The Huawei Davinci core is designed to take NVIDIA head-on in AI. What is more, the company is expecting to sell millions of Davinci core devices over the next year. While many competitors in the AI space are small and underfunded, without a clear path to market, Huawei has the resources and market to sell their AI chips which makes them very interesting. At Hot Chips 31 (2019), the company showed off its newest Ascend 910 AI training chip.
Huawei DaVinci Core
At the heart of Huawei’s efforts is the DaVinci core. “DaVinci” and “Davinci” were used throughout the Hot Chips 31 presentation, so we are going to use “DaVinci”.
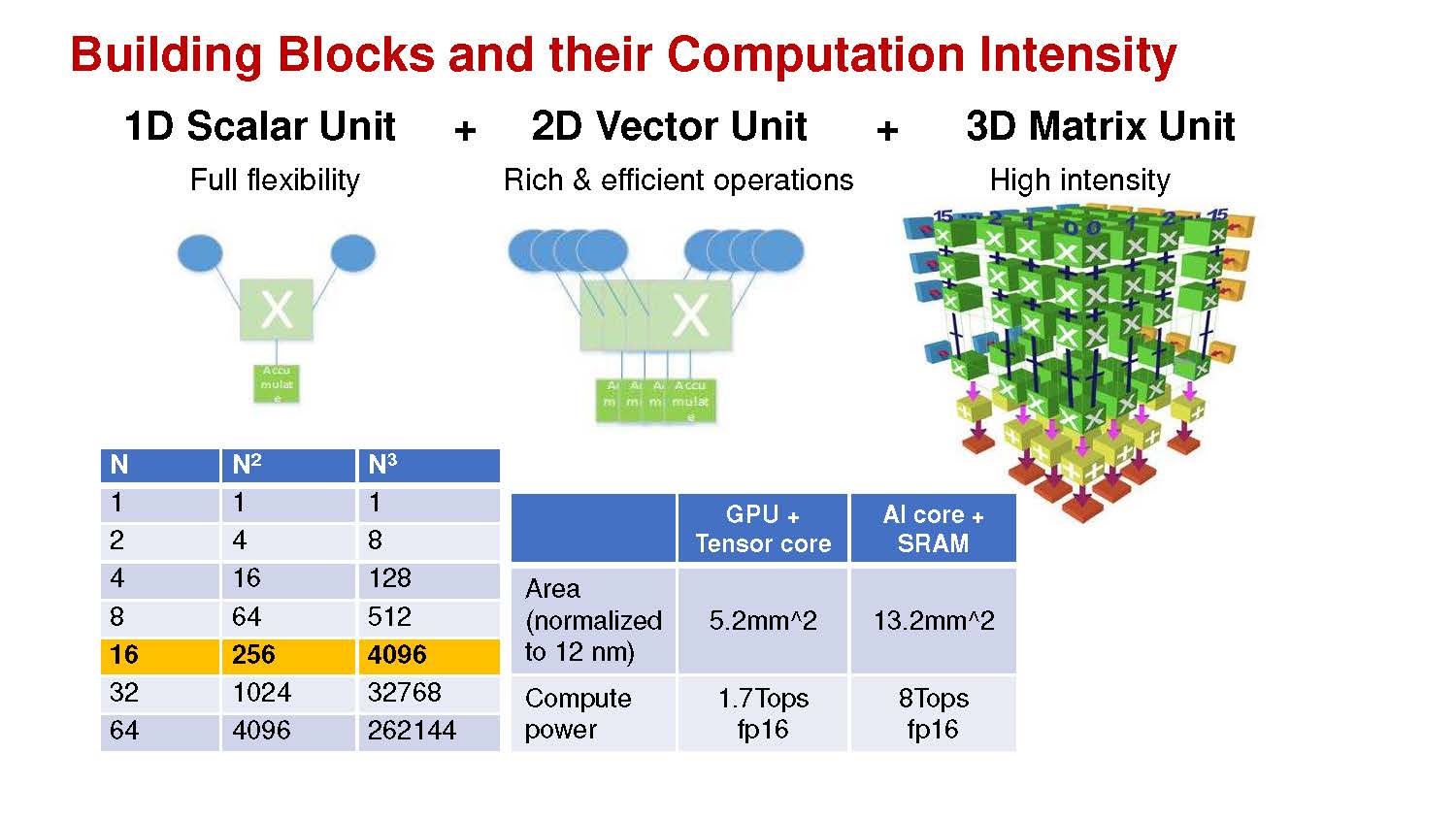
Huawei, like many of the companies coming up with specific AI chips, says that they are more efficient both in terms of compute and in terms of memory bandwidth than NVIDIA’s offerings.
