Recently we published our NVIDIA Titan RTX review. As that was in the pipeline, we saw NVIDIA double down on RTX server offerings at GTC 2019. Our editor-in-chief, Patrick, at GTC 2019 decided to buy a second Titan RTX. He thought, as did Cliff, that the NVIDIA Titan RTX would often be deployed in pairs using NVLink. For those that need desktop compute power for deep learning or other professional applications, two Titan RTX cards can offer a lot of value for getting work done quickly. In this review, we are going to look at the compute performance of two NVIDIA Titan RTX cards connected via NVLink.
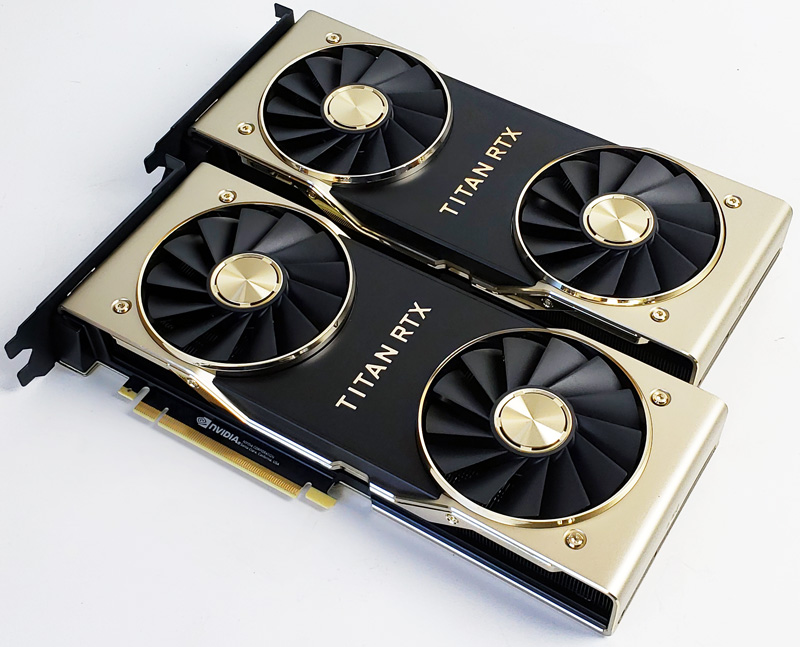
First, let us get our test system setup and find out how two NVIDIA Titan RTX’s with NVLink perform.
NVIDIA RTX NVLink Bridge Overview
To test two NVIDIA Titan RTX GPUs, we need two cards and an NVIDIA RTX NVLink Bridge.
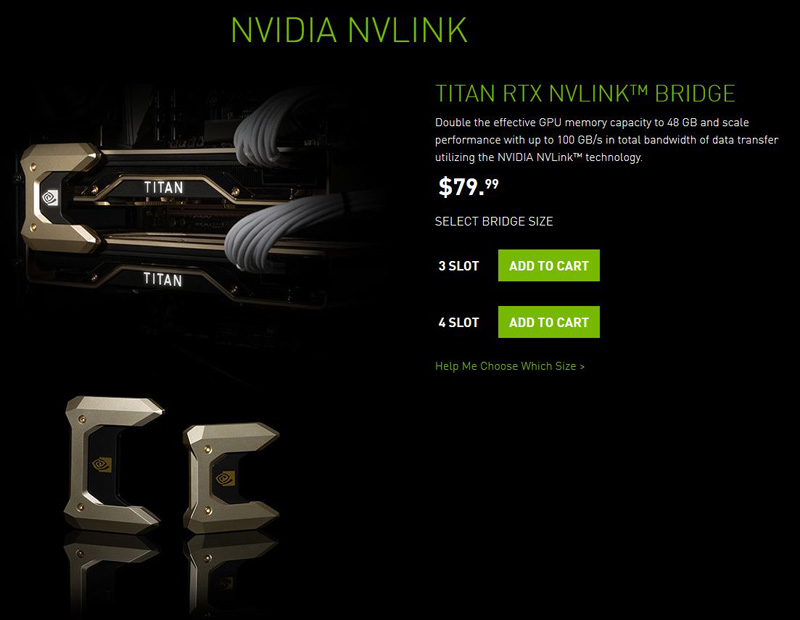
For our needs, we ordered a Titan RTX NVLink Bridge from NVIDIA’s site. These come in two sizes, a 3-Slot or 4-Slot Bridge. The motherboard we use for our GPU benchmarking purposes is an ASUS WS C621E SAGE which has plenty of space for the 4-Slot Bridge. We could have used the 3-Slot Bridge but wanted to give the extra slot space to allow for better cooling of the two NVIDIA Titan RTX GPUs. They are hot cards.
