At ISC 2022 I had the opportunity to sit down with Andy Hock at Cerebras. This was very cool after I told Cerebras and HPE that STH would not cover the HPE Superdome plus one Cerebras CS-2 being installed at LRZ for ISC, Andy still agreed to meet. As a result, we get a little article about the experience. This was one of the more interesting conversations since we veered fairly far from the Cerebras product. Instead, what I wanted to do was just give a perspective, reinforced by this discussion, on what Cerebras is doing to differentiate itself against a huge competitor (NVIDIA.)
Cerebras HPC Acceleration ISC 2022
Generally, the LRZ announcement was about combining a HPE Superdome with a Cerebras CS-2 box. That may seem to be a small installation, but given the prices of the components, support, and such, it may end up being a few million-dollar “cluster” of two machines. Even though it is two machines physically, because the Cerebras WSE-2 never goes through the step of splitting the silicon into smaller chips, then packaging those chips, putting them into multiple servers, networking them together, and creating a cluster of well over a dozen machines. We need to treat each CS-2 as a mini-cluster itself.
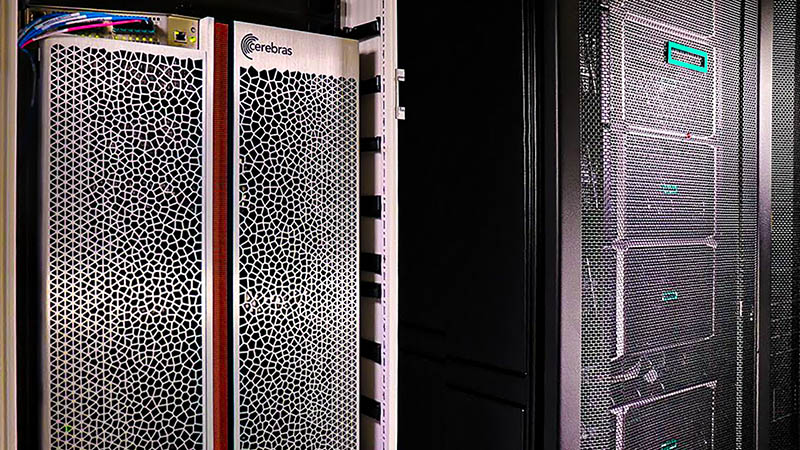
Something that Cerebras is working on, and why the LRZ announcement will be more impactful, is that the company is looking beyond AI acceleration. Some of the first areas are biomedical and life sciences. The SDK for this is apparently out there, but it is in private beta.
One of the key things that Cerebras is doing is flipping the usage of its chips, or perhaps more accurately, providing an alternative. Traditionally, Cerebras’ model has been to put large models into the on-chip SRAM, then stream in the input data. This worked for smaller models, but for larger models, Cerebras runs into the issue that even with tens of gigabytes of memory, there is nowhere near enough. Instead, The new plan is to put the input data on the SRAM and stream in the model weights to the megachip.
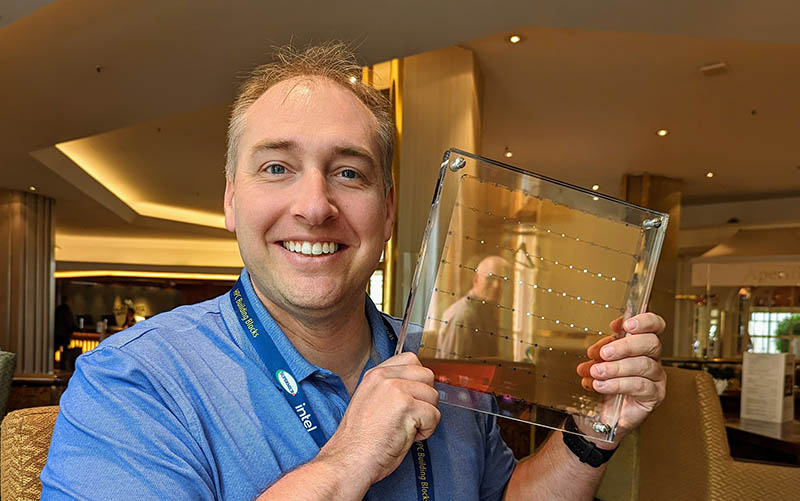
The impact of streaming the weights rather than the inputs is that large models like GPT-3 and newer larger models can be trained using a single CS-2, lowering the cost to get started on larger model sizes.
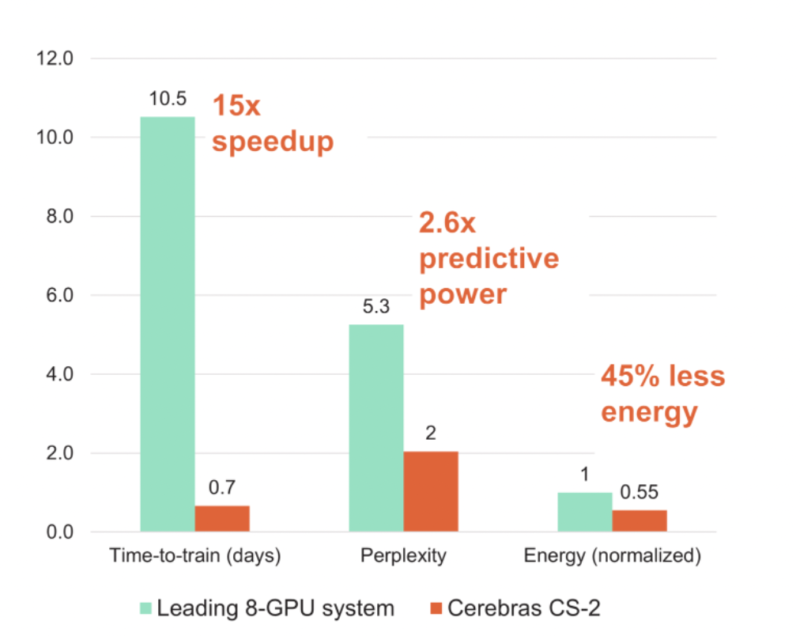
The other side, and one especially important for Europe where ISC 2022 was being held, is energy efficiency. The approach of keeping the entire wafer (or most of it) intact instead of splitting it up and then having to span longer distances with a high-speed interconnect means less power is used to train models.
Final Words
Cerebras is a company that is doing something different. Having a wafer-scale chip is clearly a major engineering achievement. There is sound logic behind its development with the ability to keep data and computation more concentrated. The next step, and a big differentiator between Cerebras and other AI startups, is the ability to leverage the architecture beyond just AI for science applications.
Now the big question is how long until we get WSE-3?
Is the comparison again A100 or H100 ? If against A100 it’s a total failure. If against H100 it’s surprisingly barely competitive. In any case, call me unimpressed…
H100 is not shipping Xpea. These are both the current generation systems in the market.
The energy efficiency is setting this chip apart and gets it commissioned [1].
[1] https://www.iis.fraunhofer.de/en/pr/2021/20210319_ks_energy_saving_AI_chip.html
If my math isn’t wrong to get about the same performance 15 systems based on A100 would consume nearly 27.3 times more power than CS-2. (in this particular use and according to data from Cerebras) Why is that a total failure @Xpea?