This week, NVIDIA gave a preview of their AI at scale system. Specifically, the company went into how they construct the NVIDIA DGX SuperPOD from each node, interconnect it with fabric, and even deploy and manage the solution. The end result culminated in the new Selene supercomputer based on the company’s A100 GPUs and DGX A100 plus Mellanox acquisition derived building blocks.
Building the NVIDIA Selene Supercomputer
NVIDIA discussed some of the big innovations that helped deploy Selene, based on its DGX SuperPOD design during the pandemic. This was a big push since there was a demand for the HPC and AI functionality for COVID research. A key enabler of the DGX SuperPOD deployment speed was that the on-site build was greatly aided by the work that came before components arrived in the data center.

The company said they were deploying the machine in shifts with two technicians working for four hours each in the data center. The company made small changes to make this work such as making the packaging server lift friendly. That made it easy to move the large machines out of the packaging and into racks and helped deploy nodes faster. They deployed 60 systems in a day that NVIDIA says is its loading dock capacity.
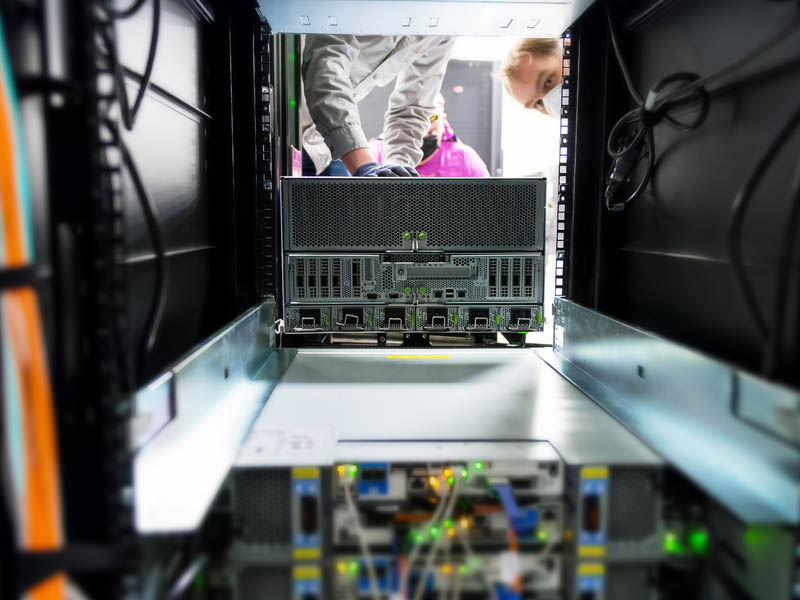
With the DGX SuperPOD, the company is utilizing NVIDIA (Mellanox) HDR 200Gbps Infiniband. NVIDIA uses 40-port Infiniband switches in the DGX SuperPOD. NVIDIA supports a number of topologies but seems to rely on a fat-tree topology for many deployments to ease cabling and deployment. The company also optimizes which port on a DGX A100 connects to specific switch ports to optimize the physical connectivity for the most prevalent software and application connectivity needs.