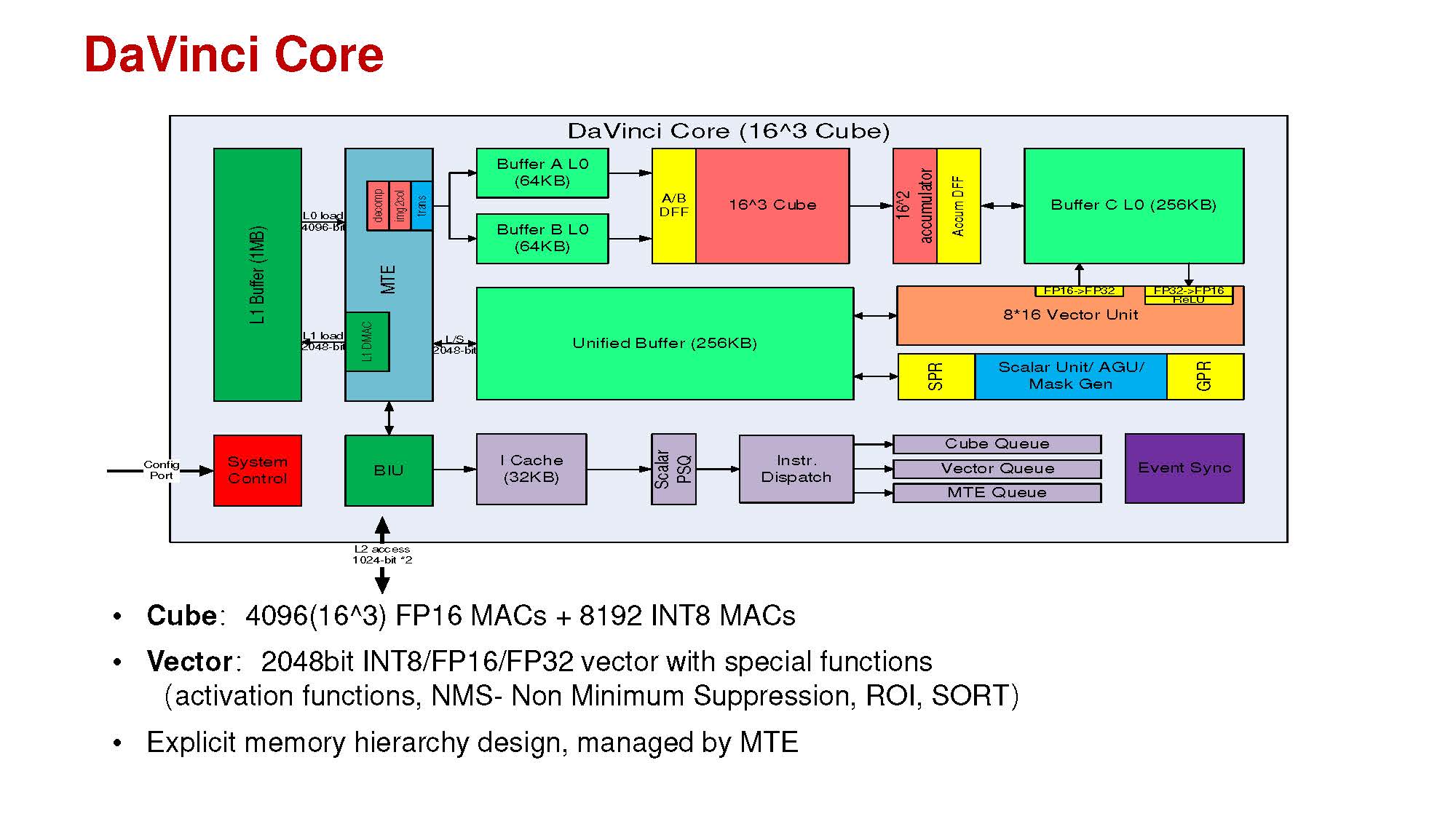
Another key to Huawei’s design is that this DaVinci core needs to scale from single units to larger units. Huawei wants to make this a building block that it can scale from IoT and automotive to large training chips.
Huawei Ascend 910 AI Training Chip
With 32 DaVinci AI cores, the Huawei Ascend 910 is the company’s flagship AI training chip. The chip combines these DaVinci AI cores with high-speed HBM2 memory, similar in some ways to how the NVIDIA Tesla solutions work. The main chip also has a number of additional logic blocks such as 128 channel video decoding engines beyond the DaVinci cores and memory controllers, but there is a Nimbus V3 chip that handles most of the I/O. This Nimbus V3 is co-packaged alongside the main chip and the HBM2 stacks.
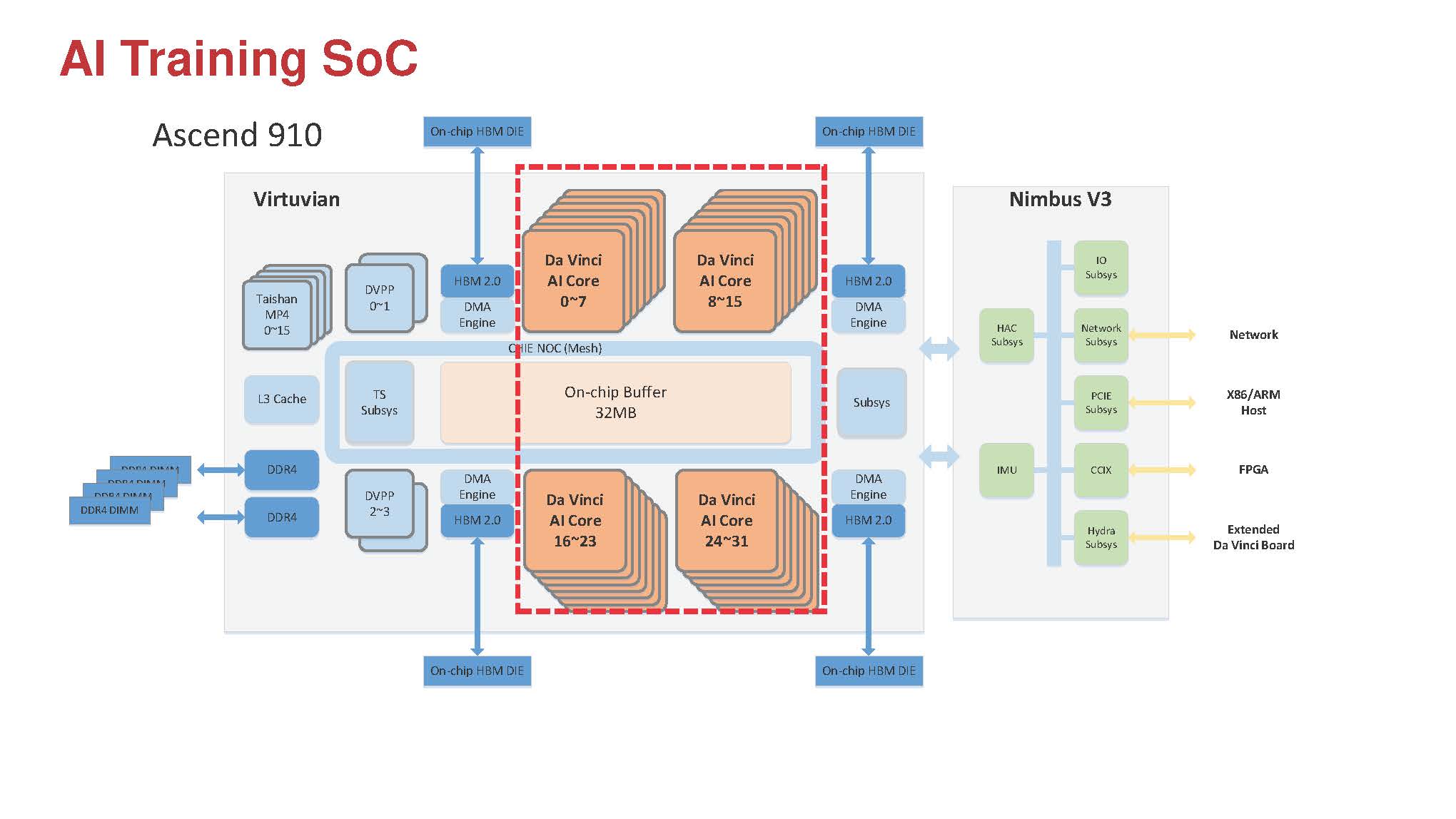
Compared to the Ascend 310, the Huawei Ascend 910 is designed to run at a higher power envelope (350W) and higher performance. Huawei claims 256 TFLOPs of FP16 performance. Update: To make this clearer, the Tesla V100 is 112-125 TFLOPs for deep learning thanks to its tensor cores.
