MLPerf Tiny v0.5 is out, bringing MLPerf to an even lower-power footprint. MLPerf and ML Commons are the aspiring industry-standard (most vendors do not participate in MLPerf so it is hard to say it is a standard) organization trying to become the SPEC of AI benchmarking. The organization now has a benchmark that goes below the traditional inference space we have covered before to cover a new class of devices. Specifically, this is targeted at the microcontroller market.
MLPerf Tiny v0.5 Launched
With the latest launch, one can see how MLPerf has been expanding from training with large systems in 2018 to 2021’s launch of the lower power inferencing.
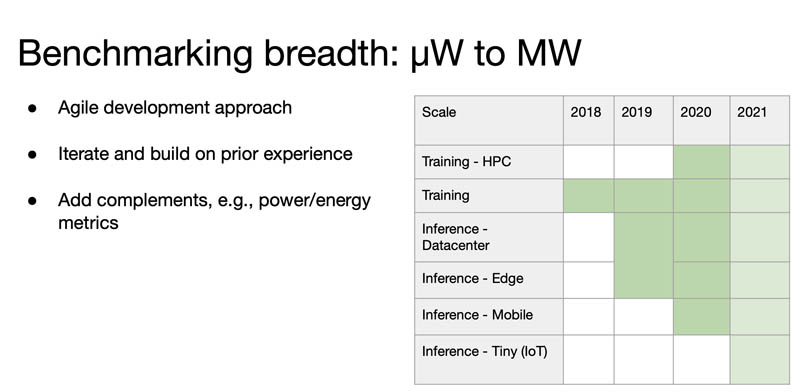
One can see the MLPerf benchmarks, albeit using different suites, are designed to cover a wide range of inference devices.
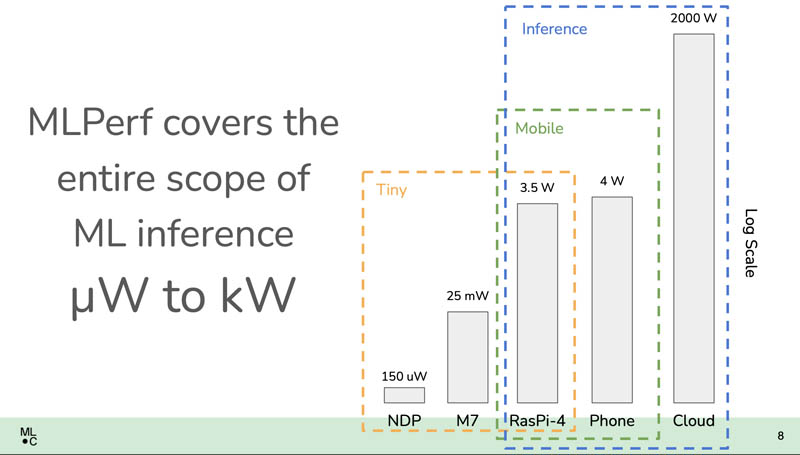
In the microcontroller inferencing market, there are a number of challenges. Usually, there is a sensor or multiple sensor inputs. Then the microcontroller needs to implement AI inferencing to react to the sensor data. Given the limited power envelopes, processing performance, and memory, a lot of work has to go into optimization because there is less capacity than with a NVIDIA T4 for example.
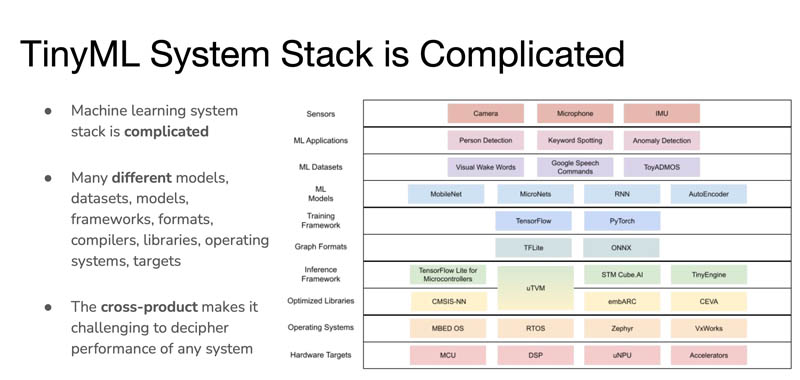
As some examples, these controllers have to wake a device when you say “Alexa…” or “OK Google…” Different types of models are being used depending on the use case and domain.
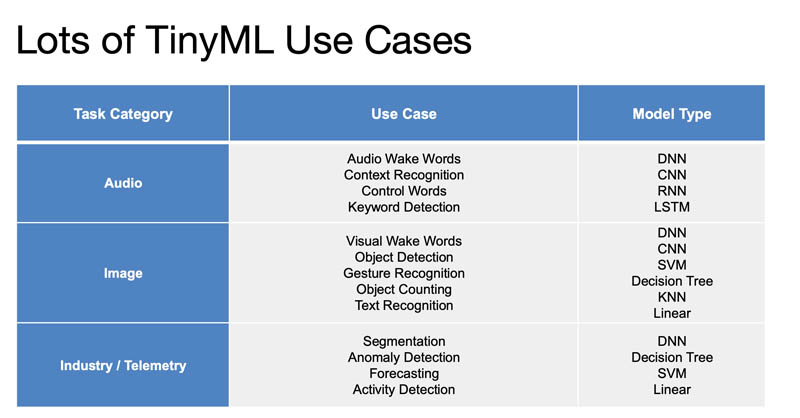
As a result, in Tiny v0.5 we have four main benchmark areas. Keyword spotting, visual wake words, anomaly detection, and image classification. These generally map to some of the use cases above. We will also note that MLPerf often starts lines with one set of benchmarks and evolves them over time.
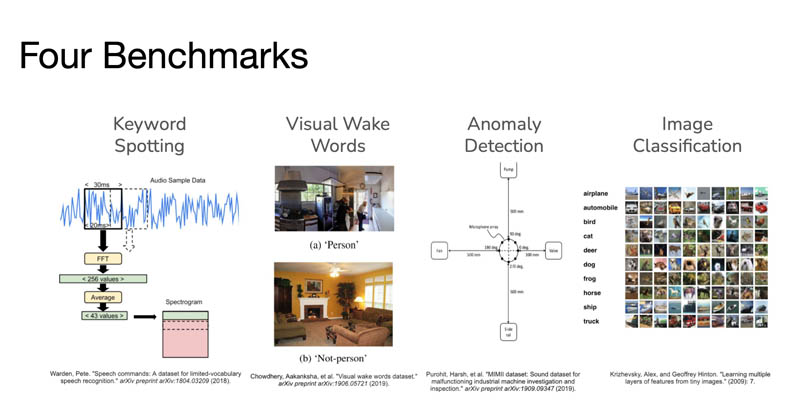
MLPerf has its normal open/ closed and other categories for submissions, but the metrics are latency, accuracy, and energy.

In this space, the energy is a big deal since a few mW can be an enormous change so there is a specific test setup to be able to measure power.
Final Words
In terms of the number of devices, and the number of companies and people impacted, MLPerf Tiny is the most important MLPerf benchmark in the suite. When one looks at the number of training GPUs or servers at the edge, there are certainly many, but we do not see even large organizations installing billions of 600W+ GPUs/ AI accelerators in the next few years. There is room in the market for billions of AI-enabled sensors doing AI inferencing. In a few years, this will be extremely common which makes this space perhaps the most exciting area in AI.