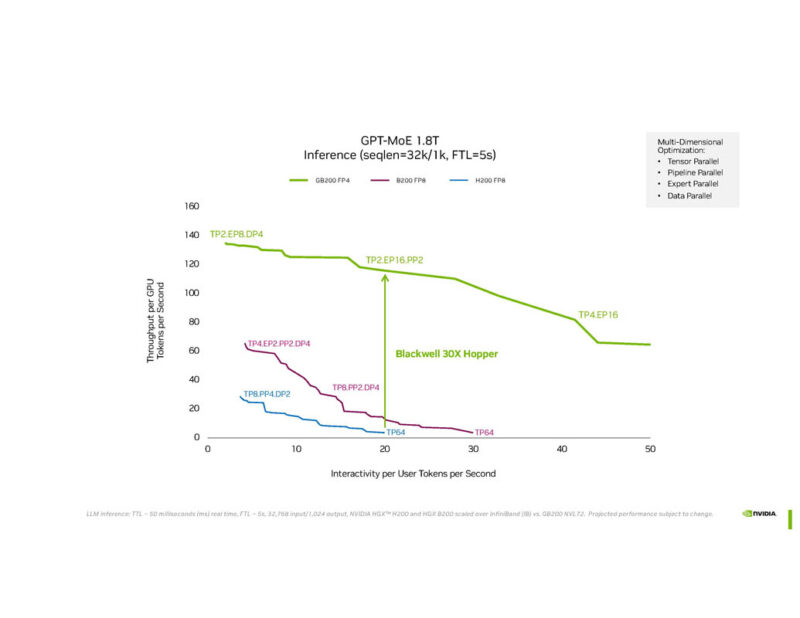
Here is the new NVIDIA Roadmap slide. With 1.6T ConnectX-9 in 2026, that means that NVIDIA seems to be pointing to the need for PCIe Gen7 since PCIe Gen6 x16 cannot handle 1.6T network connections. Perhaps one could use multi-host, but this is exciting.
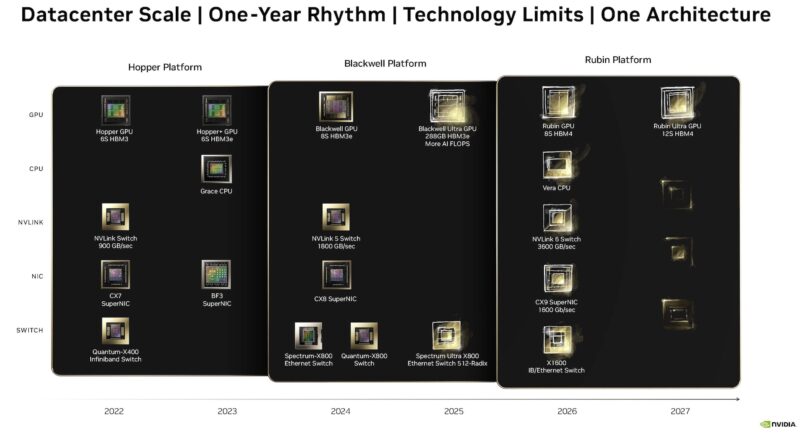
Here is the quick summary.
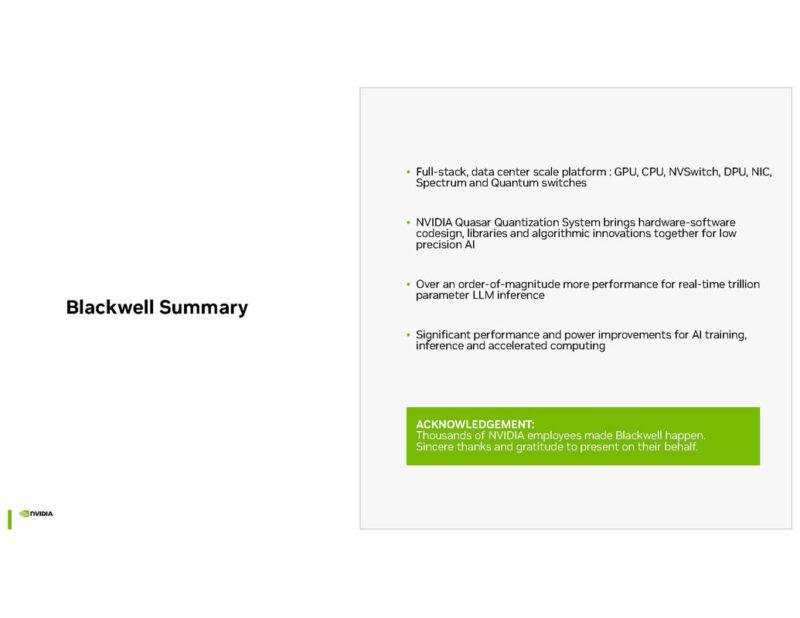
Always good, but hopefully, we see more Blackwell arrive this year.
Final Words
A lot of this we have seen before, save perhaps the roadmap slide. What is somewhat interesting is that we are sitting in a conference where there are a lot of AI accelerators. At the same time, NVIDIA is not just building clusters, but it is also optimizing everything, including the interconnects, switch chips, and even the deployment models. A challenge AI startups have is that NVIDIA is not just making today’s chips, switches, NICs, and more. Instead, it is doing frontier research so that its next-generation products meet the needs of future models at a cluster level. That is a big difference.