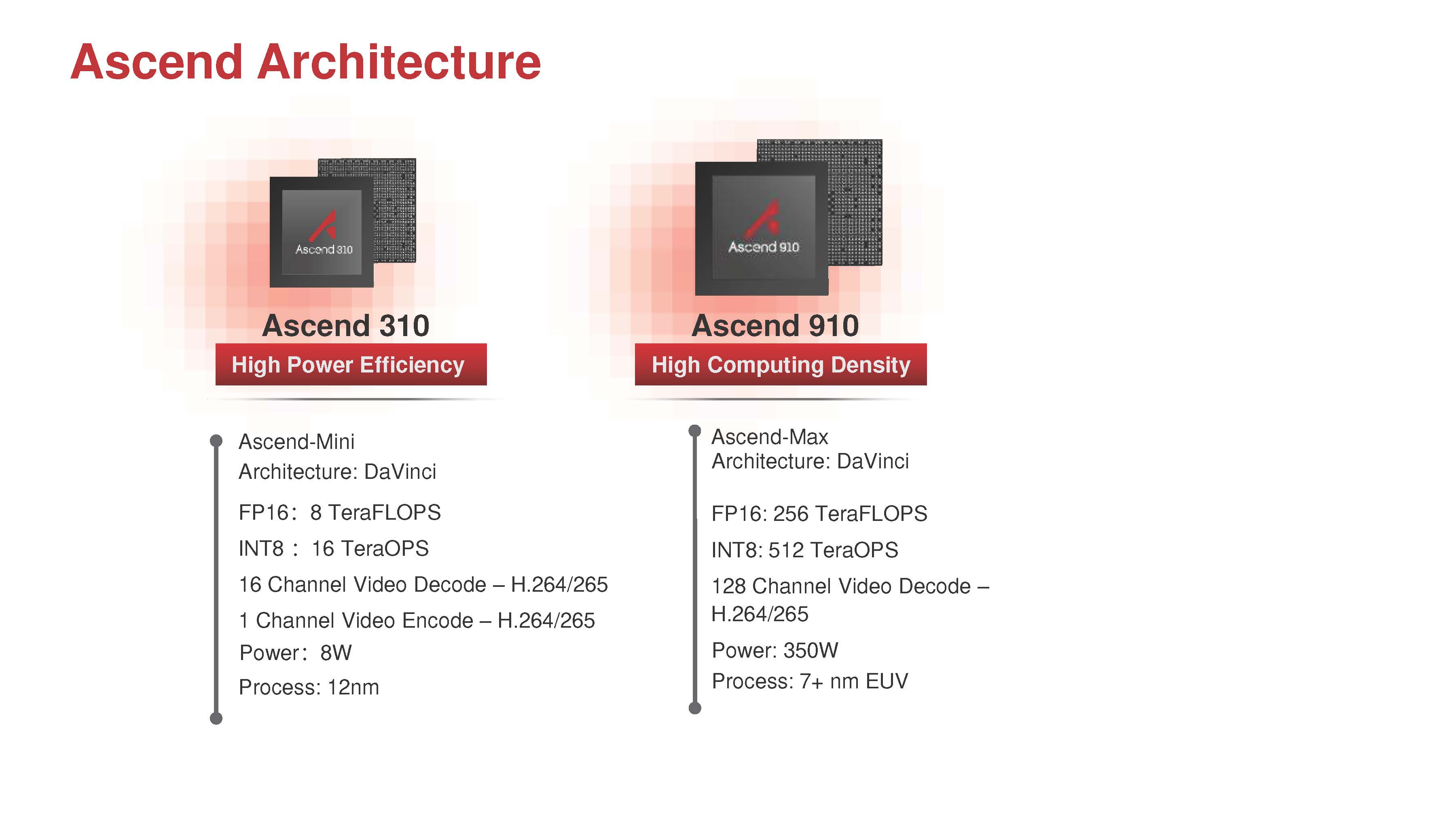
There are four HBM2 memory stacks, the main Virtuvian die with the DaVinci AI cores, and the Nimbus V3 I/O chip. For mechanical stability, there are two 110mm2 dummy dies integrated. Huawei includes the 220mm2 of dummy dies their totals, but we would likely call this a 1028mm2 solution.
