At Hot Chips 30, NVIDIA gave out more details on its largest system for deep learning and AI training. The NVIDIA DGX-2 scales up to 16x NVIDIA Tesla V100 32GB GPUs for up to 512GB. It also uses the NVSwitch fabric to allow high-speed memory access between GPU HBM2 memory. By providing a high-speed interconnect, it can reduce the cost of “remote” (in the same chassis) memory lookup. Here are some of the details that NVIDIA provided.
NVIDIA DGX-2 with NVLink
Here are the key speeds and feeds of the NVIDIA DGX-2. We covered many of these in the NVIDIA DGX-2 Pushing Limits at 16 GPUs and 512GB of HBM2 RAM launch piece.
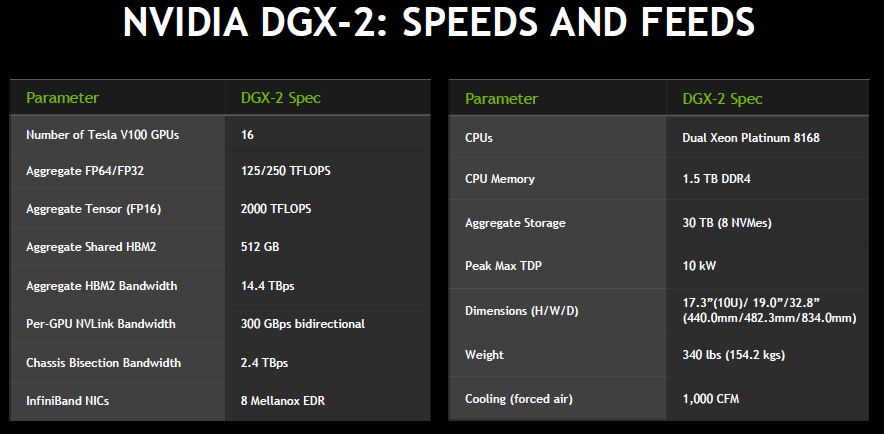
Each NVIDIA DGX-2 has two GPU boards that utilize these NVSwitches to create a fabric that allows for fast GPU-to-GPU communication.
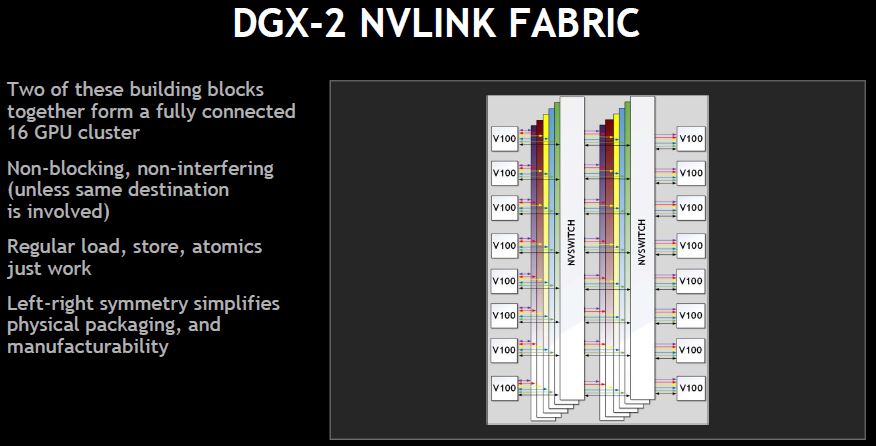
The NVIDIA DGX-2 also needs to connect the GPUs back to the CPUs and other PCIe devices such as storage and NICs. In the DGX-, each NIC has a clear path to a pair of GPUs. This allows high bandwidth GPUDirect RDMA.
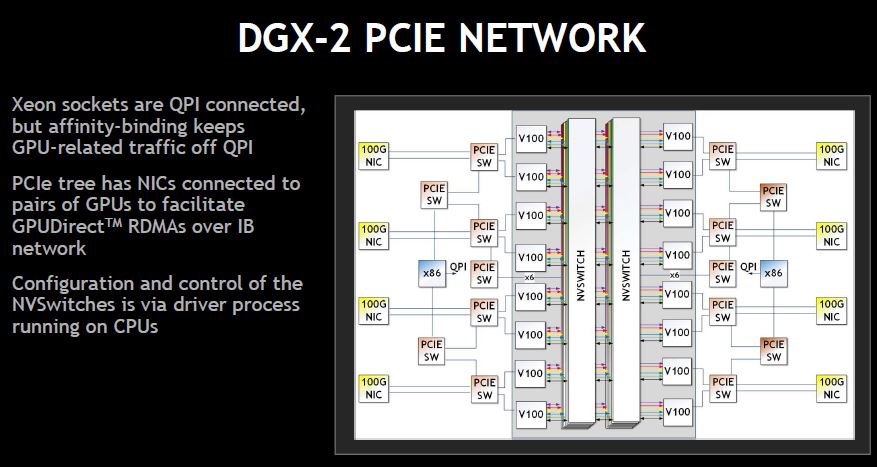
GPU baseboard. The DGX-2 has two GPU baseboards connected via passive PCBs. There are no buffers on the PCBs to conserve space and power.