This is a pricey bridge costs $79.99 before tax. Four days later we had our Titan RTX NVLink Bridge in hand. NVIDIA presents us with a very nice box for our bridge; its gold color matches our two NVIDIA Titan RTX keeping these high-end GPUs all on the same color scheme.
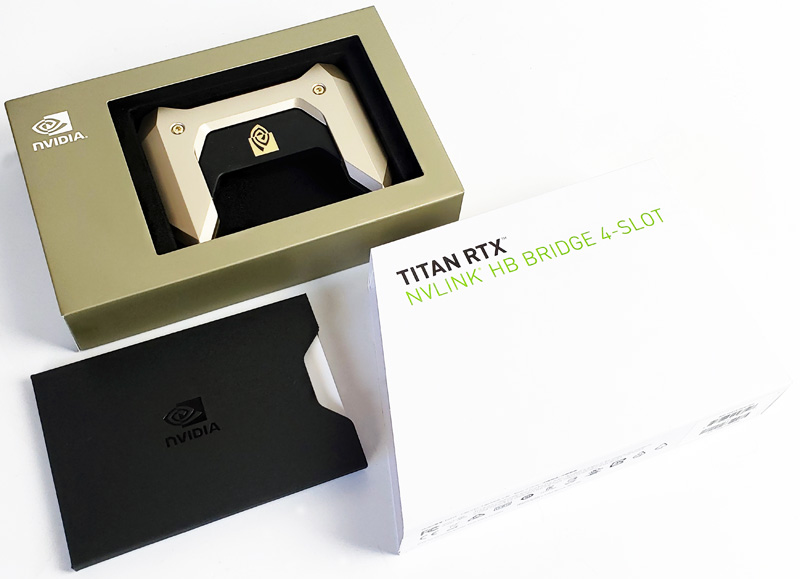
Sliding off the box cover we see the package contents. Over the bridge, is a black sleeve which contains the support guide. Not much of interest is in the guide, but we did find that the NVLink Bridge comes with a three-year warranty which we will most likely void by promptly taking it apart.
Taking the bridge out of the box we get a look at the NVIDIA Titan RTX NVLink Bridge.
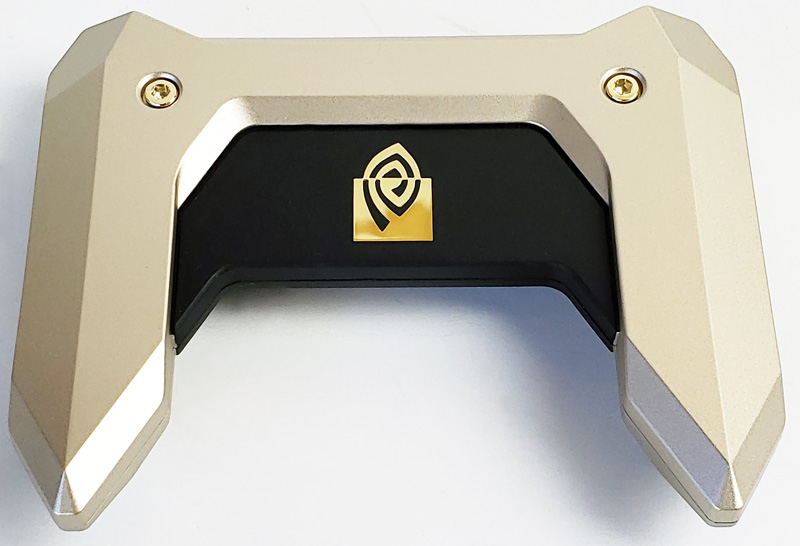
We have to admit that the bridge is very classy looking and has a quality feel to it. In addition to looking good, the NVIDIA logo is backed by LEDs that light up when in use.
Let’s flip the bridge over and take a look at the connectors.