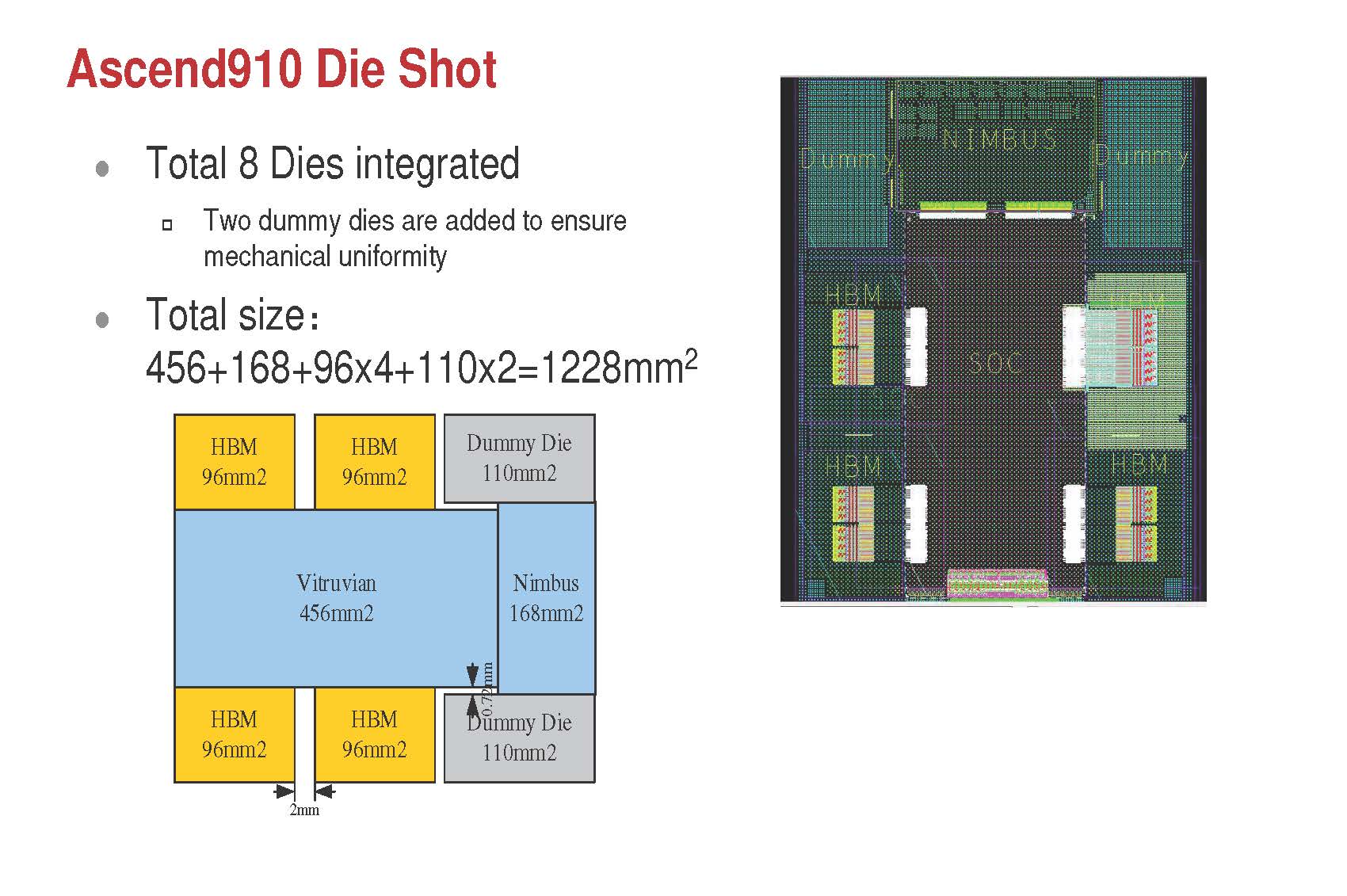
The Ascend 910 floorplan involves an array of 32 DaVinci cores along with 720Gbps of inter-chip links plus 2x 100Gbps RoCE networking interfaces.
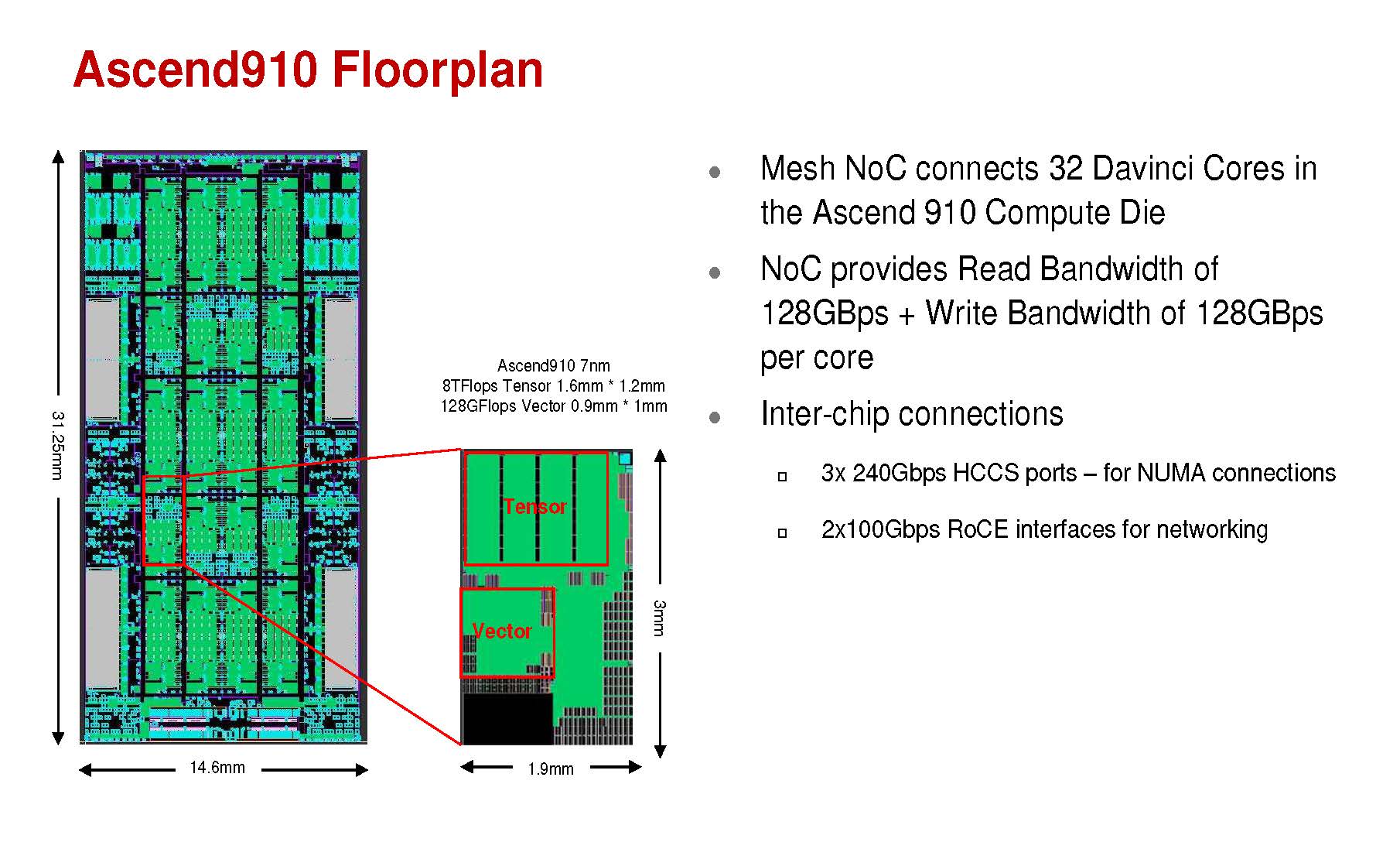
The on-chip interconnect is considered a mesh with six rows and four columns. Huawei claims TB/s figures for the L2 cache access and HBM2 access.