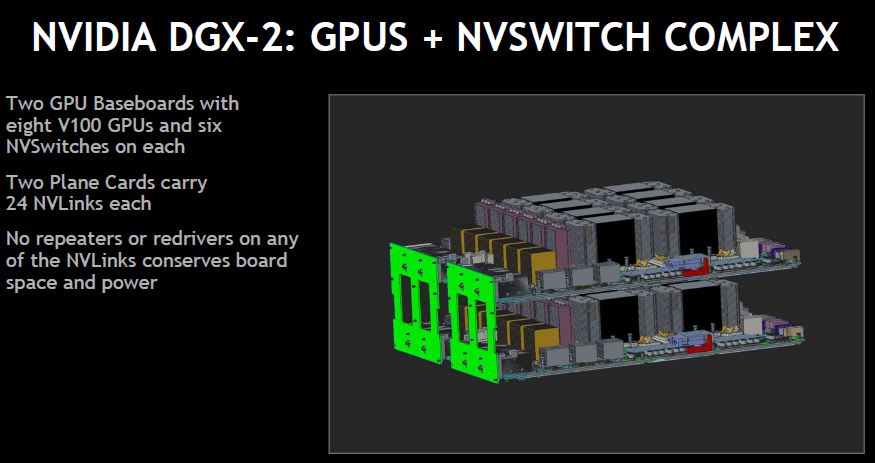
PCIe is piped in via the midplane. Switches from 12V power distribution to 48V power.
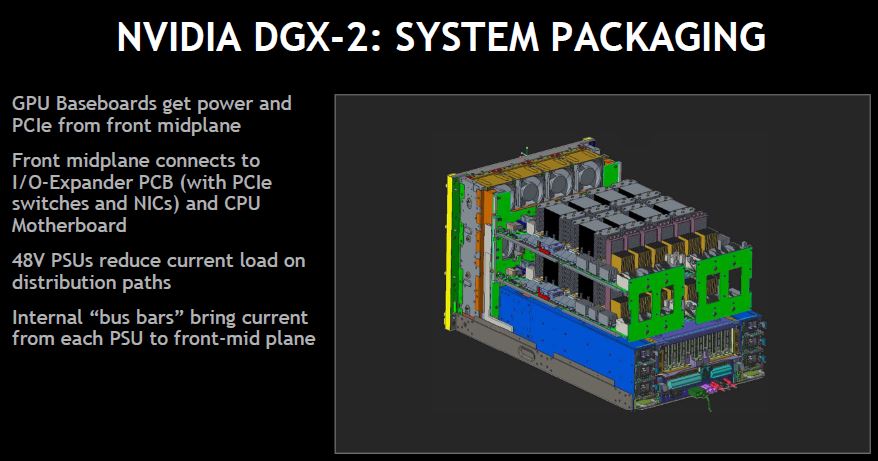
A 10kW air cooled system requires heavy-duty fans. Cooling wise there are four 92mm fans cooling each GPU baseboard. 60mm front fans and extra internal fans cooling the system.
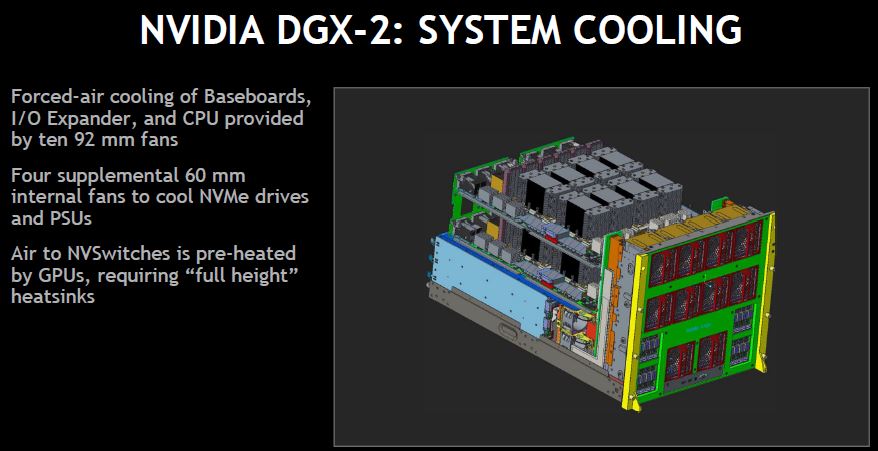
The NVIDIA DGX-2 utilizes custom connectors and traces. Instead of being standard PCIe cards, the system uses a differnet package than the DGX-1 and groups Rx and Tx I/O.