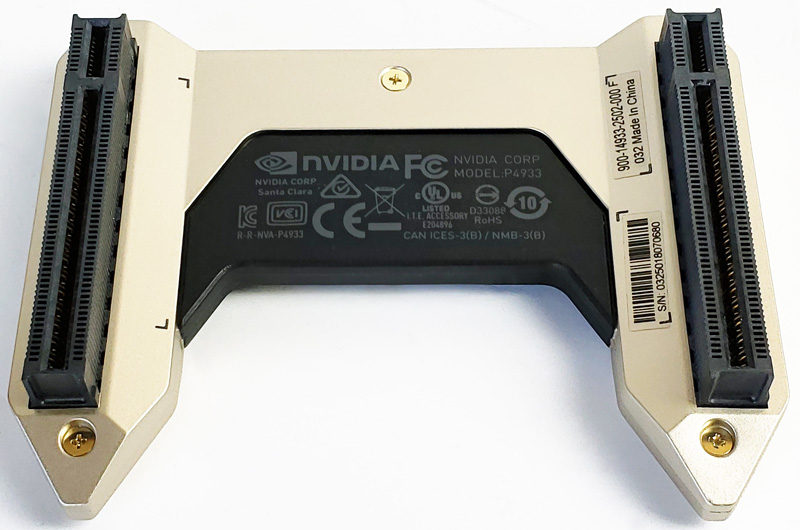
The connectors appearance remind us of a PCIe x8 slot.
Removing the five screws that hold the bridge together, we can flip the cover over and see the PCB board underneath.
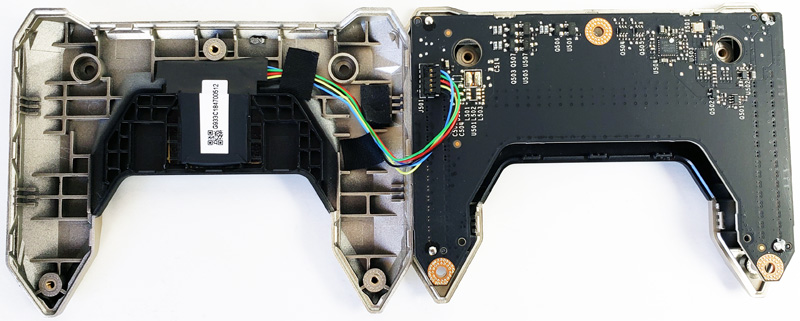
As we have said, the NVIDIA Logo on the top has a LED underneath that lights up when in use. Here we can see the connector and wires that connect the LED to the motherboard. Other manufacturers with different bridges use this to control LEDs on their bridges.
Here we see a close up of the PCB board and the small number of surface mount chips present.
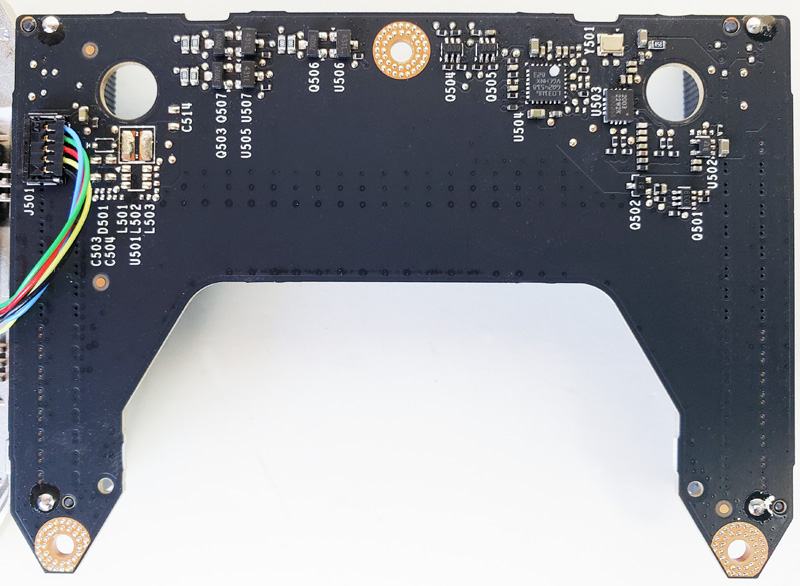
Next, let us take a look at the dual NVIDIA Titan RTX NVLink setup and continue on with our performance testing.