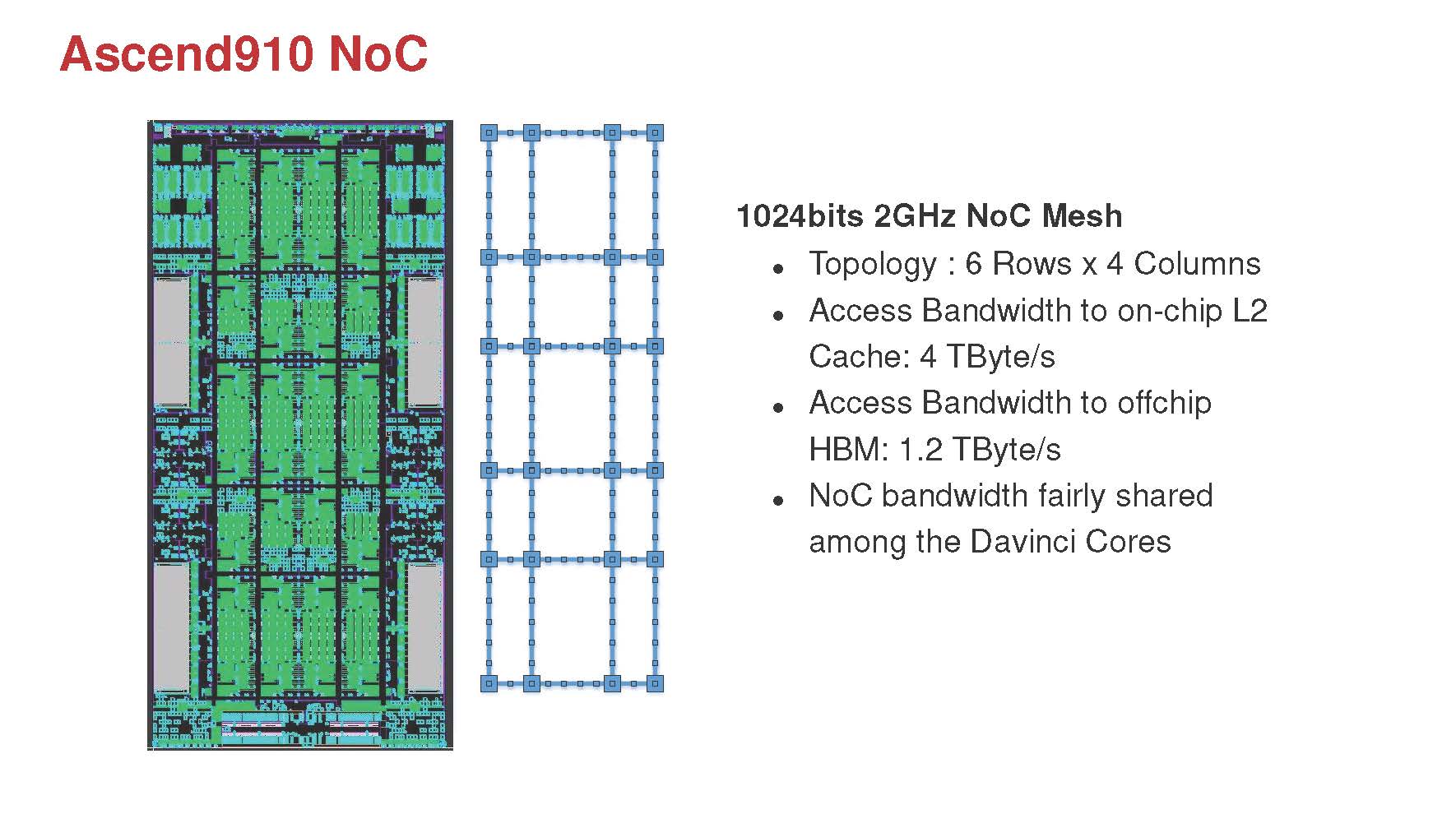
Overall, this is a very interesting solution. Huawei is focusing on the same type of form factors and spectrum that NVIDIA currently offers. Putting that together, the company has solutions to scale-up these chips into systems and clusters much as NVIDIA has done.
Huawei Ascend 910 AI Training Solutions
Beyond the chip, Huawei also has these designed into systems. Here, the topology will remind you a lot of the NVIDIA DGX-1/ HGX-1 as we saw in DeepLearning12. Here, the company has eight Ascend 910 modules installed plus a dual Intel Xeon Scalable platform. We were slightly surprised that these servers do not use the Huawei Kunpeng 920 64-Core Arm Server CPU with CCIX and PCIe Gen4.