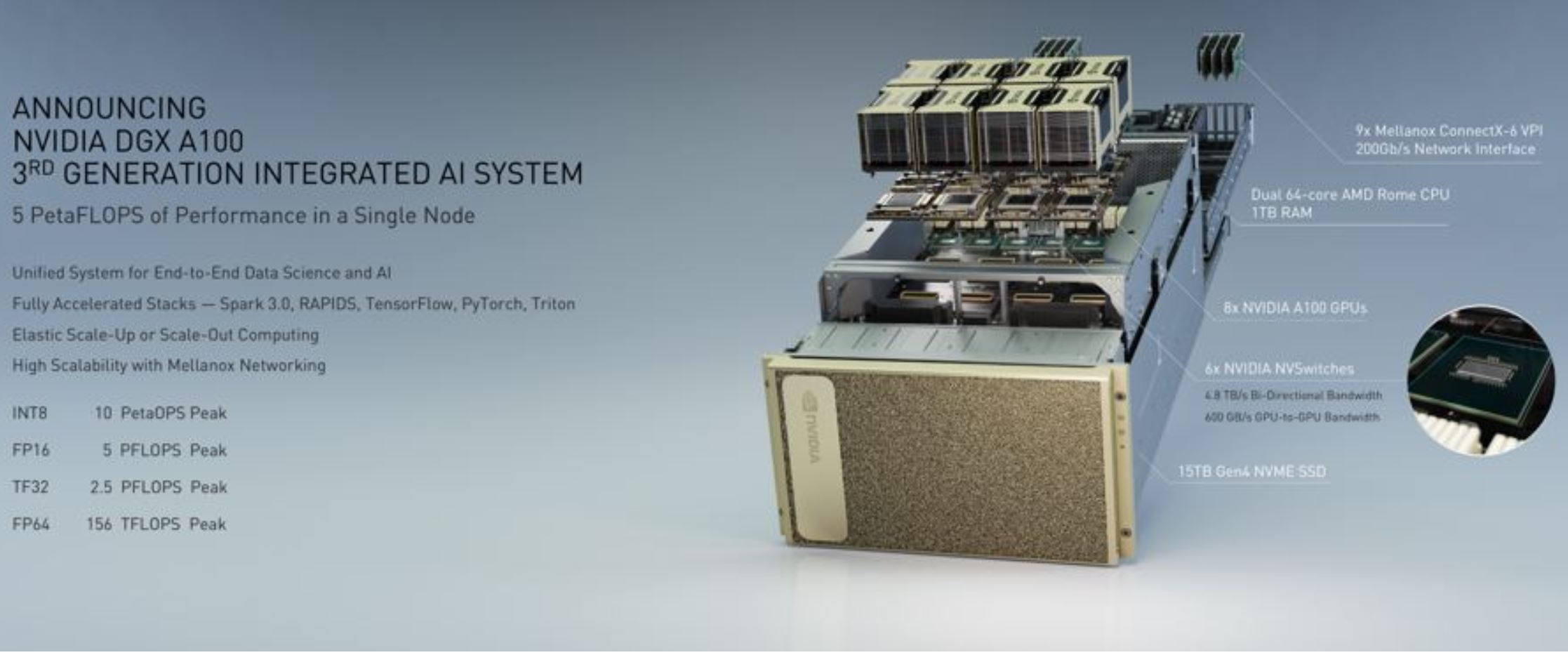
Another good example is that the company calculates and pre-bundles wiring. By using a standard SuperPOD deployment approach, the company is standardizing dimensions for cable runs. It can have cables pre-bundled so they can be brought into a data center easily. Once there, technicians can break the bundle ends out so they can service each machine and connect to the correct switch ports. NVIDIA says it leaves a little give in the cables to allow for different alignment on future generations for upgrade scenarios.

Another key to deploying quickly was how to monitor status LEDs and for loose cables remotely. There were both software tools checking and validating connectivity as well as a little in-data center robot NVIDIA called “Trip”. That nickname came from concerns on-site folks might trip over the robot. Trip is from a company called Double Robotics and is powered by a NVIDIA Jetson TX2.