Incredible ! The tandem operates at 10x the performance of the best K5200 ! This is a must have for every computer laboratory that wishes to be up to date allowing team members or students to render in minutes what would take hours or days ! I hear Dr Cray sayin ” Yes more speed! “
This test would make more sense if the benchmarks were also run with 2 Titan RTX but WITHOUT NVlink connected. Then you’d understand better whether your app is actually getting any benefit from it. NVLink can degrade performance in applications that are not tuned to take advantage of it. (meaning 2 GPUs will be better than 2+NVLink in some situations)
I’m kind of missing the: NAMD Performance.
STH is freaking awesome. Great review William. You guys have got a great dataset building here
Great review yes – thanks !
2x 2080 Ti would be nice for a comparison. Benchmarks not constrained by memory size would show similar performance to 2x Titan at half the cost.
It would also be interesting to see CPU usage for some of the benchmarks. I have seen GPUs being held back by single threaded Python performance for some ML workloads on occasion. Have you checked for CPU bottlenecks during testing? This is a potential explanation for some benchmarks not scaling as expected.
Literally any amd GPU loose even compared to the slowest RTX card in 90% of test…In int32 int64 they don’t even deserve to be on chart
@Lucusta
Yep the Radeon VII really shines in this test. The $700 Radeon VII iis only 10% faster than the $4,000 Quadro RTX 6000 in programs like davinci resolve. It’s a horrible card.
@Misha
A Useless comparison, a pro card vs a not pro in a generic gpgpu program (no viewport so why don’t you say rtx 2080?)… The new Vega VII is compable to rtx quadro 4000 1000$ single slot! (pudget review)…In compute Vega 2 win, in viewport / specviewperf it looses…
@Lucusta
MI50 ~ Radeon VII and there is also a MI60.
Radeon VII(15 fps) beats the Quadro RTX 8000(10 fps) with 8k in Resolve by 50% when doing NR(quadro RTX4000 does 8 fps).
Most if not all benchmarking programs for CPU and GPU are more or less useless, test real programs.
That’s how Puget does it and Tomshardware is also pretty good in testing with real programs.
Benchmark programs are for gamers or just being the highest on the internet in some kind of benchmark.
You critique that many benchmarks did not show the power of nvlink and using pooled memory by using the two cards in tandem. But why did you not choose those benchmarks and even more important, why did you not set up your tensorflow and pytorch test bench to actually showcase the difference between nvlink and one without?
It’s a disappointing review in my opinion because you set our a premise and did not even test the premise hence the test was quite useless.
Here my suggeation: set up a deep learning training and inference test bench that displays actual gpu memory usage, the difference in performance when using nvlink bridges and without, performance when two cards are used in parallel (equally distributed workloads) vs allocating a specific workload within the same model to one gpu and another workload in the same model to the other gpu by utilizing pooled memory.
This is a very lazy review in that you just ran a few canned benchmark suites over different gpu, hence the rest results are equally boring. It’s a fine review for rendering folks but it’s a very disappointing review for deep learning people.
I think you can do better than that. Pytorch and tensorflow have some very simple ways to allocate workloads to specific gpus. It’s not that hard and does not require a PhD.
Hey William, I’m trying to set up the same system but my second GPU doesn’t show up when its using the 4-slot bridge. Did you configure the bios to allow for the multiple gpus in a manner that’s not ‘recommended’ by the manual.
I’m planning a new workstation build and was hoping someone could confirm that two RTX cards (e.g. 2 Titan RTX) connected via NVLink can pool memory on a Windows 10 machine running PyTorch code? That is to say, that with two Titan RTX cards I could train a model that required >24GB (but <48GB, obviously), as opposed to loading the same model onto multiple cards and training in parallel? I seem to find a lot of conflicting information out there. Some indicate that two RTX cards with NVLink can pool memory, some say that only Quadro cards can, or that only Linux systems can, etc.
I am interested in building a similar rig for deep learning research. I appreciate the review. Given that cooling is so important for these setups, can you publish the case and cooling setup as well for this system?
I only looked at the deep learning section – Resnet-50 results are meaningless. It seems like you just duplicated the same task on each GPU, then added the images/sec. No wonder you get exactly 2x speedup going from a single card to two cards… The whole point of NVLink is to split a single task across two GPUs! If you do this correctly you will see that you can never reach double the performance because there’s communication overhead between cards. I recommend reporting 3 numbers (img/s): for a single card, for splitting the load over two cards without NVLink, and for splitting the load with NVLink.