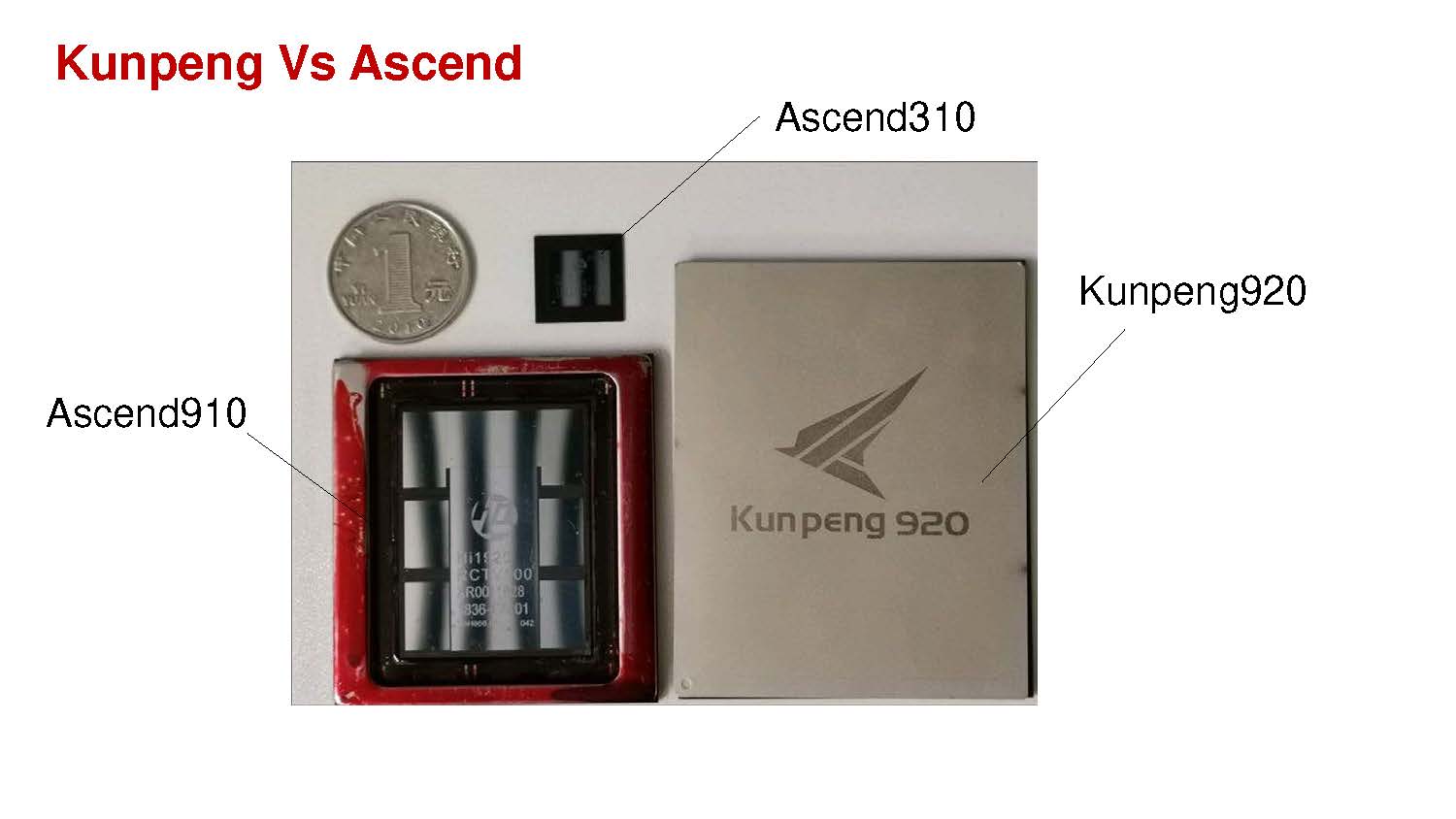
The Ascend 910 also supports CCIX so that would have made for an interesting pairing. Also of note, the Ascend 310 chip uses Arm A55 cores.
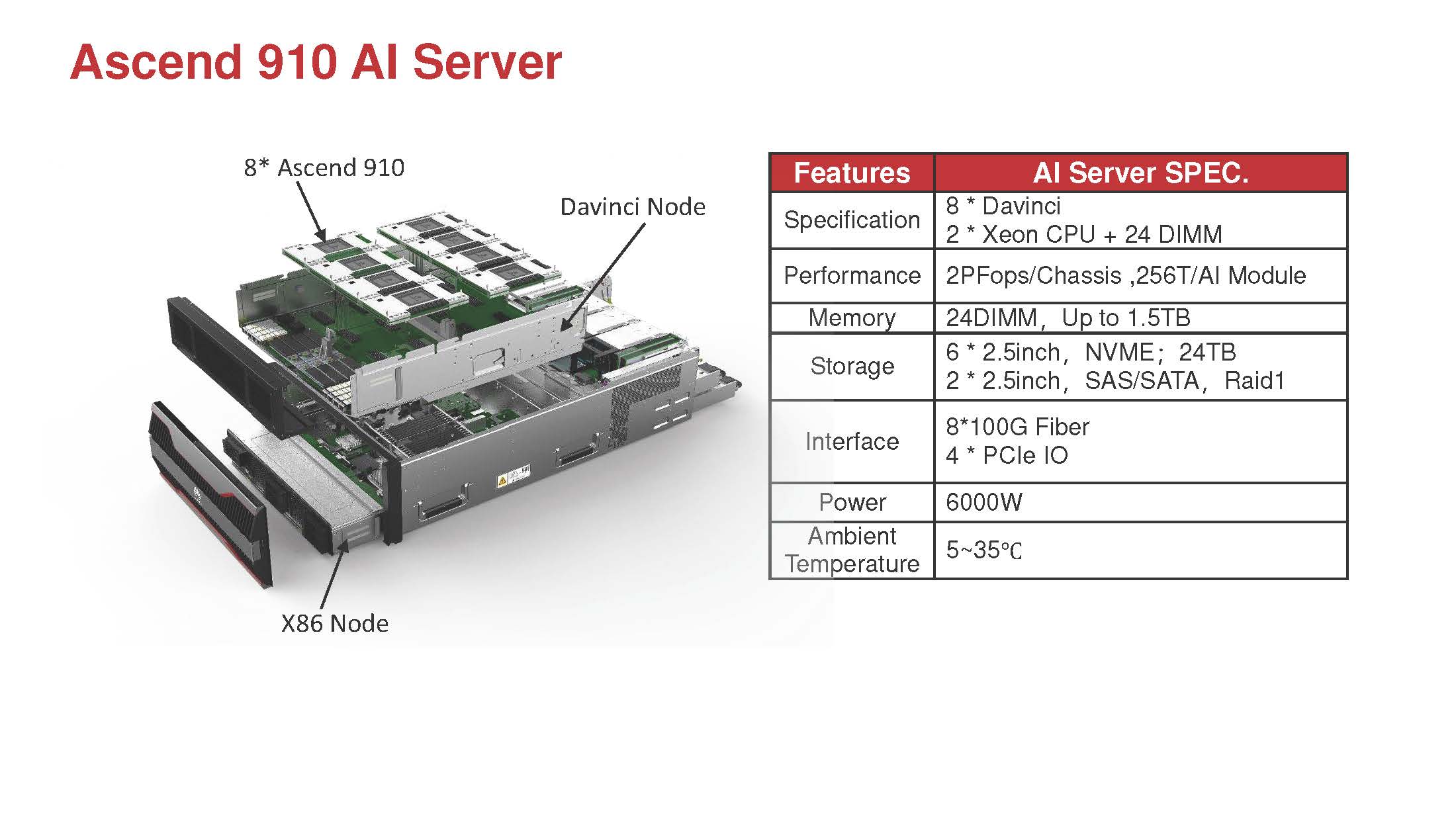
Of course, Huawei has this also designed as a larger cluster.