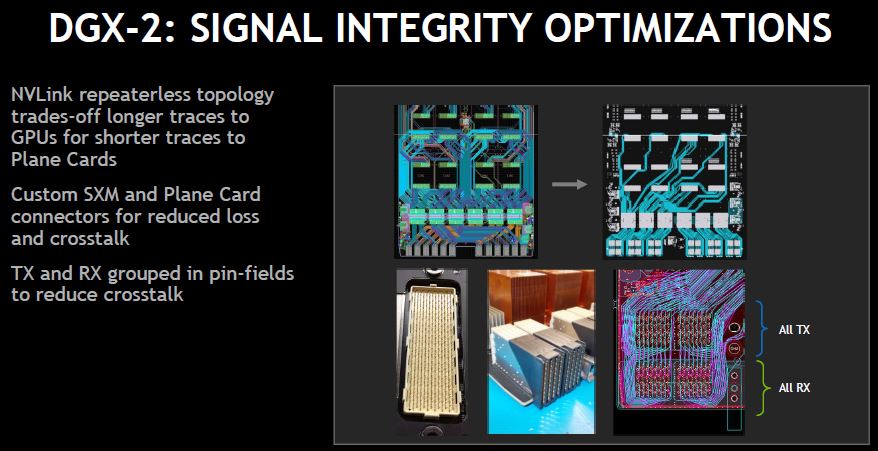
Overall, there is a lot of systems engineering that went into the DGX-2. This is for good reason. The DGX-2 has performance benefits over two DGX-1’s because it is a larger scale-up solution.
NVIDIA DGX-2 versus DGX-1
NVIDIA also shared some cherry-picked numbers on performance. The company admitted that these were best cases to show off the benefits of the DGX-2 over the DGX-1.
The first example is bisectional bandwidth for GPU-to-GPU communication. You may notice a small kink at 8 to 9 GPUs. This is where requests start eating into the responses, but the kink is relatively muted.
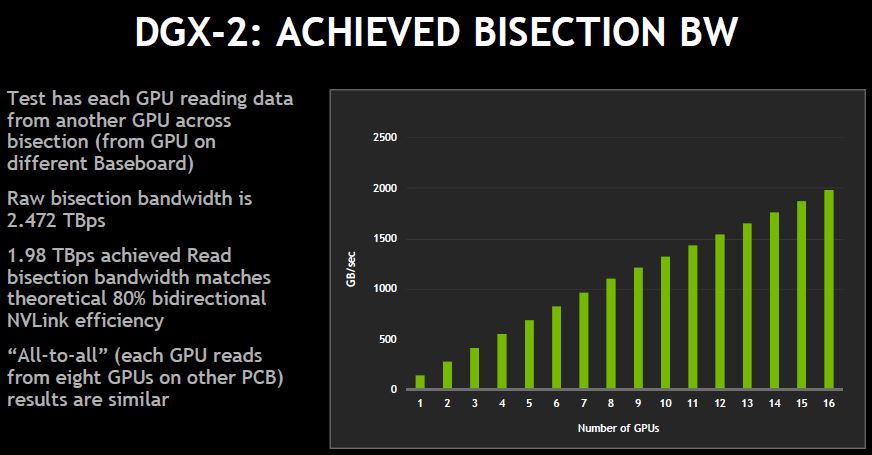
NVIDIA’s CUFFT showed half of a DGX-2 to DGX-1. This is a bit of a best case since the messaging between GPUs costs are high compared to the GPU compute required to solve the problems.