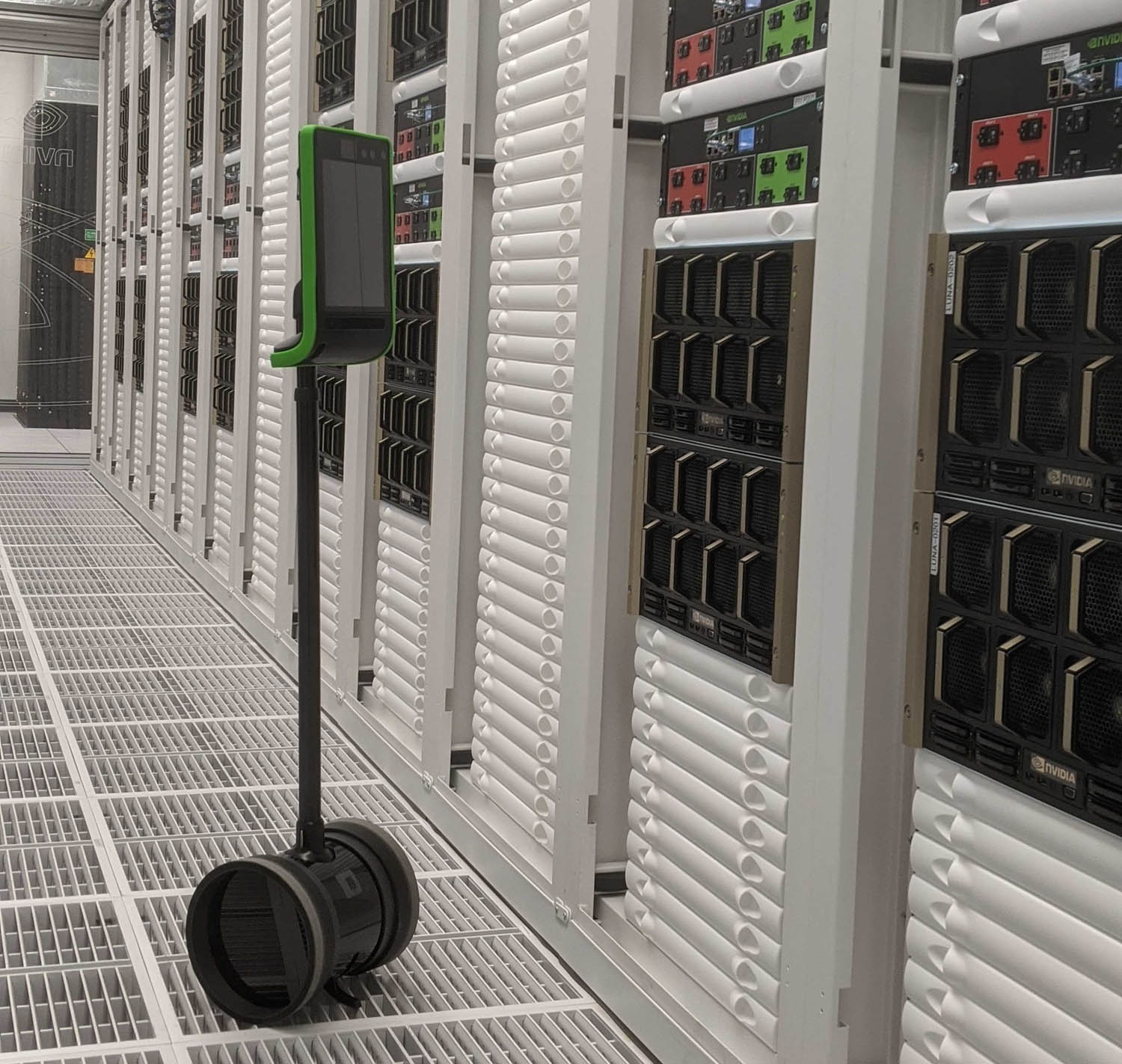
In terms of storage, the company has many tiers of storage. We thought it was interesting that the back-end capacity tier NVIDIA said was a Ceph storage solution. Some other high-performance computing solutions utilize Lustre, Gluster, or storage solutions from 3rd party vendors that NVIDIA partners with.
Final Words
Next week we are going to get deeper into the DGX A100 and Ampere design. For now, we wanted to look at some of the higher-level functions and how the SuperPOD created Selene quickly during the pandemic.
A side impact of this work, like with the DGX machines, is pushing into the territory of some of NVIDIA’s channel partners. Building large systems that are ready to deploy directly from NVIDIA bypasses the partners who have been building systems for years doing the integration work.