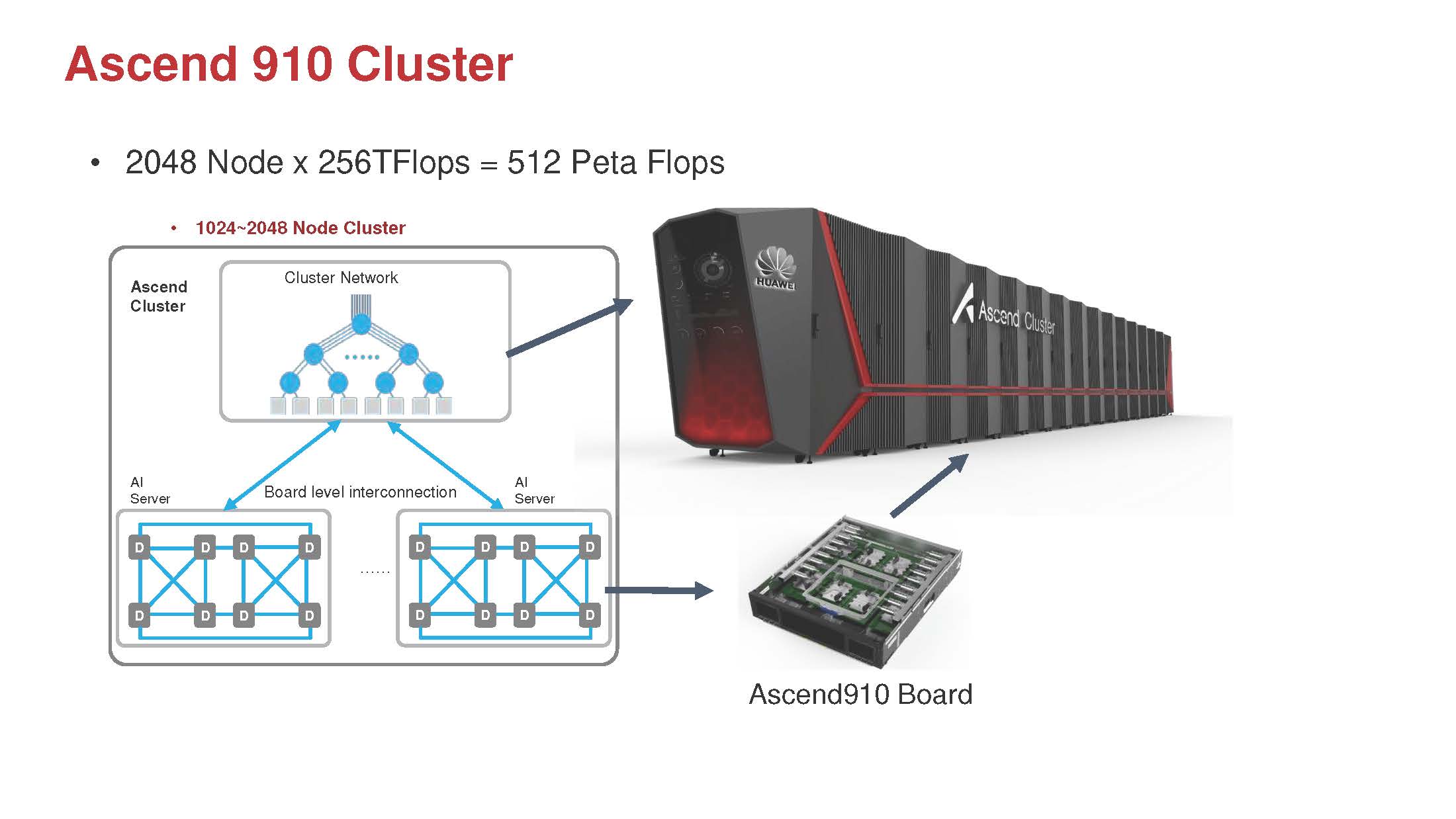
With eight Ascend 910 chips on each board, one can see that the cluster is designed with water-cooling. If you look at the NVIDIA DGX Superpod, it is clear that Huawei is forming a competitive solution.