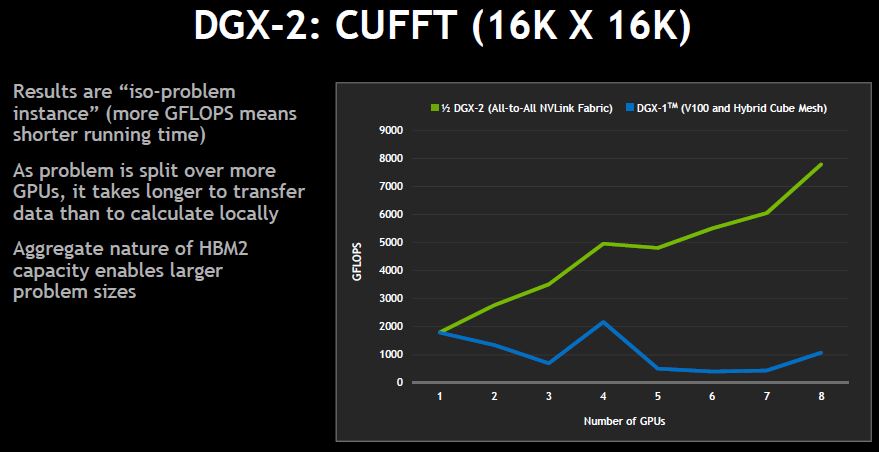
NVIDIA compared two DGX-1 servers versus a single DGX-2 in all-reduce. The performance at larger Infiniband message sizes was 3x while smaller messaging sizes offered an 8x advantage. This is another cherry-picked example that pushes a lot of data over the Infiniband fabric.
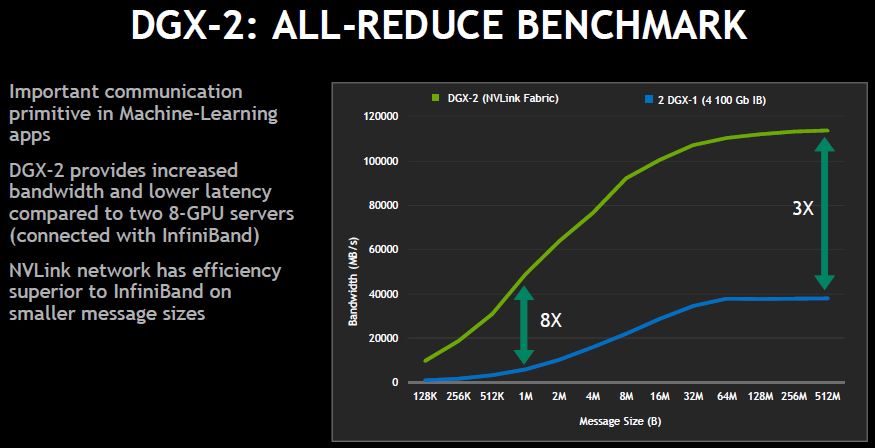
Other highlighted benchmarks give you a 2-2.7x speed-up moving from two DGX-1 class systems to a DGX-2 system.
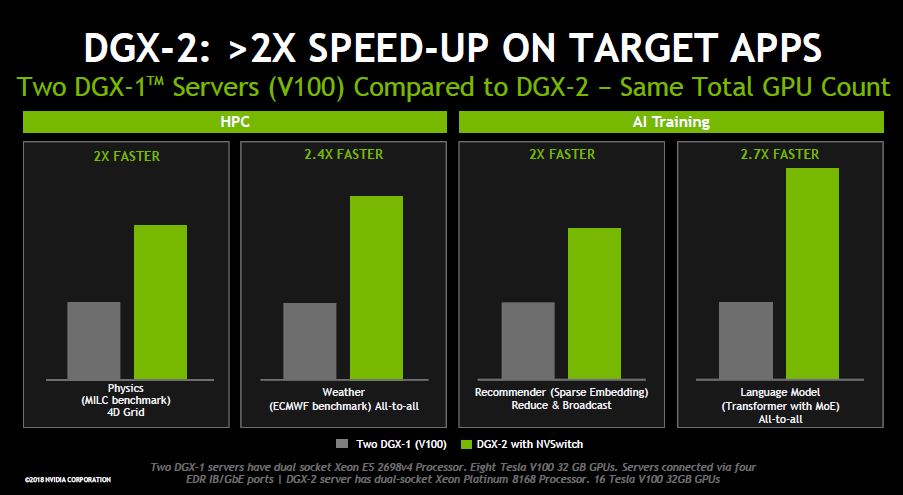
Final Words
There are always problems where scale-up servers out-perform scale-out. Many machine learning/ AI systems problems are constrained by memory bandwidth and capacity. Being able to scale up the problem sizes means that researchers can work on bigger datasets without having to go back to main memory or over a network which are both slow access patterns.
Very interesting package. When this dense integration is upgraded to Turing (1/2 FP), it should set a unit volume, compute record. I would like to get one to perform sub meter resolution planetary geophysics. I wonder if the machine is so noisy when running a large work load that it needs to be acoustically isolated.