Final Words
The DaVinci cores are not just designed for training. Huawei also has the Ascend 310 for AI inferencing at the edge. There is also a mid-range Ascend 610 mentioned that seems to be a higher bandwidth per DaVinci core, but lower DaVinci core count unit versus the Ascend 910.
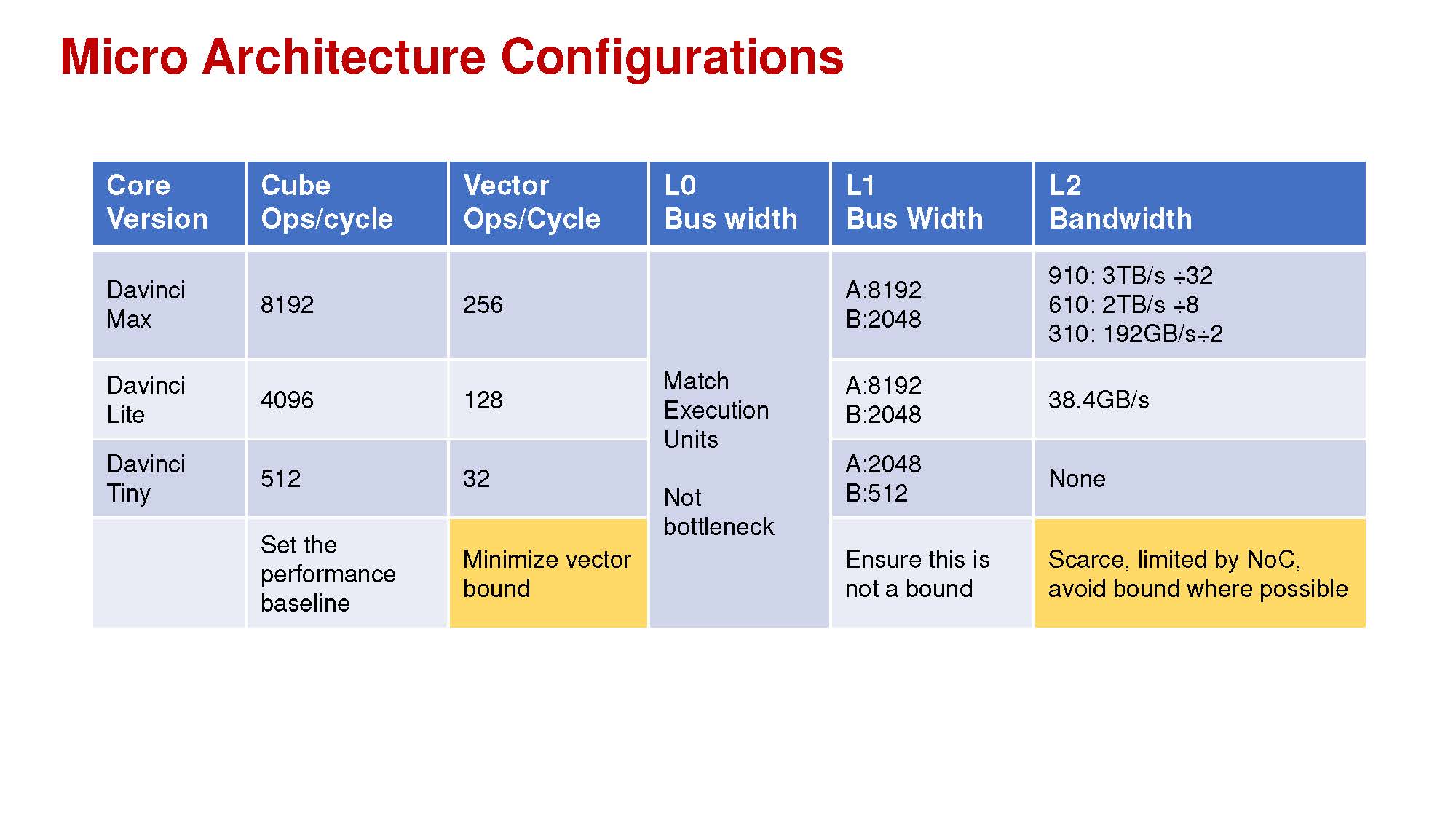
What is clear is that Huawei is going to be pushing away from its dependence on NVIDIA Tesla V100 cards with these releases. It has chips designed for training through edge inferencing and automotive. One of NVIDIA’s strong points is CUDA’s ability to scale from the Jetson Nano to the DGX-2H. Huawei is building a similar scale ecosystem and has the ability to make it the solution of choice for its domestic market.
@Patrick Do you ever see Huawei offering their chips as standalone modules on the OCM form factor or do you see this mostly as an in-house solution?
Comment: Just went back over the slides of Ian Cutress to look for the answer to above question and on their own slides they quoted the V100 at 120 TFlops FP16. So I guess their FP16 output is around 2 times that of the V100, assuming their claims are accurate.
L.P. it is in the article FP16 is around 30TFLOPs. The actual range is ~28-31 so 30 sounds right. If someone is telling you a V100 is 120TFLOPS at FP16 they’re wrong.
But they have tensor ops which are different and much higher.
@GregUT I work with them every day so know V100s quite well. It is more of an isolated quote of their claims, where the actual 30TFlops seem to be incomparable to the quoted 256TFlops number.
Here is the respective slide:
https://images.anandtech.com/doci/14756/IMG_20190819_171052.jpg
Guys – added an update. I was in the midst of doing VMworld coverage and missed that one.
@L.P. and @GregUT: if you look at source image, you see that claim is not professional – you can‘t compare NVIDIA Tensor Core, TPU and Ascend like they do. Just not similar things compare. Bettet check MLPerf https://mlperf.org/ – more professional benchmark on real cases.
Great article. Thank you Patrick for the coverage. This was more than 2 years ago. Have there been any updates since?